DiffuserLite: Towards Real-time Diffusion Planning
CoRR(2024)
摘要
Diffusion planning has been recognized as an effective decision-making
paradigm in various domains. The high-quality conditional generation capability
of long-horizon trajectories makes it a promising research direction. However,
existing diffusion planning methods suffer from low decision-making frequencies
because of the expensive iterative sampling cost. To address this issue, we
introduce DiffuserLite, a fast and lightweight diffusion planning framework.
DiffuserLite employs a planning refinement process (PRP) to generate
coarse-to-fine-grained trajectories, which significantly reduces the modeling
of redundant information and leads to notable increases in decision-making
frequency. Our experimental results demonstrate that DiffuserLite incurs only
0.88% of the runtime cost compared to previous frameworks, achieves an
average decision-making frequency of 122Hz, and reaches state-of-the-art
performance on D4RL benchmarks. In addition, our clean DiffuserLite framework
can serve as a flexible plugin to enhance decision frequency in other diffusion
planning algorithms, providing a structural design reference for future works.
More details and visualizations are available at [project
website](https://diffuserlite.github.io/).
更多查看译文
AI 理解论文
溯源树
样例
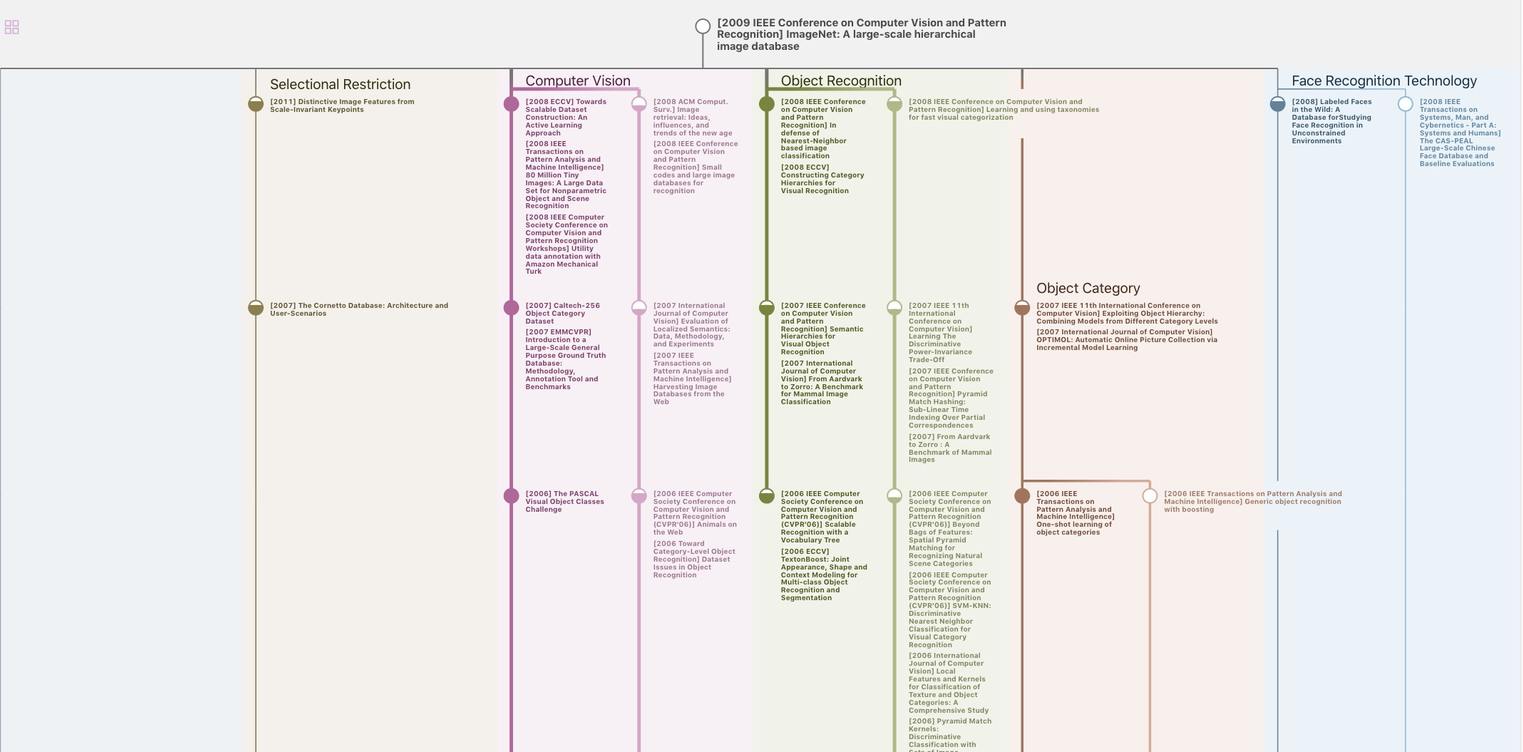
生成溯源树,研究论文发展脉络
Chat Paper
正在生成论文摘要