Deep Learning for Defect Detection in Inspection
openalex(2024)
摘要
Remanufacturing is the process of restoring a used product to the condition that satisfies the original performance specifications. One of the most critical stages of this process is inspection, which assesses the returned item's condition. Practically, the inspection process is carried out with manual or automated systems. Manual systems are generally expensive due to labor-intensive and time-consuming workloads. Additionally, they depend highly on the inspector's experience and psychophysical abilities, e.g., morning shift vs. night shift. With recent advancements in automated inspection enabled by machine vision and machine learning techniques, high inspection accuracy is possible without significant economic barriers. In this paper, we implement three object detection algorithms, YOLO v5s (small), Faster R-CNN ResNet101, and MobileNet-SSD with FPN, to classify and locate the defects encountered during remanufacturing. Using an industry-originated data set, the overall performance analysis and comparison results of three algorithms are presented for average accuracy metric. These initial results provide insights about the capability of the algorithms in defect detection as an alternative to human-operated systems and to understand which algorithm performs better under which circumstances. Overall, our findings will contribute to the design of future studies focusing on improvements in automated inspection technology.
更多查看译文
AI 理解论文
溯源树
样例
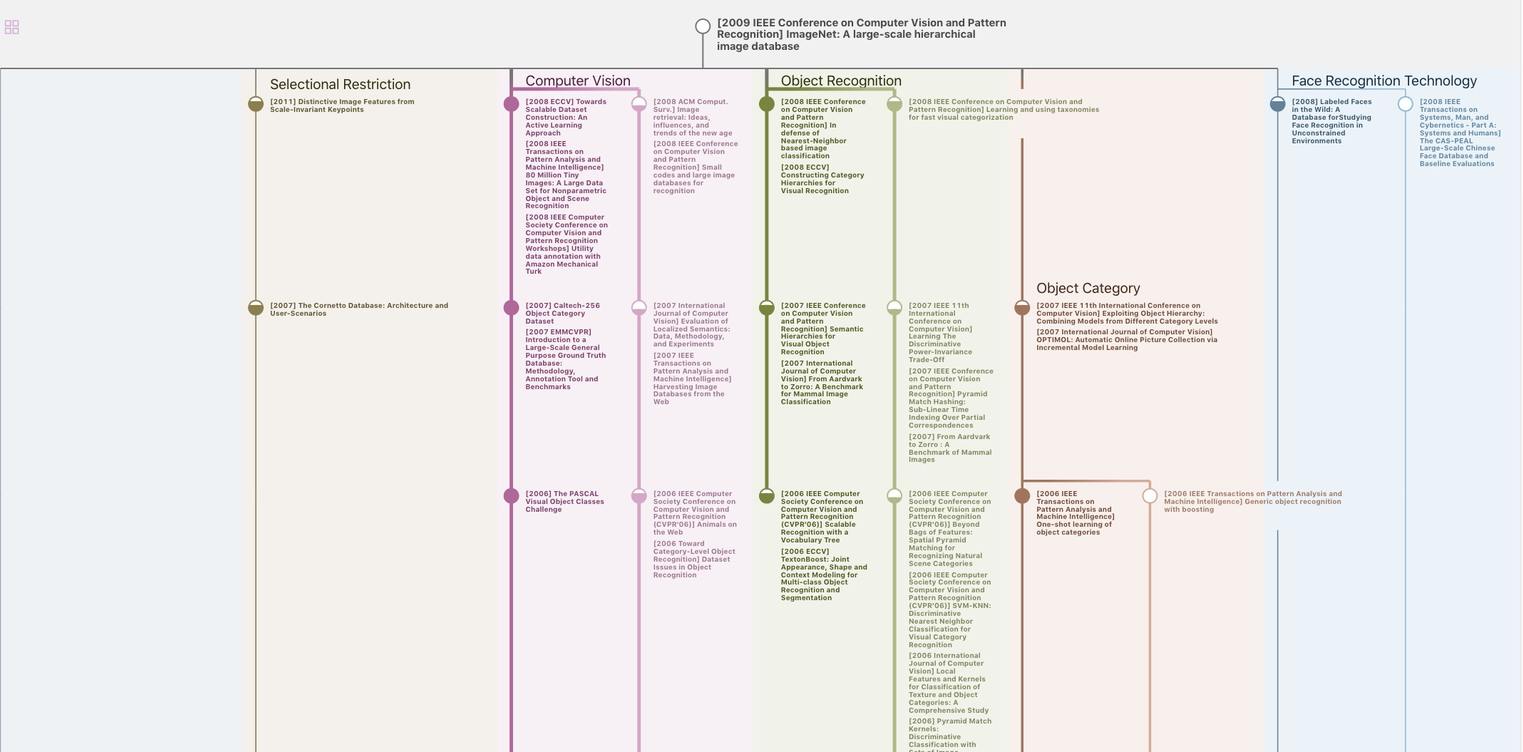
生成溯源树,研究论文发展脉络
Chat Paper
正在生成论文摘要