Particle dispersion for indoor air quality control considering air change approach: A novel accelerated CFD-DNN prediction
ENERGY AND BUILDINGS(2024)
摘要
Computational Fluid Dynamics (CFD) is a well-established tool to study fluid dynamics and particle movement, while Artificial Neural Network (ANN) models offer machine learning capabilities to accelerate indoor airflow predictions, but they still maintain a reasonable level of accuracy for prediction purposes. This study pioneers the integration of Deep Neural Network (DNN) models into indoor airflow dynamics, aiming to provide an accurate and accelerated prediction efficiency. The objective is to train two DNN models (classical and modified DNN models) to capture the complex relationships between ventilation rate, airflow patterns, and particle dispersion characteristics within buildings. Using a dataset generated from CFD simulations encompassing various air change rates, the trained modified DNN model significantly enhances prediction efficiency in term of the computational cost by 67 % reduction of CFD computational time (1 h to 20 min) while also resulting in very similar accuracy compared to the CFD outputs. The R2 values of classical and modified DNN models (plane 1) at air flow rate equals to 4 ach are 0.6867 and 0.9567 in term of the DPM distribution, respectively. The similar pattern is observed as the accuracy of modified DNN is higher than the classical DNN for other air flow rates in terms of the DPM and velocity distributions. Accordingly, the number of prediction errors is significantly decreased as the model alters from the classical DNN to modified DNN model. The significance of this research lies in its potential to enhance the efficiency of assessing particle dispersion, allowing for the more efficient design of targeted ventilation strategies and indoor air quality control measures tailored to diverse pollutant sources emitted from humans. Integrating DNN and CFD in assessing particle dispersion characteristics is promising for improving the understanding of indoor air dynamics and facilitating data-driven decision-making for ensuring healthier and safer indoor environments.
更多查看译文
关键词
Computational fluid dynamics (CFD),Artificial Neural Network (ANN),Deep Neural Network (DNN),Indoor air quality (IAQ),Ventilation approach,Infection disease,Airborne particle dispersion
AI 理解论文
溯源树
样例
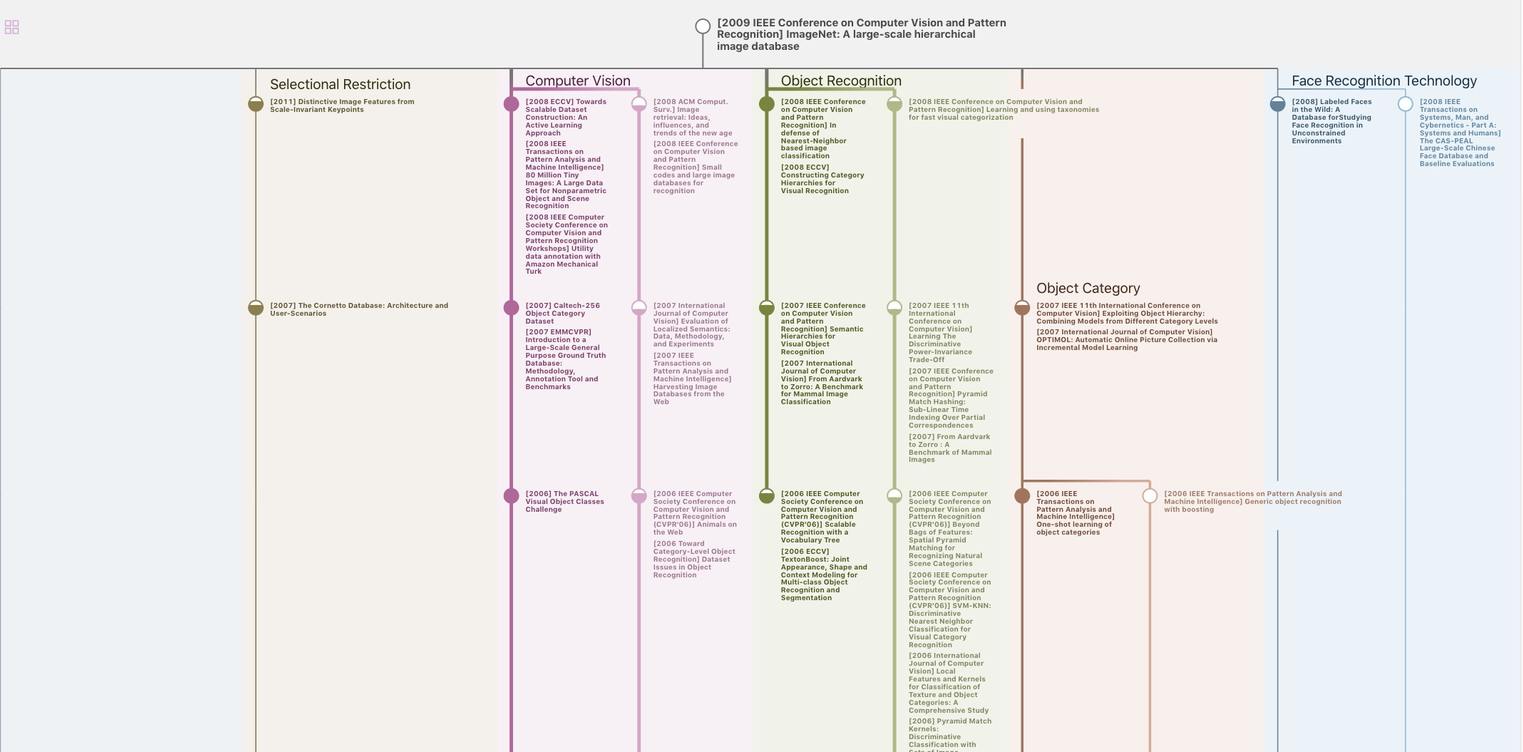
生成溯源树,研究论文发展脉络
Chat Paper
正在生成论文摘要