Towards Temporal Information Processing - Printed Neuromorphic Circuits with Learnable Filters.
IEEE/ACM International Symposium on Nanoscale Architectures(2023)
摘要
Many consumer products such as wearable and disposable electronics require flexibility, biocompatibility and ultra low-costs, which can hardly be matched by silicon electronics. Therefore, printed electronics (PE) becomes a competitive candidate thanks to its additive manufacturing. To address fundamental signal-processing tasks, printed neuromorphic circuits (pNCs) have received increasing attention, as they can achieve promising computational capabilities by assembling simple circuit primitives. However, many target domains of PE are based on processing temporal sensory data, which can not be reached by existing pNCs, since they lack components with time dependencies. This paper proposes a printed temporal processing block that combines existing pNCs with learnable filters. We model the proposed circuit and proposed the corresponding training objective to enable their bespoke design. Simulations on 15 benchmark time-series datasets reveal that, the proposed circuits can effectively process temporal data by using 1.5 × and 1.3 × of device counts and power respectively. The classification accuracy reaches 98% of that from classic Elman recurrent neural networks.
更多查看译文
AI 理解论文
溯源树
样例
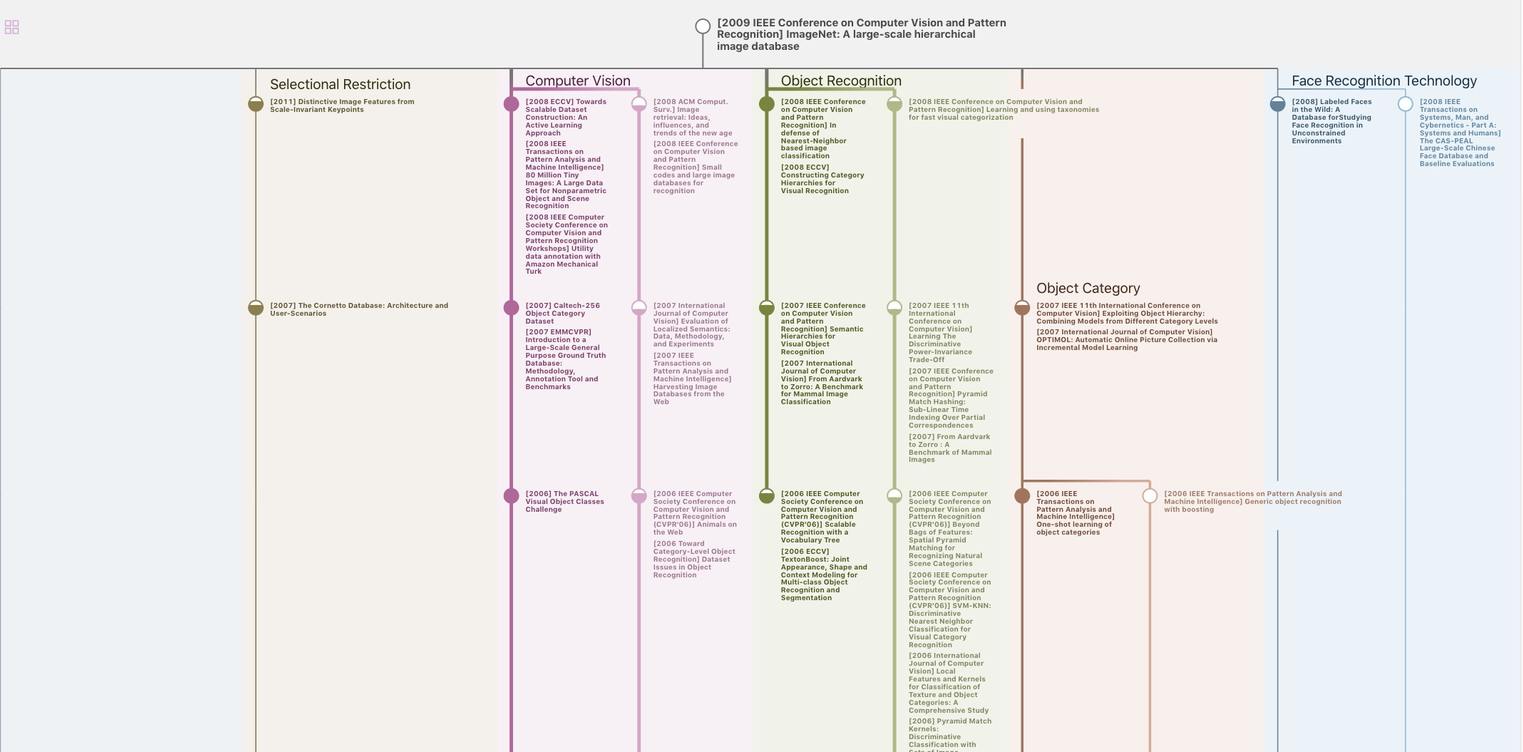
生成溯源树,研究论文发展脉络
Chat Paper
正在生成论文摘要