Comparison of Bayesian approaches for developing prediction models in rare disease: application to the identification of patients with Maturity-Onset Diabetes of the Young
medrxiv(2024)
摘要
Background Clinical prediction models can help identify high-risk patients and facilitate timely interventions. However, developing such models for rare diseases presents challenges due to the scarcity of affected patients for developing and calibrating models. Methods that pool information from multiple sources can help with these challenges.
Methods We compared three approaches for developing clinical prediction models for population-screening based on an example of discriminating a rare form of diabetes (Maturity-Onset Diabetes of the Young - MODY) in insulin-treated patients from the more common Type 1 diabetes (T1D). Two datasets were used: a case-control dataset (278 T1D, 177 MODY) and a population-representative dataset (1418 patients, 96 MODY tested with biomarker testing, 7 MODY positive). To build a population-level prediction model, we compared three methods for recalibrating models developed in case-control data. These were: prevalence adjustment (“offset”), shrinkage recalibration in the population-level dataset (“recalibration”), and a refitting of the model to the population-level dataset (“re-estimation”). We then developed a Bayesian hierarchical mixture model combining shrinkage recalibration with additional informative biomarker information only available in the population-representative dataset. We developed prior information from the literature and other data sources to deal with missing biomarker and outcome information, and to ensure clinical validity of predictions for certain biomarker combinations.
Results The offset, re-estimation, and recalibration methods showed good calibration in the population-representative dataset. Among these, the offset and recalibration methods displayed the lowest predictive uncertainty, due to borrowing information from the fitted case-control model. We demonstrate the potential of a mixture model for incorporating informative biomarkers, which significantly enhanced the model’s predictive accuracy, reduced uncertainty, and showed higher stability in all ranges of predictive outcome probabilities.
Conclusion We have compared several approaches that could be used to develop prediction models for rare diseases. Our findings highlight the recalibration mixture model as the optimal strategy if a population-level dataset is available. This approach offers the flexibility to incorporate additional predictors and informed prior probabilities, contributing to enhanced prediction accuracy for rare diseases, and allows predictions without these additional tests, providing additional information on whether a patient should undergo further biomarker testing before genetic testing.
### Competing Interest Statement
The authors have declared no competing interest.
### Funding Statement
PC and TJM (McKinley) are funded by Research England's Expanding Excellence in England (E3) fund. KAP is a Wellcome Trust fellow (219606/Z/19/Z). This work was further supported by Diabetes UK (reference 21/0006328), the National Institute for Health and Care Research (NIHR) Exeter Biomedical Research Centre (BRC) and the National Institute for Health and Care Research Exeter Clinical Research Facility. The views expressed are those of the author(s) and not necessarily those of the NHS, the NIHR, the Wellcome Trust or the Department of Health and Social Care.
### Author Declarations
I confirm all relevant ethical guidelines have been followed, and any necessary IRB and/or ethics committee approvals have been obtained.
Yes
The details of the IRB/oversight body that provided approval or exemption for the research described are given below:
For the UNITED study, ethics approval was granted by the NRES Committee South West-Central Bristol (REC no. 10/H0106/03). For the case control data, this study was approved by the Genetic Beta Cell Research Bank, Exeter, UK (ethical approval was provided by the North Wales Research Ethics Committee, UK (IRAS project ID 231760)) and the Peninsula Research Bank (Devon & Torbay Research Ethics Committee, ref: 2002/7/118).
I confirm that all necessary patient/participant consent has been obtained and the appropriate institutional forms have been archived, and that any patient/participant/sample identifiers included were not known to anyone (e.g., hospital staff, patients or participants themselves) outside the research group so cannot be used to identify individuals.
Yes
I understand that all clinical trials and any other prospective interventional studies must be registered with an ICMJE-approved registry, such as ClinicalTrials.gov. I confirm that any such study reported in the manuscript has been registered and the trial registration ID is provided (note: if posting a prospective study registered retrospectively, please provide a statement in the trial ID field explaining why the study was not registered in advance).
Yes
I have followed all appropriate research reporting guidelines, such as any relevant EQUATOR Network research reporting checklist(s) and other pertinent material, if applicable.
Yes
Data are available through application to the Genetic Beta Cell Research Bank and the Peninsula Research Bank . Example R code for fitting the approaches used in this study is available on GitHub within the repository "Exeter-Diabetes/Pedro-MODY\_recal\_approaches".
[https://github.com/Exeter-Diabetes/Pedro-MODY\_recal\_approaches][1]
[1]: https://github.com/Exeter-Diabetes/Pedro-MODY_recal_approaches
更多查看译文
AI 理解论文
溯源树
样例
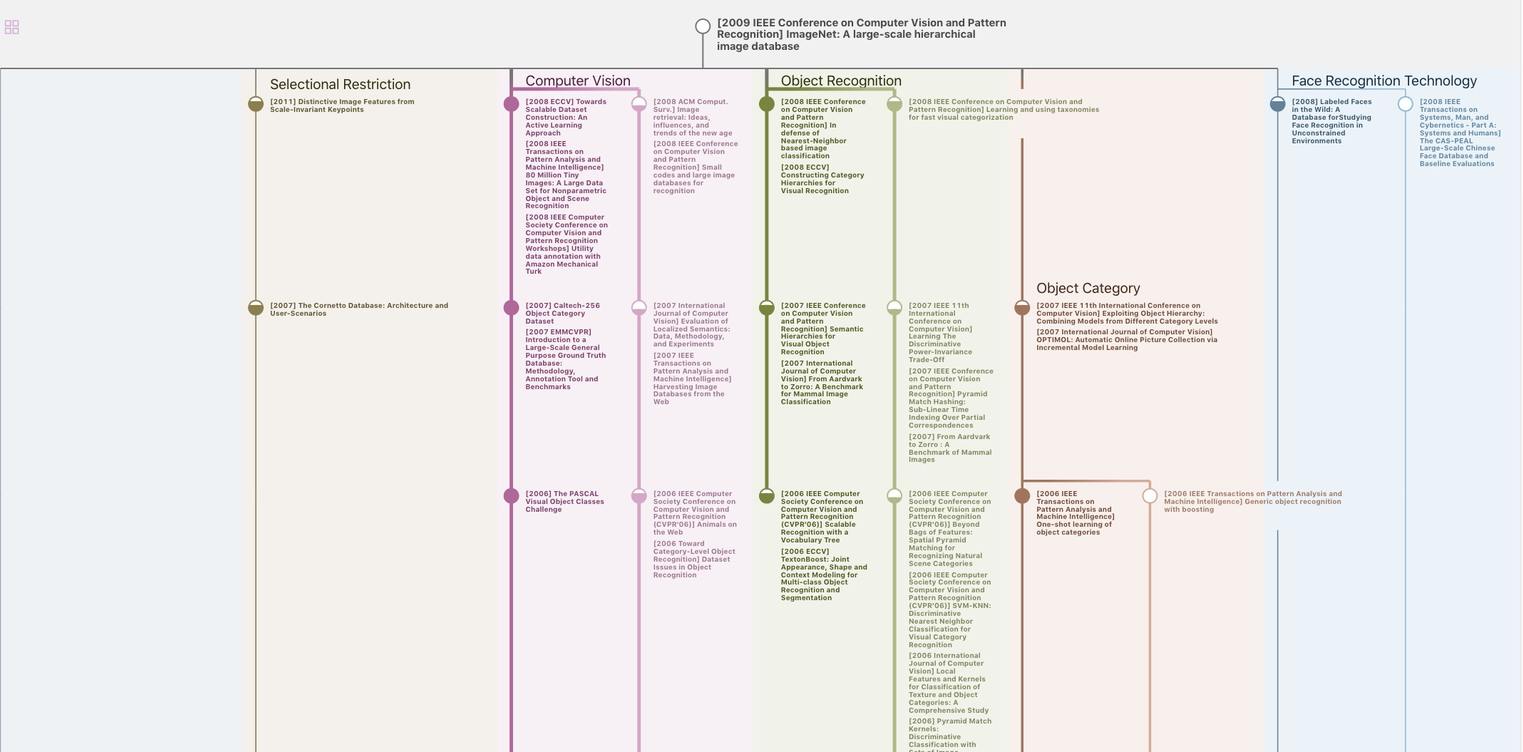
生成溯源树,研究论文发展脉络
Chat Paper
正在生成论文摘要