Stroke classification based on deep reinforcement learning over stroke screening imbalanced data
Computers and Electrical Engineering(2024)
摘要
Stroke screening is a crucial measure for reducing stroke occurrence, disability, and mortality. However there are numerous risk factors and the limited number of high-risk stroke groups in all screening populations, the screening data is redundant and imbalanced. We propose an improved feature selection algorithm to identify stroke key risk factors and an oversampling MRF-SMOTE algorithm to balance data. Then, a deep reinforcement learning classification model based on the Dueling DQN (Deep Q-network) algorithm is constructed for stroke classification, with the optimized loss function. Benchmark models include KNN, SVM, random forest, and Dueling DQN. Stroke screening data is pre-processed by selecting key risk factors and oversampled by MRF-SMOTE. Experiments show that the optimized Dueling DQN model can classify the pre-processed stroke screening data in terms of accuracy, AUC, precision, and F1-measure, which are 0.8982, 0.96, 0.899, and 0.8981 and improved by 4.37 %, 6.92 %, 3.78 % and 4.04 %, respectively, compared with the existing Dueling DQN.
更多查看译文
关键词
Stroke classification,Stroke key risk factors selection,Deep reinforcement learning model,Imbalanced data
AI 理解论文
溯源树
样例
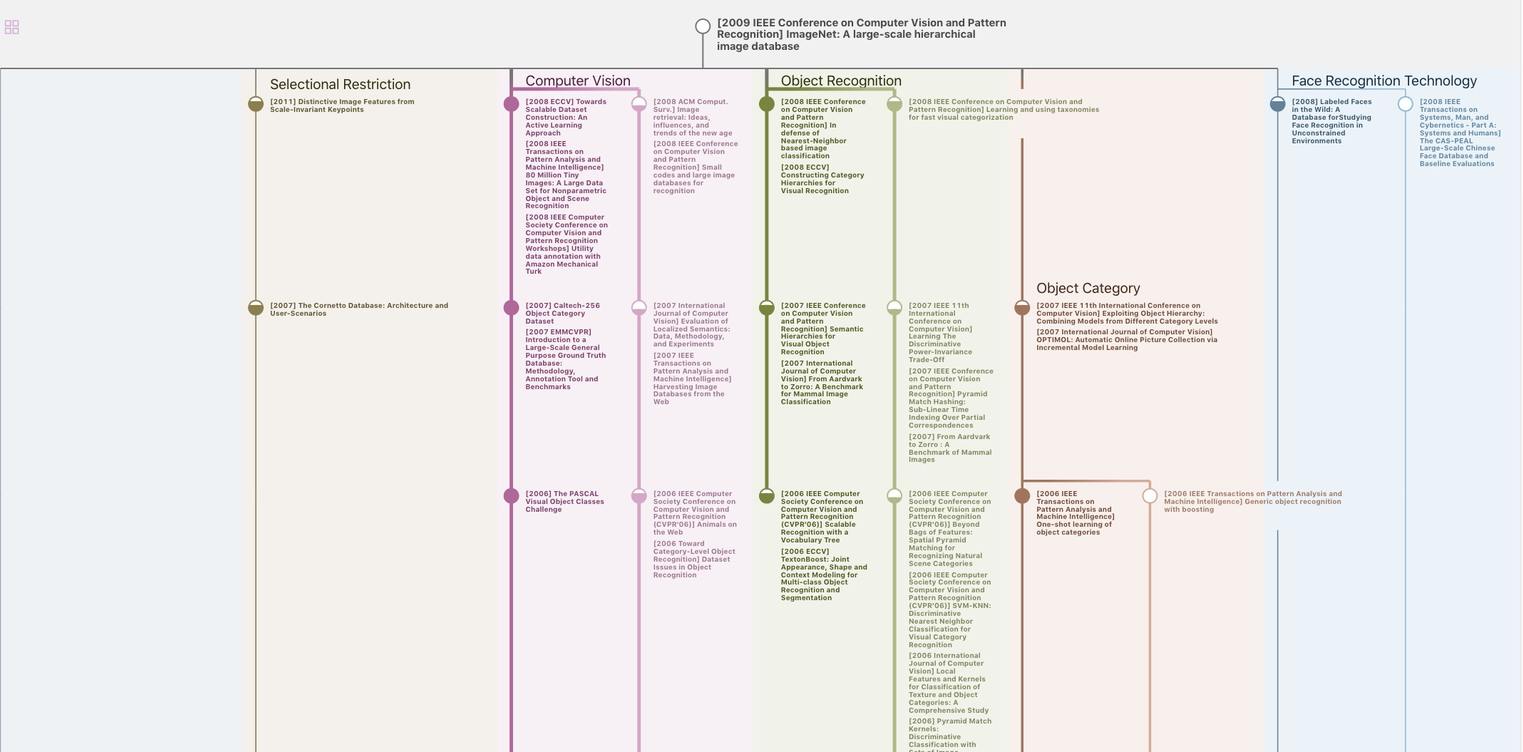
生成溯源树,研究论文发展脉络
Chat Paper
正在生成论文摘要