Learning When to See for Long-term Traffic Data Collection on Power-constrained Devices
2023 IEEE 26th International Conference on Intelligent Transportation Systems (ITSC)(2024)
摘要
Collecting traffic data is crucial for transportation systems and urban
planning, and is often more desirable through easy-to-deploy but
power-constrained devices, due to the unavailability or high cost of power and
network infrastructure. The limited power means an inevitable trade-off between
data collection duration and accuracy/resolution. We introduce a novel
learning-based framework that strategically decides observation timings for
battery-powered devices and reconstructs the full data stream from sparsely
sampled observations, resulting in minimal performance loss and a significantly
prolonged system lifetime. Our framework comprises a predictor, a controller,
and an estimator. The predictor utilizes historical data to forecast future
trends within a fixed time horizon. The controller uses the forecasts to
determine the next optimal timing for data collection. Finally, the estimator
reconstructs the complete data profile from the sampled observations. We
evaluate the performance of the proposed method on PeMS data by an RNN
(Recurrent Neural Network) predictor and estimator, and a DRQN (Deep Recurrent
Q-Network) controller, and compare it against the baseline that uses Kalman
filter and uniform sampling. The results indicate that our method outperforms
the baseline, primarily due to the inclusion of more representative data points
in the profile, resulting in an overall 10% improvement in estimation
accuracy. Source code will be publicly available.
更多查看译文
关键词
Traffic Data,Long-term Data Collection,Traffic Data Collection,Power-constrained Devices,Neural Network,Recurrent Neural Network,Limited Power,Kalman Filter,Battery Power,Network Infrastructure,Learning-based Framework,Improve Estimation Accuracy,Time Series Data,Object Detection,Mean Absolute Error,Long Short-term Memory,Autoregressive Model,Control Mode,Kriging,Video Data,Uniform Policy,Prediction Module,Part Of The Input,Adaptive Sampling,Mean Absolute Percentage Error,Model Predictive Control,Surveillance Cameras,Estimation Module,Markov Decision Process,Initial Days
AI 理解论文
溯源树
样例
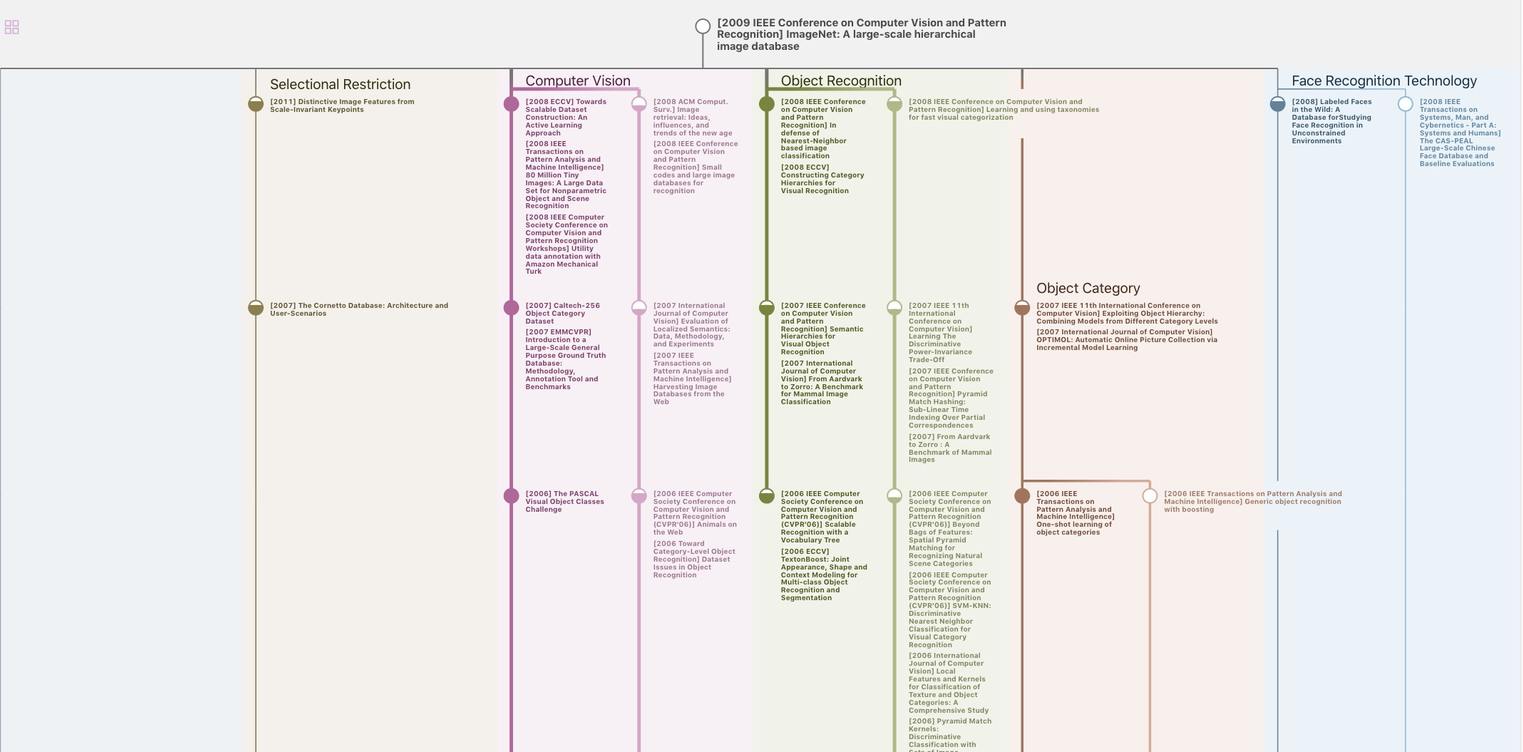
生成溯源树,研究论文发展脉络
Chat Paper
正在生成论文摘要