MCFNet: Multi-Attentional Class Feature Augmentation Network for Real-Time Scene ParsingJust Accepted
ACM Transactions on Multimedia Computing, Communications, and Applications(2023)
摘要
For real-time scene parsing tasks, capturing multi-scale semantic features and performing effective feature fusion is crucial. However, many existing solutions ignore stripe-shaped things like poles, traffic lights and are so computationally expensive that cannot meet the high real-time requirements. This paper presents a novel model, the Multi-Attention Class Feature Augmentation Network (MCFNet) to address this challenge. MCFNet is designed to capture long-range dependencies across different scales with low computational cost and to perform a weighted fusion of feature maps. It features the BAM (Strip Matrix Based Attention Module) for extracting strip objects in images. The BAM module replaces the conventional self-attention method using square matrices with strip matrices, which allows it to focus more on strip objects while reducing computation. Additionally, MCFNet has a parallel branch that focuses on global information based on self-attention to avoid wasting computation. The two branches are merged to enhance the performance of traditional self-attention modules. Experimental results on two mainstream datasets demonstrate the effectiveness of MCFNet. On the Camvid and Cityscapes test sets, MCFNet achieved 207.5 FPS/73.5% mIoU and 136.1 FPS/71.63% mIoU, respectively. The experiments show that MCFNet outperforms other models on the Camvid dataset and can significantly improve the performance of real-time scene parsing tasks.
更多查看译文
关键词
Computer Vision,CNN,Real-time Semantic Segmentation,Attention Mechanism
AI 理解论文
溯源树
样例
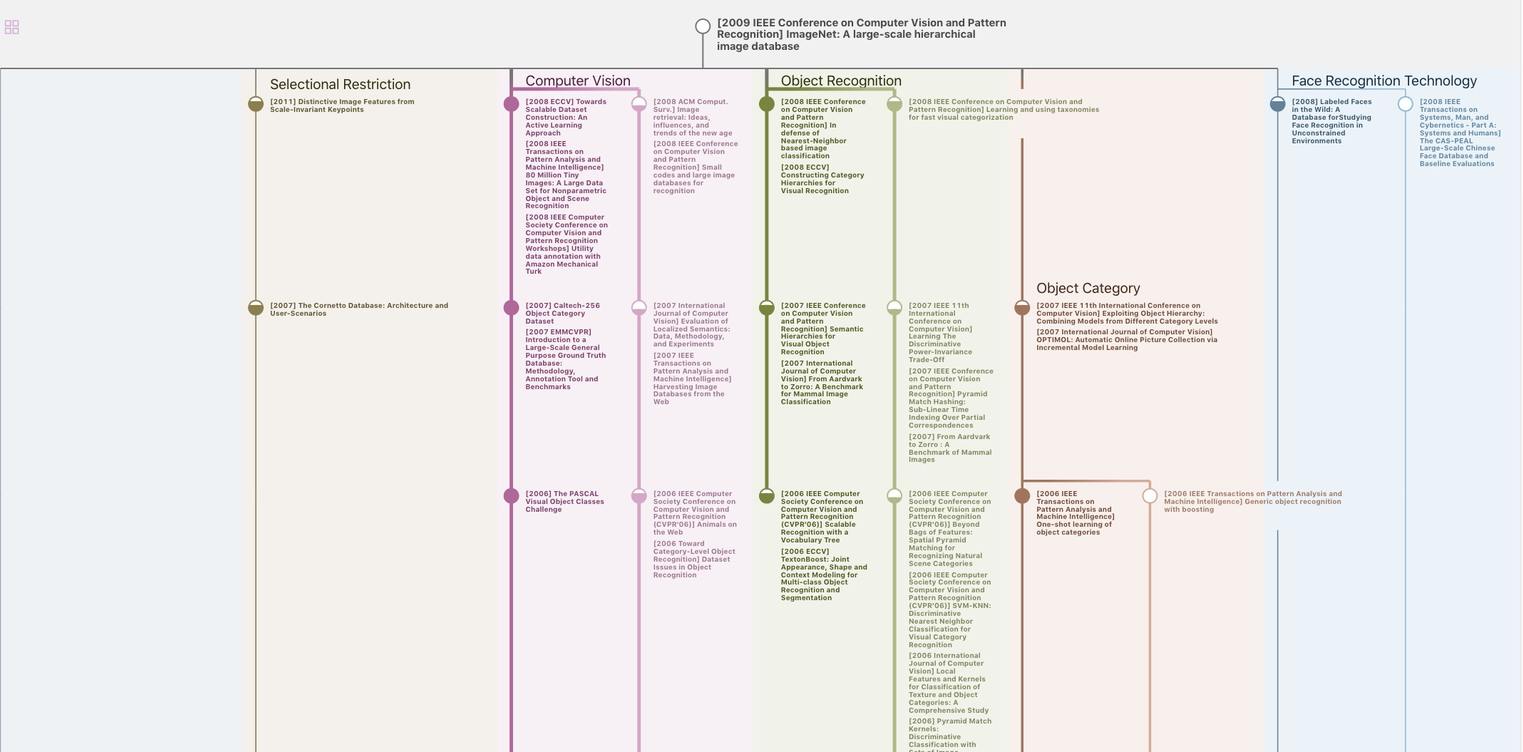
生成溯源树,研究论文发展脉络
Chat Paper
正在生成论文摘要