Monitoring of Mineral Processing Operations with Isolation Forests
MINERALS(2024)
摘要
Reliable monitoring of mineral process systems is key to more efficient plant operation. Multivariate statistical process control based on principal component analysis is well-established in industry but may not be effective when dealing with dynamic nonlinear or transient processes, where process behavior may change rapidly from time to time. Although a large variety of nonlinear models have been proposed to address these problems, the monitoring of complex dynamic process systems remains challenging. Isolation forests are unsupervised machine learning models that provide an interesting approach to process monitoring that has not been explored extensively yet. In this investigation, this approach is compared with traditional multivariate statistical process monitoring based on principal component models. Three real-world case studies are considered. The first case study is based on coal flotation, the second is based on features extracted from a platinum group metal flotation froth; and the third is based on data from an industrial semi-autogenous grinding circuit. In each case, the models were trained on data representing normal operating conditions and then tested on new process data that were generally different from the training data to test their ability to identify these data as out-of-control. The isolation forest models performed better than the principal component models when the data were nonlinear, but not when the data associated with normal operation and faulty conditions were linearly separable, as was the case with the flotation data.
更多查看译文
关键词
mineral processing,flotation froth features,semi-autogenous grinding,isolation forest,multivariate statistical process control,principal component analysis
AI 理解论文
溯源树
样例
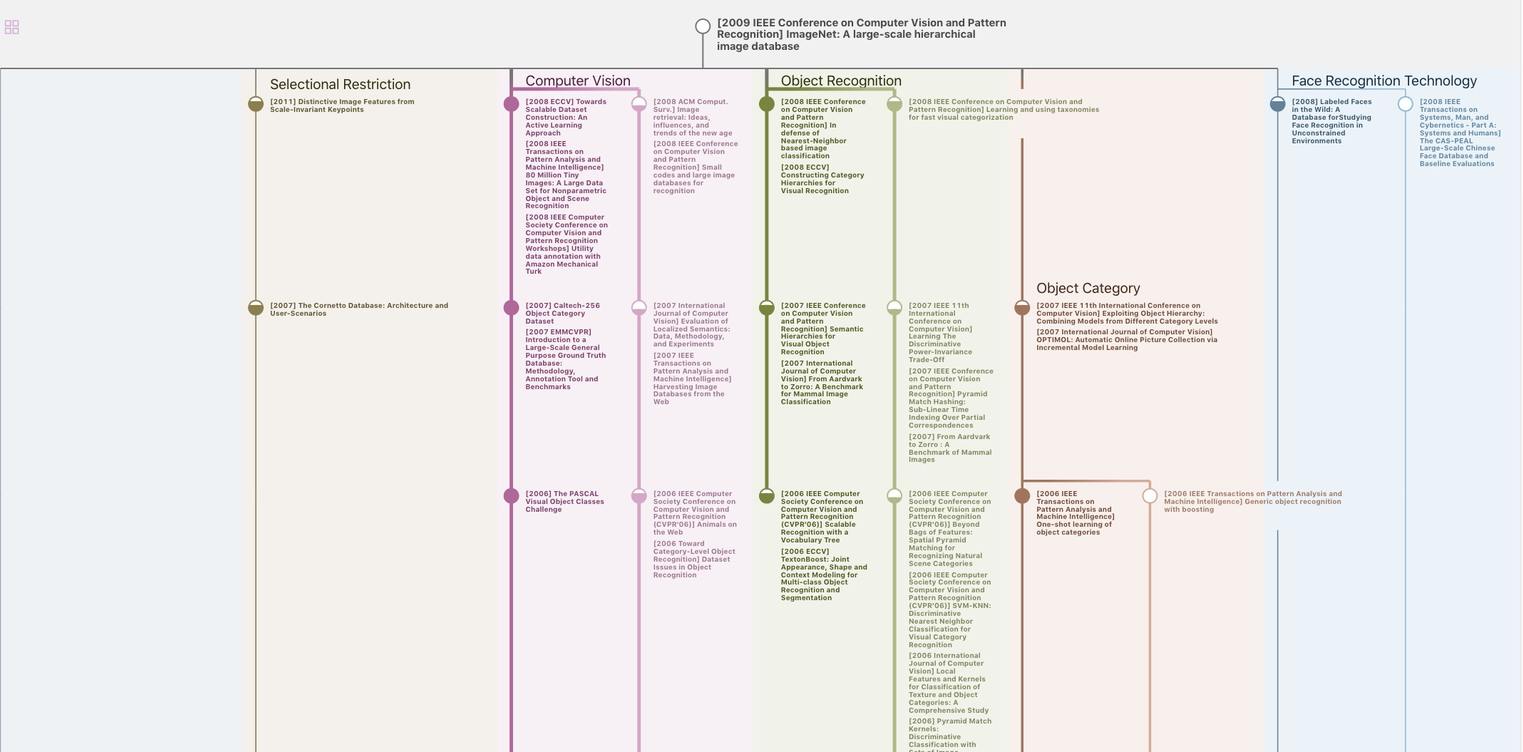
生成溯源树,研究论文发展脉络
Chat Paper
正在生成论文摘要