Dynamic Social Recommendation with High-Matching Inhomogeneous Relations.
2023 IEEE International Conference on Systems, Man, and Cybernetics (SMC)(2023)
摘要
Social recommendation utilizing social relations as auxiliary information can alleviate data sparsity issues of collaborative filtering methods. However, existing social recommendation methods usually focus on the shallow relations of user-item interactions and social networks to mine potential user preferences, which fail to provide a fine-grained consideration of the inhomogeneous influence and the changes in user interest or item attractiveness over time in high-order interactions of multiple relation types. To address these issues, we propose a novel method named Dynamic Social Recommendation with High-matching Inhomogeneous Relations (DSRHIR) to reveal the influence of inhomogeneity in dynamic interactions on social recommendation from multiple perspectives. Specifically, we first model the short-term dynamic and long-term static representations of both users with social relations and similar relations, and items with correlations. Then, we combine their dynamic and static representations during the interaction and the information from the high-order neighbors of these three relations to obtain a graph-augmented representation. Finally, the graph-augmented representation is used to modulate high-matching user interest and item attractiveness. Extensive experiments on two real-world datasets demonstrate the effectiveness of DSRHIR.
更多查看译文
关键词
Recommender systems,social networks,inhomogeneous relations,graph neural network
AI 理解论文
溯源树
样例
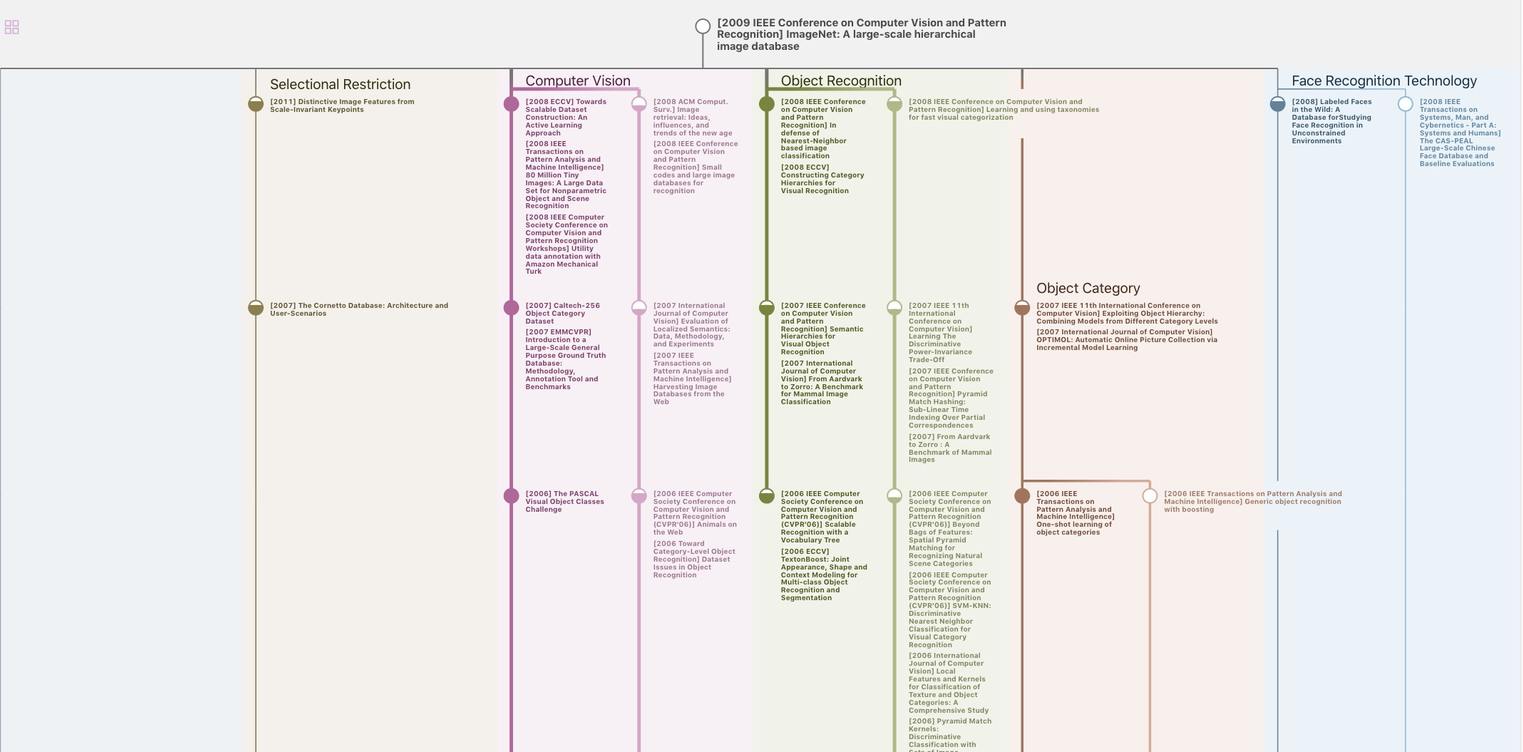
生成溯源树,研究论文发展脉络
Chat Paper
正在生成论文摘要