Noninvasive Isocitrate Dehydrogenase 1 Status Prediction in Grade II/III Glioma Based on Magnetic Resonance Images: A Transfer Learning Strategy.
Journal of computer assisted tomography(2024)
摘要
OBJECTIVE:The aim of this study was to evaluate transfer learning combined with various convolutional neural networks (TL-CNNs) in predicting isocitrate dehydrogenase 1 (IDH1) status of grade II/III gliomas.
METHODS:Grade II/III glioma patients diagnosed at the Tangdu Hospital (August 2009 to May 2017) were retrospectively enrolled, including 54 patients with IDH1 mutant and 56 patients with wild-type IDH1. Convolutional neural networks, AlexNet, GoogLeNet, ResNet, and VGGNet were fine-tuned with T2-weighted imaging (T2WI), fluid attenuation inversion recovery (FLAIR), and contrast-enhanced T1-weighted imaging (T1CE) images. The single-modal networks were integrated with averaged sigmoid probabilities, logistic regression, and support vector machine. FLAIR-T1CE-fusion (FC-fusion), T2WI-T1CE-fusion (TC-fusion), and FLAIR-T2WI-T1CE-fusion (FTC-fusion) were used for fine-tuning TL-CNNs.
RESULTS:IDH1-mutant prediction accuracies using AlexNet, GoogLeNet, ResNet, and VGGNet achieved 70.0% (AUC = 0.660), 65.0% (AUC = 0.600), 70.0% (AUC = 0.700), and 80.0% (AUC = 0.730) for T2WI images, 70.0% (AUC = 0.660), 70.0% (AUC = 0.620), 70.0% (AUC = 0.710), and 80.0% (AUC = 0.720) for FLAIR images, and 73.7% (AUC = 0.744), 73.7% (AUC = 0.656), 73.7% (AUC = 0.633), and 73.7% (AUC = 0.700) for T1CE images, respectively. The highest AUC (0.800) was achieved using VGGNet and FC-fusion images.
CONCLUSIONS:TL-CNNs (especially VGGNet) had a potential predictive value for IDH1-mutant status of grade II/III gliomas.
更多查看译文
AI 理解论文
溯源树
样例
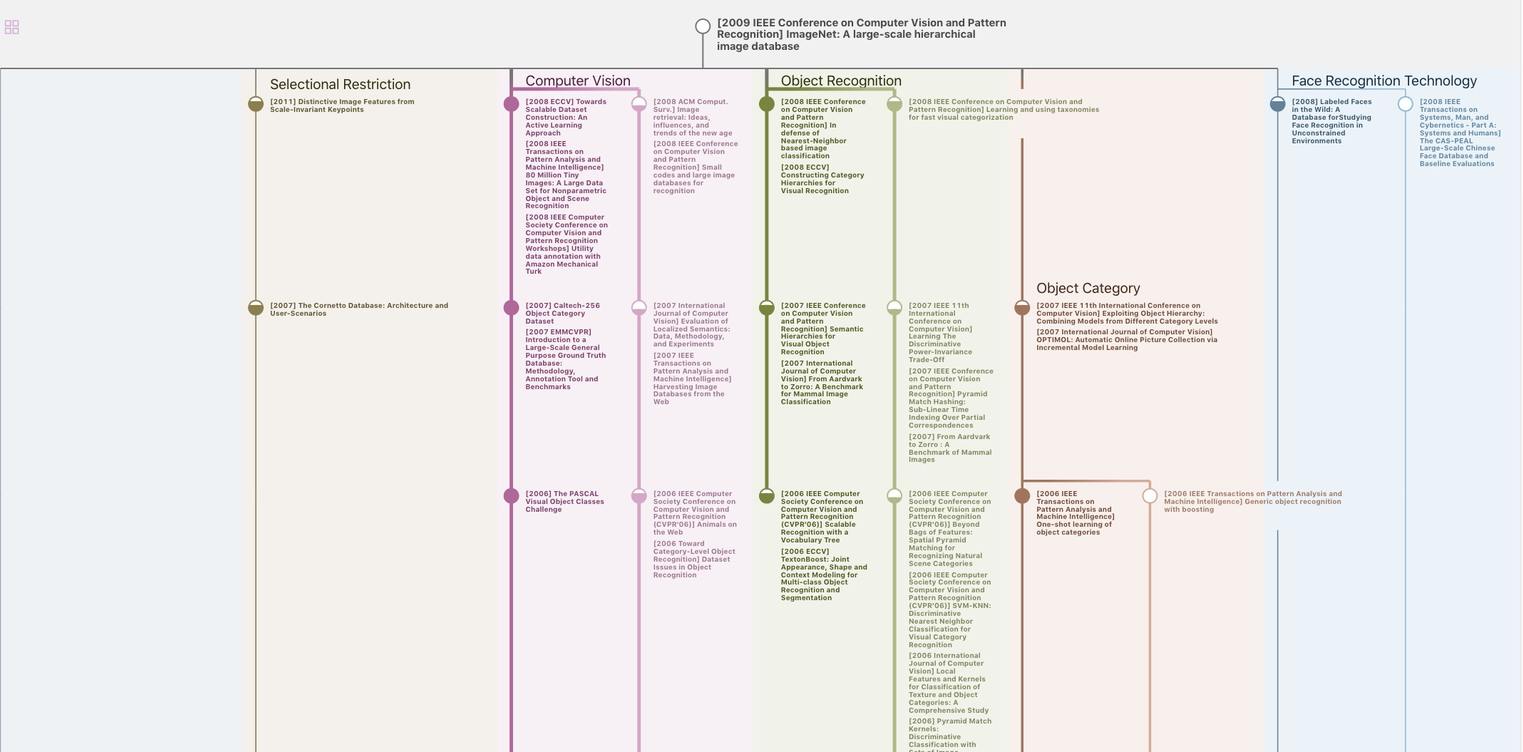
生成溯源树,研究论文发展脉络
Chat Paper
正在生成论文摘要