Geometric Contrastive Learning for Heterogeneous Graphs Encoding.
2023 IEEE International Conference on Systems, Man, and Cybernetics (SMC)(2023)
Abstract
Heterogeneous graphs can represent many network structures in the real world, and research on heterogeneous graph data has attracted more attention. Most existing approaches require additional label information to obtain meaningful node representations. However, labeling heterogeneous graphs is tedious and time-consuming, and low-quality labels will harm the efficiency of models. In addition, the number of nodes increases exponentially with the distance from the root node, but the linearly expanding Euclidean space is difficult to match this growth rate. In this paper, we propose a unified framework that leverages the label irrelevance of contrastive learning and the unique expressive ability of hyperbolic space to encode heterogeneous graphs. Specifically, we use the contrast mechanism to obtain semantic information in an unsupervised way. Meanwhile, we design hyperbolic encoders that are more suitable for graph structure to learn the latent information from heterogeneous graphs efficiently. Experiments on four real-world heterogeneous graph data sets demonstrate the competitive efficacy of the proposed method.
MoreTranslated text
Key words
contrastive learning,heterogeneous graphs,geometric learning
AI Read Science
Must-Reading Tree
Example
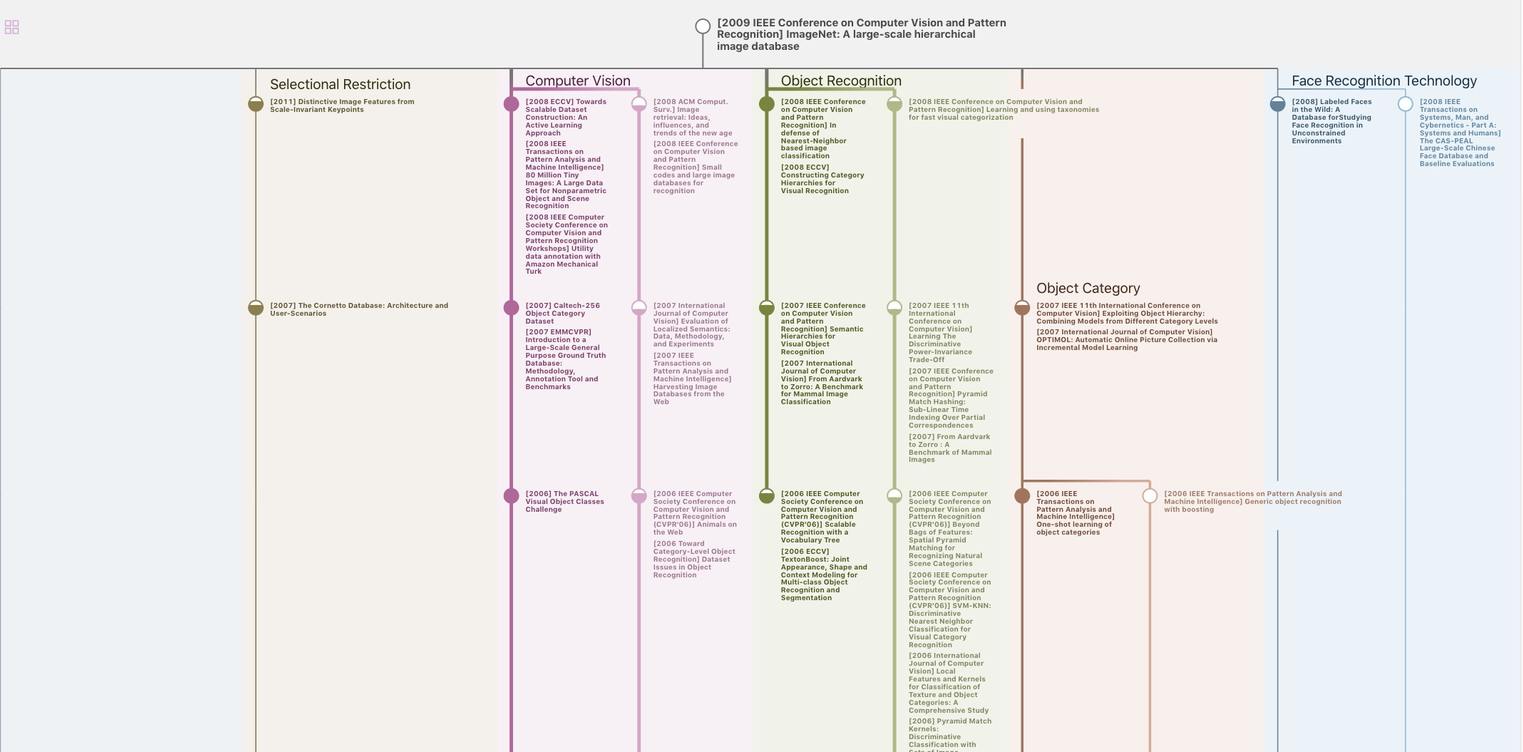
Generate MRT to find the research sequence of this paper
Chat Paper
Summary is being generated by the instructions you defined