Enhancing the expressivity of quantum neural networks with residual connections
arxiv(2024)
摘要
In the recent noisy intermediate-scale quantum era, the research on the
combination of artificial intelligence and quantum computing has been greatly
developed. Inspired by neural networks, developing quantum neural networks with
specific structures is one of the most promising directions for improving
network performance. In this work, we propose a quantum circuit-based algorithm
to implement quantum residual neural networks (QResNets), where the residual
connection channels are constructed by introducing auxiliary qubits to the
data-encoding and trainable blocks of the quantum neural networks. Importantly,
we prove that when this particular network architecture is applied to a
l-layer data-encoding, the number of frequency generation forms can be
extended from one, namely the difference of the sum of generator eigenvalues,
to 𝒪(l^2). And the flexibility in adjusting the corresponding
Fourier coefficients can also be improved due to the diversity of spectrum
construction methods and the additional optimization degrees of freedom in the
generalized residual operators. These results indicate that the residual
encoding scheme can achieve better spectral richness and enhance the
expressivity of various parameterized quantum circuits. Extensive numerical
demonstrations in regression tasks of fitting various functions and
applications in image classification with MNIST datasets are offered to present
the expressivity enhancement. Our work lays the foundation for a complete
quantum implementation of the classical residual neural networks and explores a
new strategy for quantum feature map in quantum machine learning.
更多查看译文
AI 理解论文
溯源树
样例
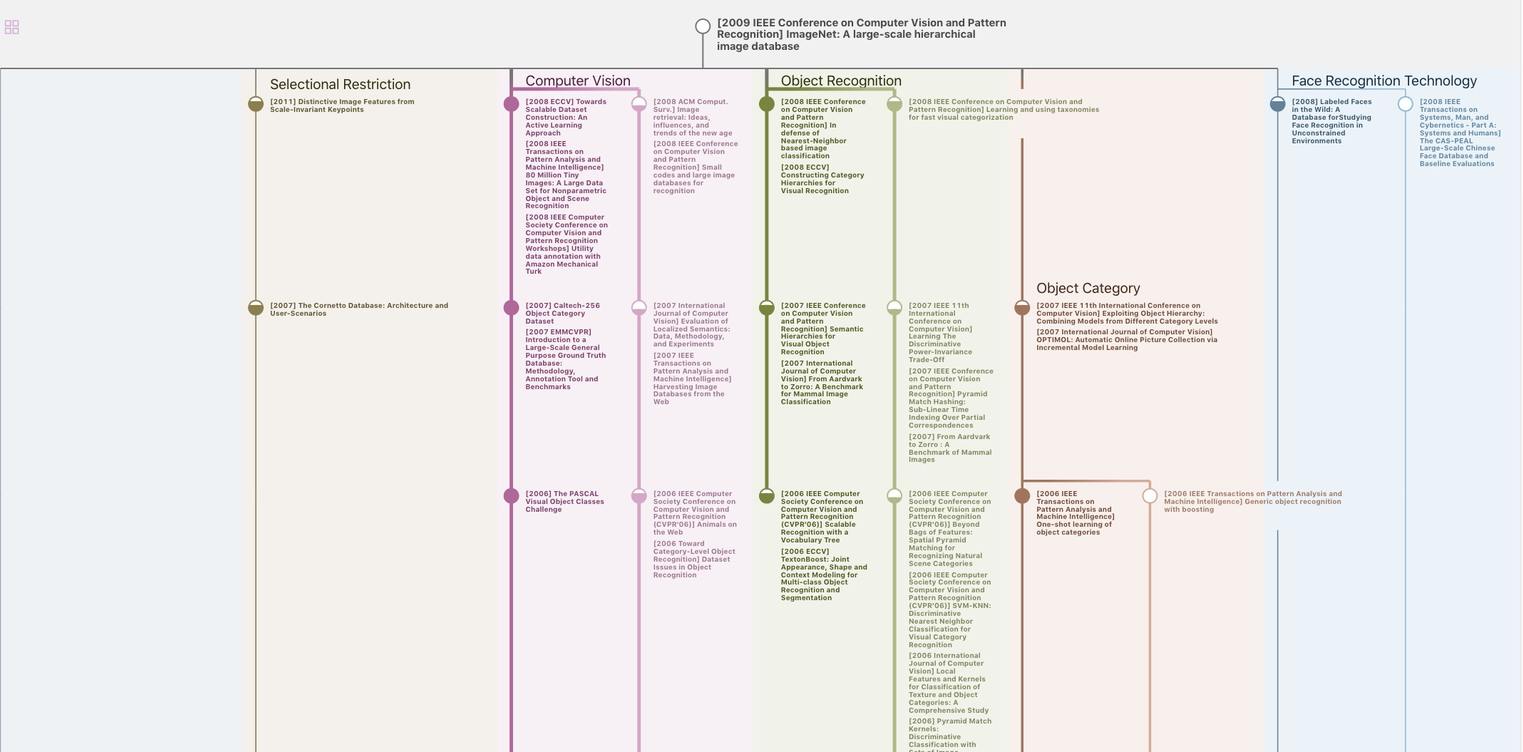
生成溯源树,研究论文发展脉络
Chat Paper
正在生成论文摘要