Deconstructing Denoising Diffusion Models for Self-Supervised Learning
CoRR(2024)
摘要
In this study, we examine the representation learning abilities of Denoising
Diffusion Models (DDM) that were originally purposed for image generation. Our
philosophy is to deconstruct a DDM, gradually transforming it into a classical
Denoising Autoencoder (DAE). This deconstructive procedure allows us to explore
how various components of modern DDMs influence self-supervised representation
learning. We observe that only a very few modern components are critical for
learning good representations, while many others are nonessential. Our study
ultimately arrives at an approach that is highly simplified and to a large
extent resembles a classical DAE. We hope our study will rekindle interest in a
family of classical methods within the realm of modern self-supervised
learning.
更多查看译文
AI 理解论文
溯源树
样例
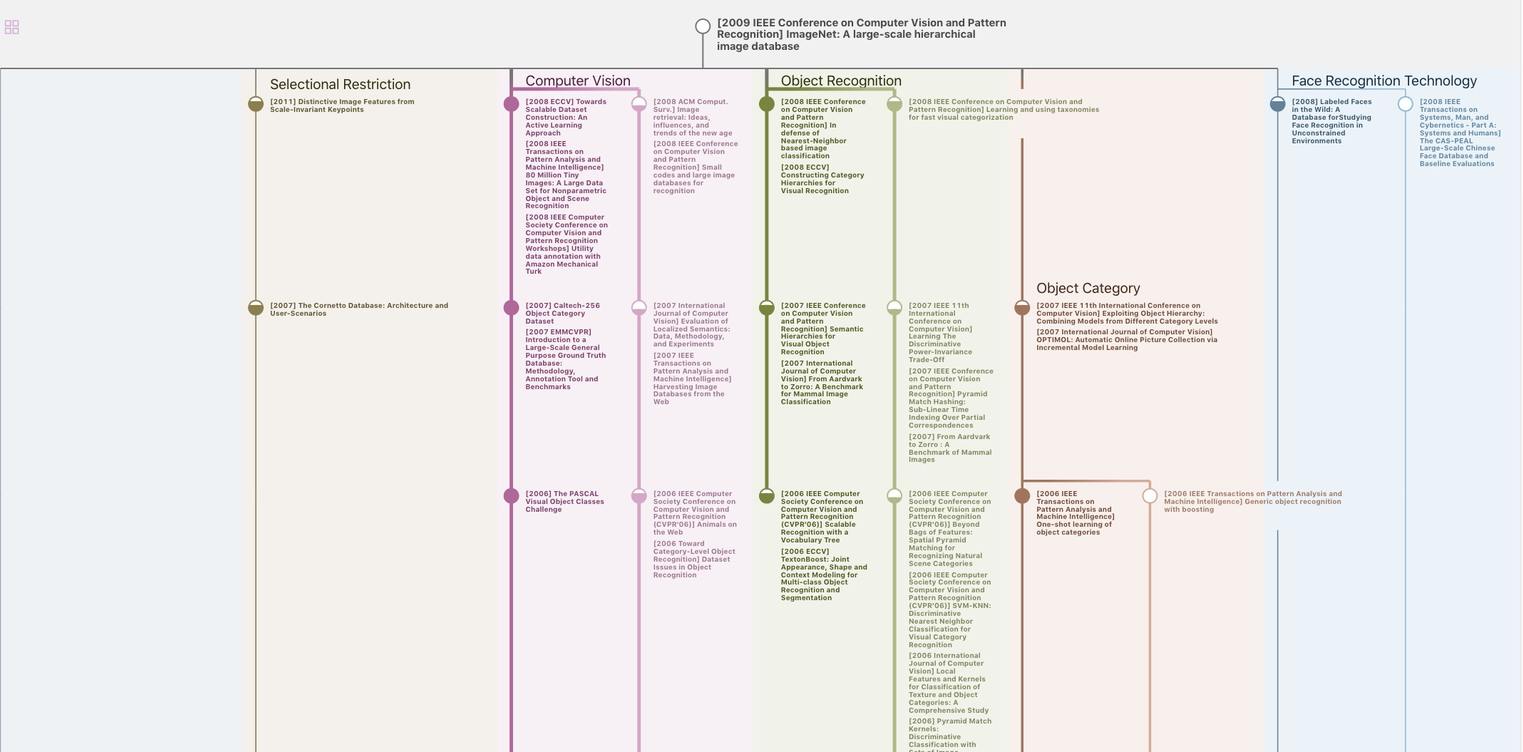
生成溯源树,研究论文发展脉络
Chat Paper
正在生成论文摘要