FeDDkw – Federated Learning with Dynamic Kullback–Leibler-divergence WeightJust Accepted
ACM Transactions on Asian and Low-Resource Language Information Processing(2022)
摘要
Federated learning (FL) has emerged as a promising framework for collaborative machine learning. As one of the most well-known bottlenecks of FL, data heterogeneity, i.e., non-IID data, has seriously hampered the convergence rate and model accuracy of FL. Although there are many works that aim to deal with this problem, none of them have directly exploited the data heterogeneity information, and thus cannot handle the problem effectively in general. In this paper, we try to answer the following fundamental question, whether this data heterogeneity information can be utilized to enhance the system performance. To this end, we propose FedDkw – Federated Learning with Dynamic KL-divergence Weight. Specifically, in every round, while uploading the model to the server, each participated client also piggybacks the distribution of the training data. The server maintains a global distribution, which is updated after receiving the distributions of all clients in each round. Then the weight of each client i is proportional to the \(\frac{1}{KL(i)} \), where KL ( i ) is the Kullback-Leibler (KL) divergence between the client’s distribution and the global counterpart. This indicates that the clients whose distribution is closer to the global one should be assigned with a larger weight, which coincides with our intuition. Furthermore, since uploading the clients’ data distribution in FedDkw may bring about potential security risks, we further propose FedDkw++ to avoid this procedure. In particular, after each client uploads its model, the server uses its local data as the input to the model, and the obtained outcome is used as an estimation of the client’s distribution (we name this method “data distribution inference”). We have conducted extensive experiments in various scenarios. The results show that our algorithm can significantly accelerate the convergence rate than the current state-of-art algorithms under heterogeneous data.
更多查看译文
关键词
datasets,neural networks,gaze detection,text tagging
AI 理解论文
溯源树
样例
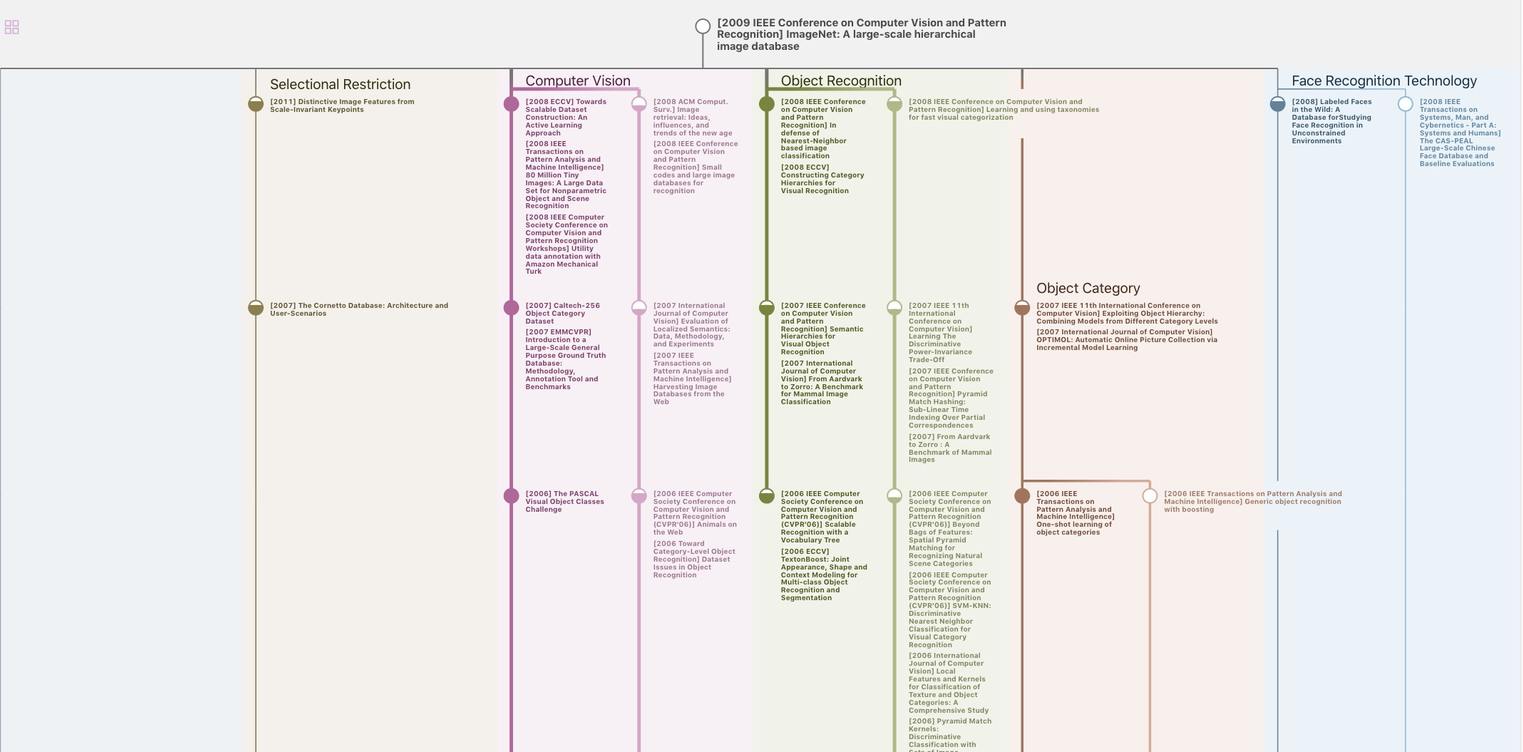
生成溯源树,研究论文发展脉络
Chat Paper
正在生成论文摘要