Splitting tensile strength of basalt fiber reinforced coral aggregate concrete: Optimized XGBoost models and experimental validation
CONSTRUCTION AND BUILDING MATERIALS(2024)
摘要
The split tensile strength of basalt fiber-reinforced coral aggregate concrete (BFRCAC-SS) is a critical parameter in structural design because it directly affects the load-bearing capacity and durability of BFRCAC structures. BFRCAC-SS is influenced by multiple variables, and the accuracy and generalization capability of traditional explicit models for predicting BFRCAC-SS with individual variables are limited. Therefore, this study involved collecting 313 data sets from 14 articles to establish a comprehensive BFRCAC-SS database. The hyperparameters (iteration count, tree depth, and learning rate) of the XGBoost algorithm were optimized using prairie dog optimization, hunger games search, and egret swarm optimization (ESOA) algorithms. Consequently, three optimized XGBoost models for BFRCAC-SS were developed. Furthermore, feature importance was analyzed using the Shapley additive explanation method. The performance of the optimized XGBoost model was subsequently validated through experimental testing. Results indicate that the ESOA-XGBoost model provides predictions that are closer to the actual values, with smaller mean errors and standard deviations. The performance indicators, including coefficient of determination, mean absolute error, mean absolute percentage error, mean square error, and root mean square error, of the ESOA-XGBoost model are 0.9633, 0.1002, 2.8862, 0.0188, and 0.1373, respectively, and are superior to those of the other tested models. Curing time and the water-binder ratio are identified as the two most critical factors. Prolonging curing time and reducing the water-binder ratio enhance the BFRCAC-SS. A graphical user interface for BFRCAC-SS is developed on the basis of the ESOA-XGBoost model, which enables the visualization of BFRCAC-SS predictions. Furthermore, the relative error between the experimental and predicted values consistently remains below 5%, which highlights the strong generalization and accuracy of the ESOA-XGBoost model.
更多查看译文
关键词
Basalt fiber,Coral aggregate concrete,Split tensile strength,Shapley additive explanations,Machine learning,Egret swarm optimization algorithm
AI 理解论文
溯源树
样例
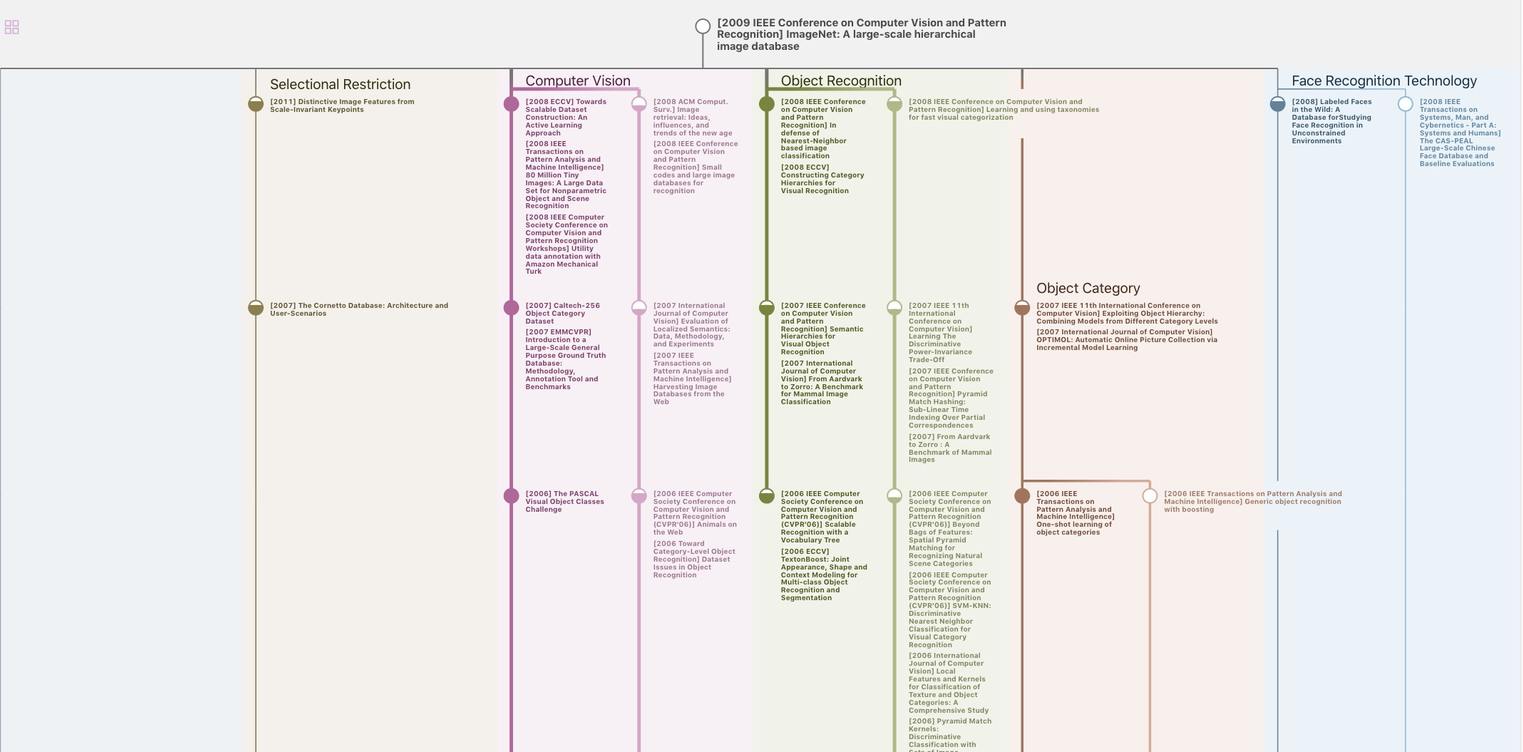
生成溯源树,研究论文发展脉络
Chat Paper
正在生成论文摘要