Feature Importance Estimation Using Gradient Based Method for Multimodal Fused Neural Networks
2022 IEEE Nuclear Science Symposium and Medical Imaging Conference (NSS/MIC)(2022)
摘要
The combination of multimodal data such as phenotype, clinical, and imaging data has been shown to improve performance in many medical machine learning applications. While intuition suggests that more information should help in many applications, there has been limited study interpreting the relative importance of combining multimodal features in deep neural networks. This work proposes a novel gradient based feature importance method for multimodal neural networks to help improve interpretability and to guide architecture selection. To demonstrate our method, we develop a validation platform to establish performance baselines; this platform simulates test cases and compares the performance of our feature importance method with ground truth. As a use case, we employ a hybrid fusion neural network to solve a binary classification problem with multiple image and non-image inputs. The gradient based method for feature importance is used in the post-fusion section of the architecture to obtain feature importances for deep features. These importances are then aggregated for each modality to obtain importance for each multimodal input. In our example application, we use 58830 abdominal CT scans from medMNIST with simulated clinical data for training. The ground truth labels and ground truth importances are obtained using a synthetic decision functions. All of our eight test cases show strong agreement with the ground truth ranks with an average rank biased overlap of 0.93 and a root mean squared error of around 0.13 across all modalities. This work represents among the first effort to estimate feature importance in multimodal machine learning models, paving the way for improved interpretability of deep learning methods.
更多查看译文
关键词
Neural Network,Important Characteristics,Feature Importance Estimation,Deep Learning,Learning Models,Simulated Data,Deep Neural Network,Ground Truth Labels,Binary Classification Problem,Abdominal Computed Tomography,Multimodal Learning,Multimodal Model,Multimodal Network,Linear Model,Convolutional Neural Network,Input Features,Artificial Intelligence Methods,Explainable Artificial Intelligence,Fusion Layer
AI 理解论文
溯源树
样例
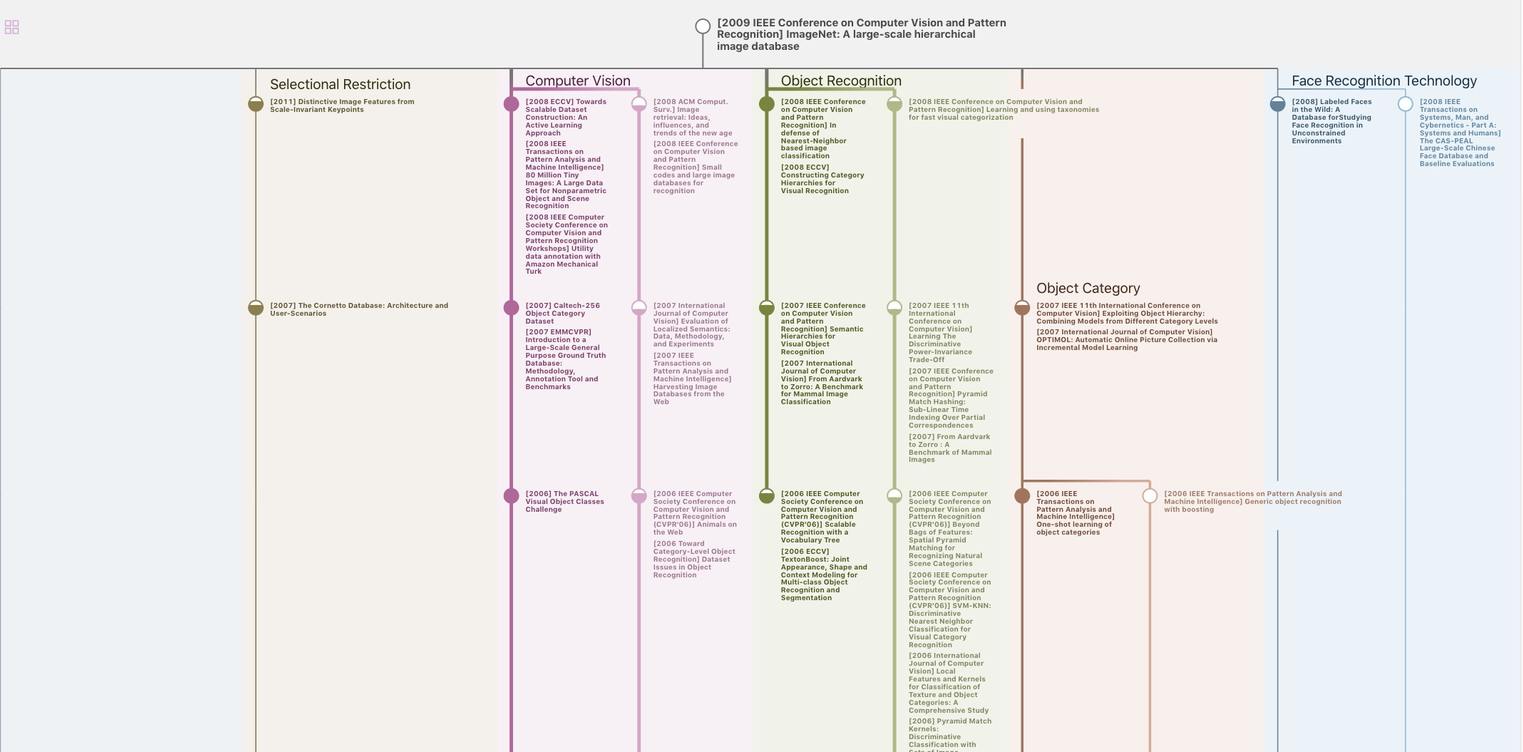
生成溯源树,研究论文发展脉络
Chat Paper
正在生成论文摘要