Multi agent reinforcement learning for online layout planning and scheduling in flexible assembly systems
Journal of Intelligent Manufacturing(2024)
摘要
Manufacturing systems are undergoing systematic change facing the trade-off between the customer's needs and the economic and ecological pressure. Especially assembly systems must be more flexible due to many product generations or unpredictable material and demand fluctuations. As a solution line-less mobile assembly systems implement flexible job routes through movable multi-purpose resources and flexible transportation systems. Moreover, a completely reactive rearrangeable layout with mobile resources enables reconfigurations without interrupting production. A scheduling that can handle the complexity of dynamic events is necessary to plan job routes and control transportation in such an assembly system. Conventional approaches for this control task require exponentially rising computational capacities with increasing problem sizes. Therefore, the contribution of this work is an algorithm to dynamically solve the integrated problem of layout optimization and scheduling in line-less mobile assembly systems. The proposed multi agent deep reinforcement learning algorithm uses proximal policy optimization and consists of a decoder and encoder, allowing for various-sized system state descriptions. A simulation study shows that the proposed algorithm performs better in 78% of the scenarios compared to a random agent regarding the makespan optimization objective. This allows for adaptive optimization of line-less mobile assembly systems that can face global challenges.
更多查看译文
关键词
Production control,Production scheduling,Layout optimization,Multi-agent deep reinforcement learning,Proximal policy optimization,Mobile resources,Flexible assembly
AI 理解论文
溯源树
样例
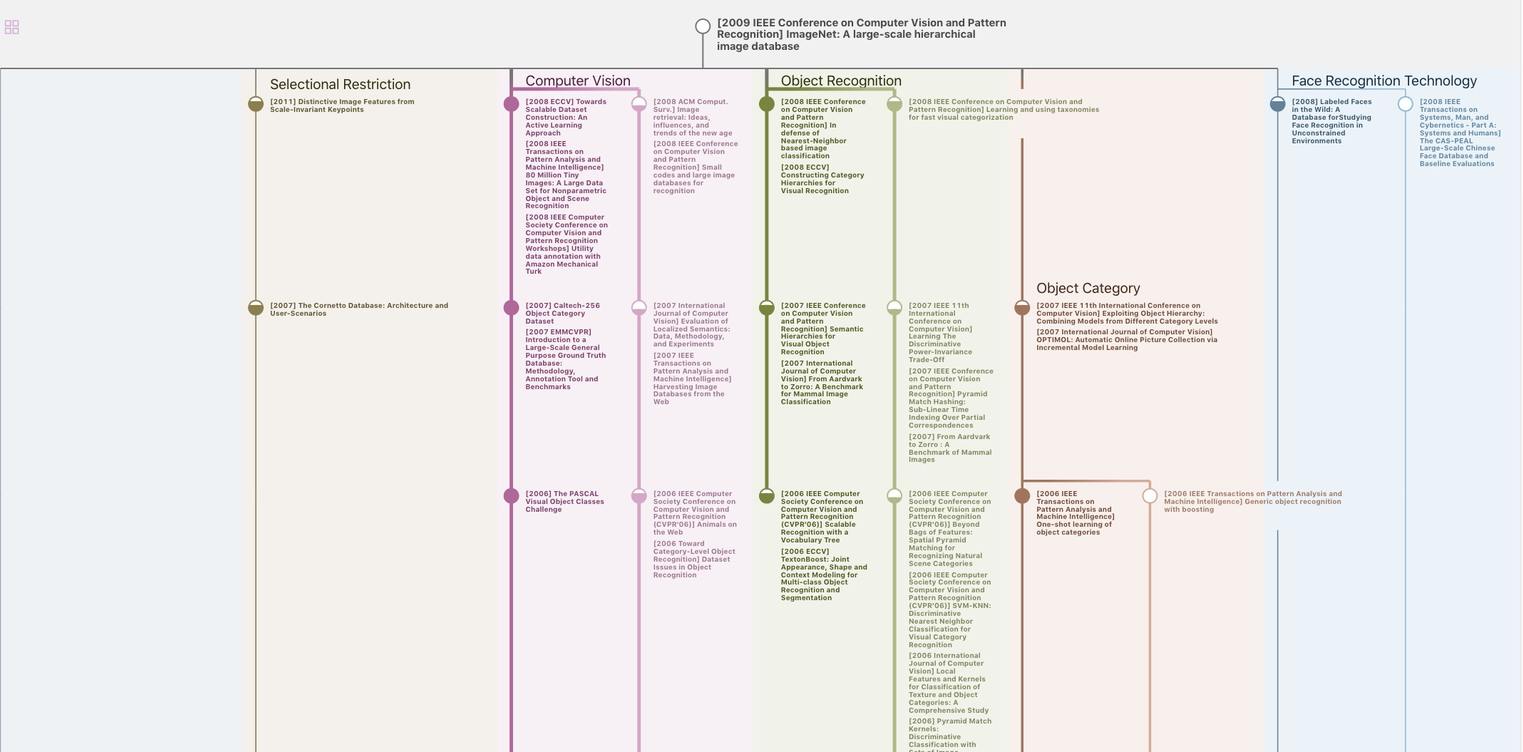
生成溯源树,研究论文发展脉络
Chat Paper
正在生成论文摘要