MEDO: Minimizing Effective Distortions Only for Machine-Oriented Visual Feature Compression.
2023 IEEE International Conference on Visual Communications and Image Processing (VCIP)(2023)
摘要
In search for efficient feature compression technologies for machine consumption, MPEG recently issued a call for proposal (CfP) on feature compression for video coding for machine (FCVCM). One issue in feature compression is that the input feature maps generally have high redundancy in them. Various researches to reduce such redundancy have been made. For example, a recent study called L-MSFC (learnable multi-scale feature compression), which effectively combines multi-scale feature fusion and compression in an end-to-end learnable framework, showed up to 98% BD rate gain over the anchor model defined in the FCVCM CfP. Despite these advances in FCVCM, relation between distortions in feature maps and performance of vision tasks has stayed relatively unexplored. In this paper, we propose a novel loss function called MEDO (minimizing effective distortions only) based on our hypothesis that distortions below some threshold do not improve task performance. Experimental results on instance segmentation task show that our MEDO loss on top of L-MSFC improves the overall rate-mAP performance without compromising complexity. Being more practical for real-world uses, we also present an extension to L-MSFC for variable-rate support with a single model.
更多查看译文
关键词
feature compression,video coding for machine,feature fusion,effective distortions
AI 理解论文
溯源树
样例
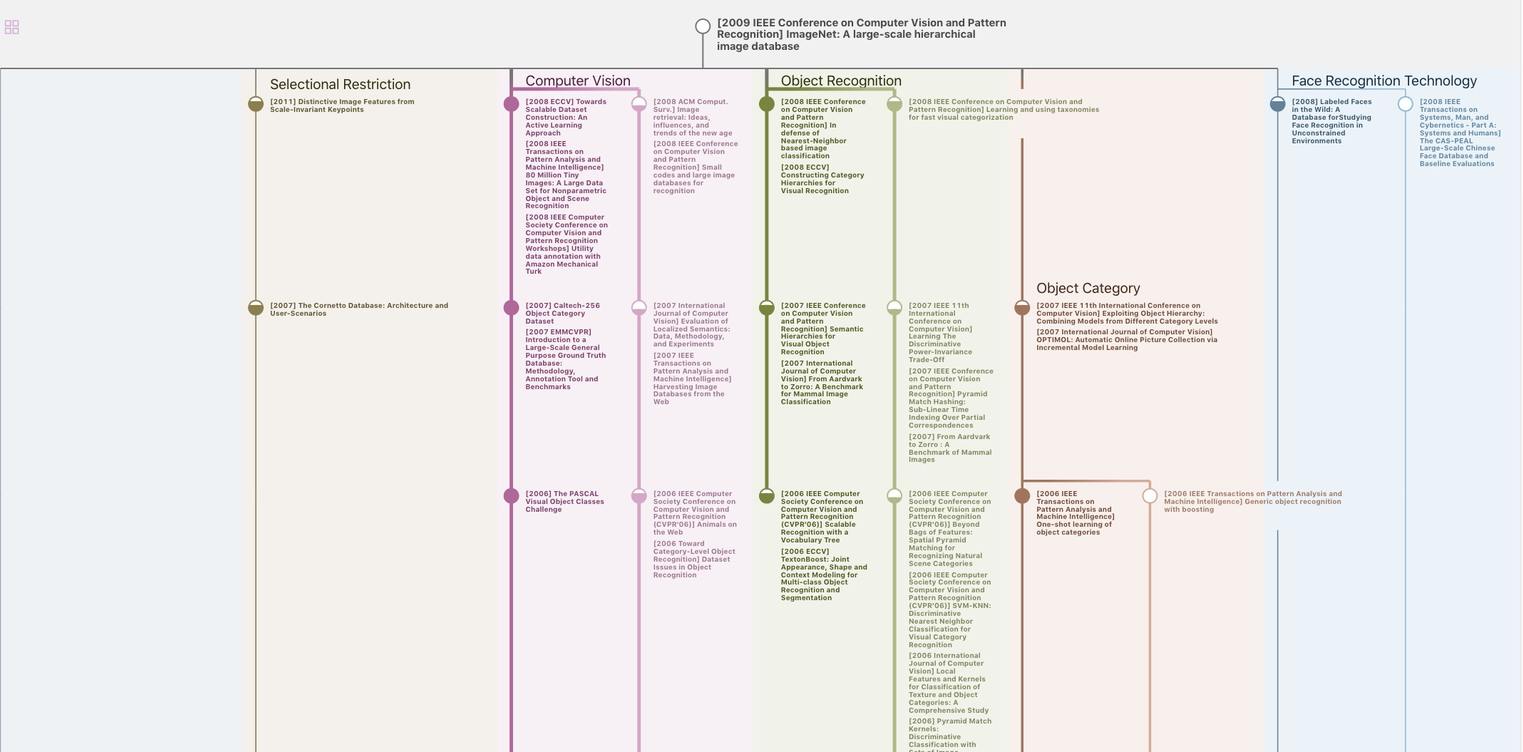
生成溯源树,研究论文发展脉络
Chat Paper
正在生成论文摘要