Rolling theory-guided prediction of hot-rolled plate width based on parameter transfer strategy
ISA Transactions(2024)
摘要
Machine learning performs well in many problems. However, the tendency to generate predictions that violate theoretical knowledge makes it difficult to apply to practical processing. To resolve this situation, this paper combines domain knowledge with a data-driven model, proposes a theory-guided machine learning framework based on a parameter transfer strategy, and applies it to the width prediction of plates after multiple passes of hot rolling. The framework applies a swarm optimization algorithm to the original theoretical model and generates numerous highly-physical consistent samples. The established deep neural network (DNN) model is trained with simulated data, and the parameters are fine-tuned using a parameter transfer strategy combined with actual data to ensure excellent adaptation to the actual environment based on adequate learning of theoretical knowledge. In tests, the proposed model had the best overall prediction performance in this paper. Meanwhile, the developed model is consistent with the existing perception of rolling theory. This allows for the quick and reliable application of machine learning models in production.
更多查看译文
关键词
Hot-rolled plate,Width prediction,Data-driven model,Rolling theory-guided,Parameter transfer
AI 理解论文
溯源树
样例
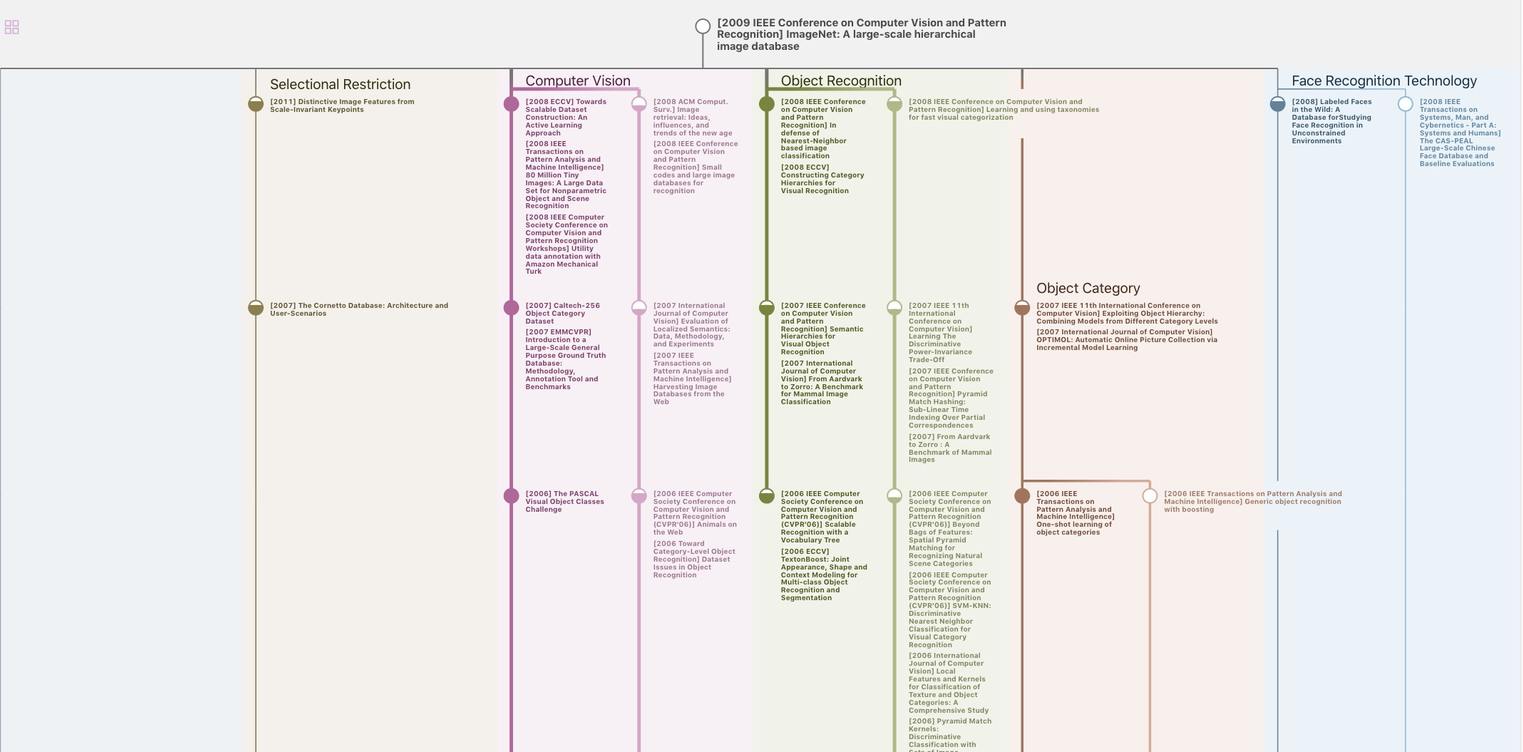
生成溯源树,研究论文发展脉络
Chat Paper
正在生成论文摘要