The learning costs of Federated Learning in constrained scenarios.
International Conference on Future Internet of Things and Cloud(2023)
摘要
Recently, machine-learning model sizes have grown considerably. Consequently, training the model on a single high-end machine can take up to several months. Since waiting such a long time is not feasible for most practical applications, a new approach was proposed. This approach, named distributed learning, leverages multiple computing nodes on a single machine or distributed across several to reduce the training times and is usually applied in high-performance computing clusters with centralized data. However, one of its implementations, federated learning, aims to take the training closer to the data sources. This approach applies the same algorithms as distributed learning but uses machines with limited hardware resources and communication capabilities. Although the advantages of these algorithms are well known, such as increased data privacy, they also have costs, as they create network overhead and require synchronization between devices. To that extent, understanding the trade-offs of federated learning is necessary to enable its correct deployment. This paper analyzes the trade-offs of implementing federated learning by deploying a small test bed of Raspberry Pis connected over a gigabit network. The results show that training on a single device is, on average, 2.99% faster when compared with distributed approaches.
更多查看译文
关键词
Distributed learning,Federated learning,Network Slicing
AI 理解论文
溯源树
样例
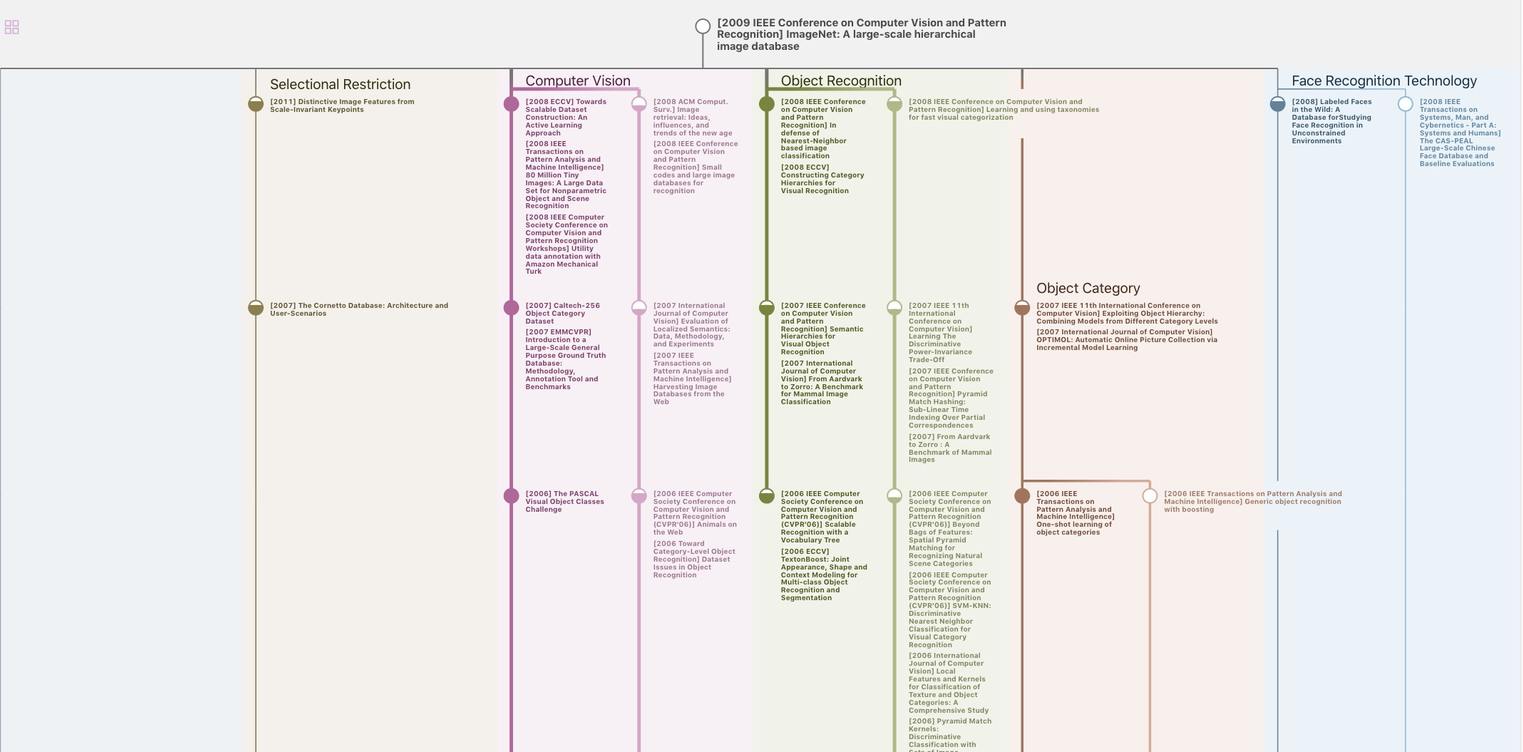
生成溯源树,研究论文发展脉络
Chat Paper
正在生成论文摘要