Reliability and Variability of Ki-67 Digital Image Analysis Methods for Clinical Diagnostics in Breast Cancer
Laboratory Investigation(2024)
摘要
Ki-67 is a nuclear protein associated with proliferation, and a strong potential biomarker in breast cancer, but is not routinely measured in current clinical management due to a lack of standardization. Digital image analysis (DIA) is a promising technology that could allow high throughput analysis and standardization. There is a dearth of data on the clinical reliability as well as intra- and inter-algorithmic variability of different DIA methods. In this study, we scored and compared a set of breast cancer cases in which manually counted Ki-67 has already been demonstrated to have prognostic value (n=278) to five DIA methods; namely, Aperio ePathology, Definiens Tissue Studio, Qupath, an unsupervised IHC color histogram (IHCCH) algorithm and a deep learning pipeline piNET. The piNET system achieved high agreement (ICC: 0.850) and correlation (R= 0.85) with the reference score. The Qupath algorithm exhibited a high degree of reproducibility between all rater instances (ICC: 0.889). Although piNET performed well against absolute manual counts, none of the tested DIA methods classified common Ki-67 cutoffs with high agreement or reached the clinically relevant Cohen’s kappa of at least 0.8. The highest agreement achieved was Cohen’s kappa statistic of 0.73 for cutoffs 20% and 25% by the piNET system. The main contributors to inter-algorithmic variation and poor cutoff characterization included heterogeneous tumor biology, varying algorithm implementation, and setting assignments. It appears that image segmentation is the primary explanation for semi-automated intra-algorithmic variation, which involves significant manual intervention to correct. Automated pipelines such as piNET may be crucial in developing robust and reproducible unbiased DIA approaches to accurately quantify Ki-67 for clinical diagnosis in the future.
更多查看译文
关键词
Breast Cancer,Digital Pathology,Ki-67,Biomarker,Image Analysis
AI 理解论文
溯源树
样例
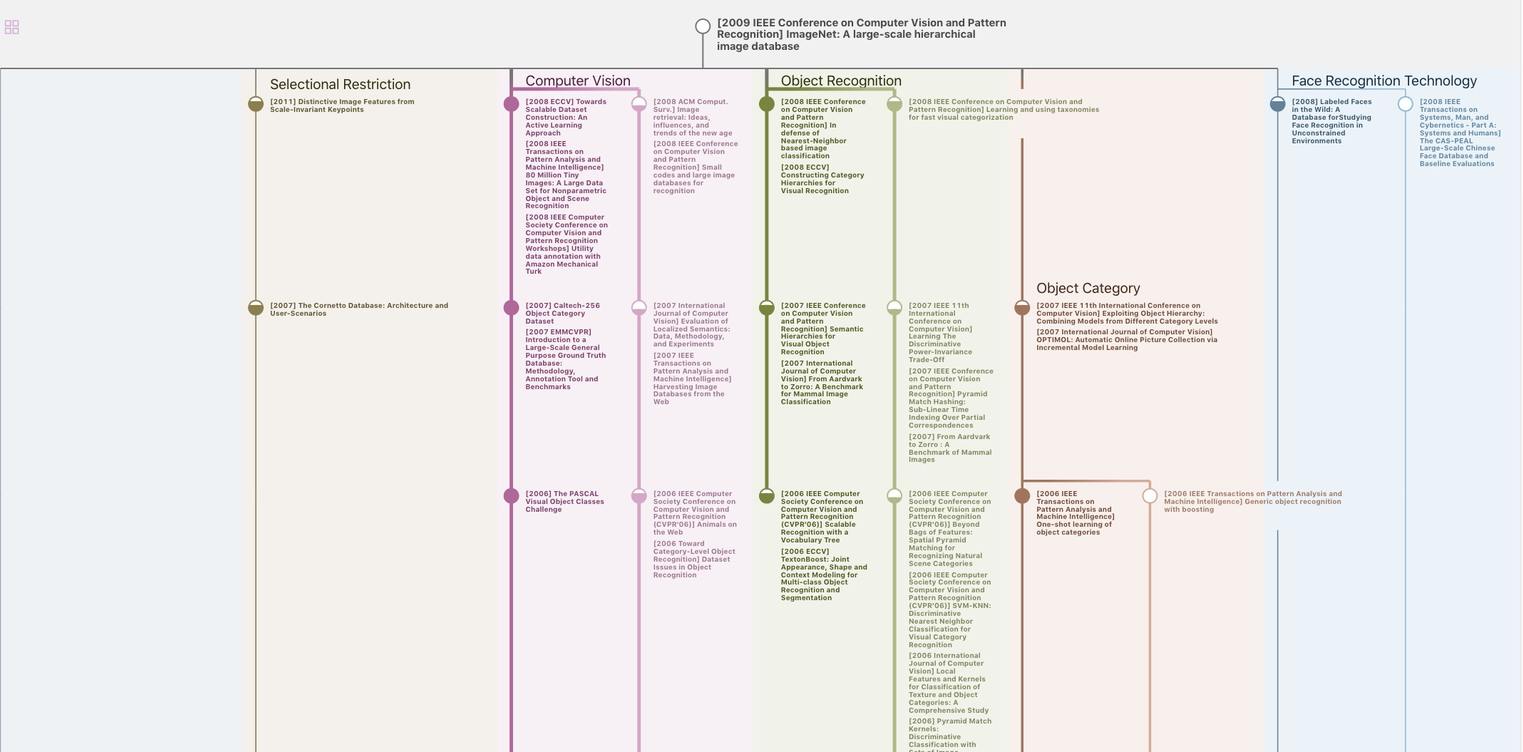
生成溯源树,研究论文发展脉络
Chat Paper
正在生成论文摘要