Dissociable neural signals for reward and emotion prediction errors
biorxiv(2024)
摘要
Reinforcement learning models focus on reward prediction errors (PEs) as the driver of behavior. However, recent evidence indicates that deviations from emotion expectations, termed affective PEs, play a crucial role in shaping behavior. Whether there is neural separability between emotion and reward signals remains unknown. We employ electroencephalography during social learning to investigate the neural signatures of reward and affective PEs. Behavioral results reveal that while affective PEs predict choices when little is known about how a partner will behave, reward PEs become more predictive overtime as uncertainty about a partners behavior diminishes. This functional dissociation is mirrored neurally by engagement of distinct event–related potentials. The FRN indexes reward PEs while the P3b tracks affective PEs. Only the P3b predicts subsequent choices, highlighting the mechanistic influence of affective PEs during social learning. These findings present evidence for a neurobiologically viable emotion learning signal that is distinguishable—behaviorally and neurally—from reward.
### Competing Interest Statement
The authors have declared no competing interest.
更多查看译文
AI 理解论文
溯源树
样例
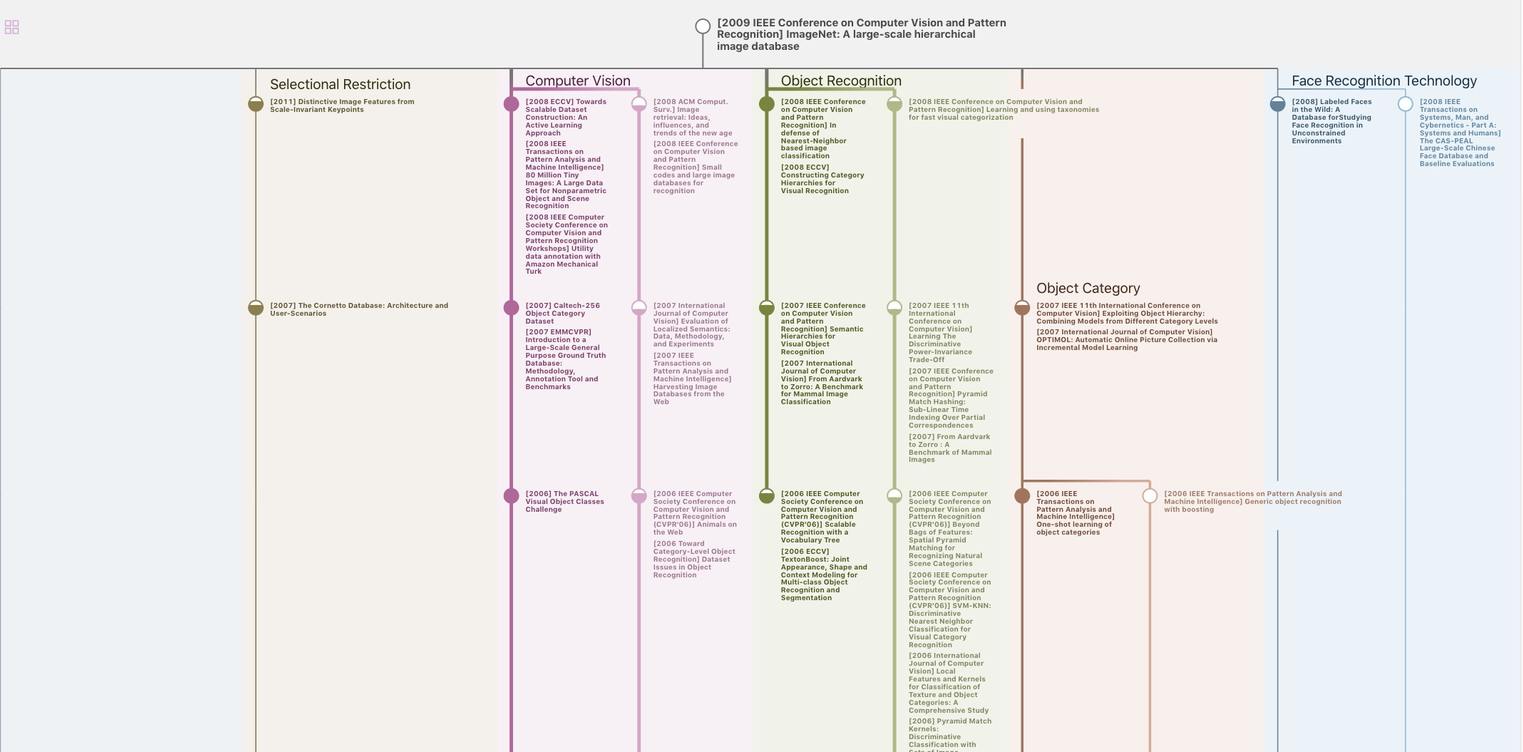
生成溯源树,研究论文发展脉络
Chat Paper
正在生成论文摘要