DBSN:Self-supervised Denoising for OCT Images via Dual Blind Strategy and Blind-Spot Network
2023 IEEE 11th International Conference on Information, Communication and Networks (ICICN)(2023)
摘要
Blind-spot network and its variants have shown promising results in self-supervised denoising tasks. The aim of these methods is to conceal pixels of noisy image and use self-supervised learning to recover them. However, for OCT(optical coherence tomography) images, which have strong auto-correlation between pixels, more effective mask strategies and denoising optimization techniques are needed to improve these methods. To address this issue, this paper proposes a dual mask strategy and blind-spot network (DBSN). Firstly, a fast global mask mapper is designed to break the correlation between pixels in an OCT image. A conditional mask convolution block with centrally masked convolution is embedded inside the blind-spot network. Images after being fed through the global mask mapper and pixel shuffle are then fed into the blind-spot network according to corresponding conditions to achieve two different blind-spot recoveries. Meanwhile, the lower bound of proposed loss function in the case of convergence is discussed, and changes in the weight of the loss function are adapted during training. Finally, a denoising refinement module is used to improve the denoising effect during the inference stage. Numerous experiments demonstrate that DBSN, as a self-supervised denoising approach, outperforms existing methods on OCT data.
更多查看译文
关键词
blind-spot network,self-supervised learning,denoising,optical coherence tomography
AI 理解论文
溯源树
样例
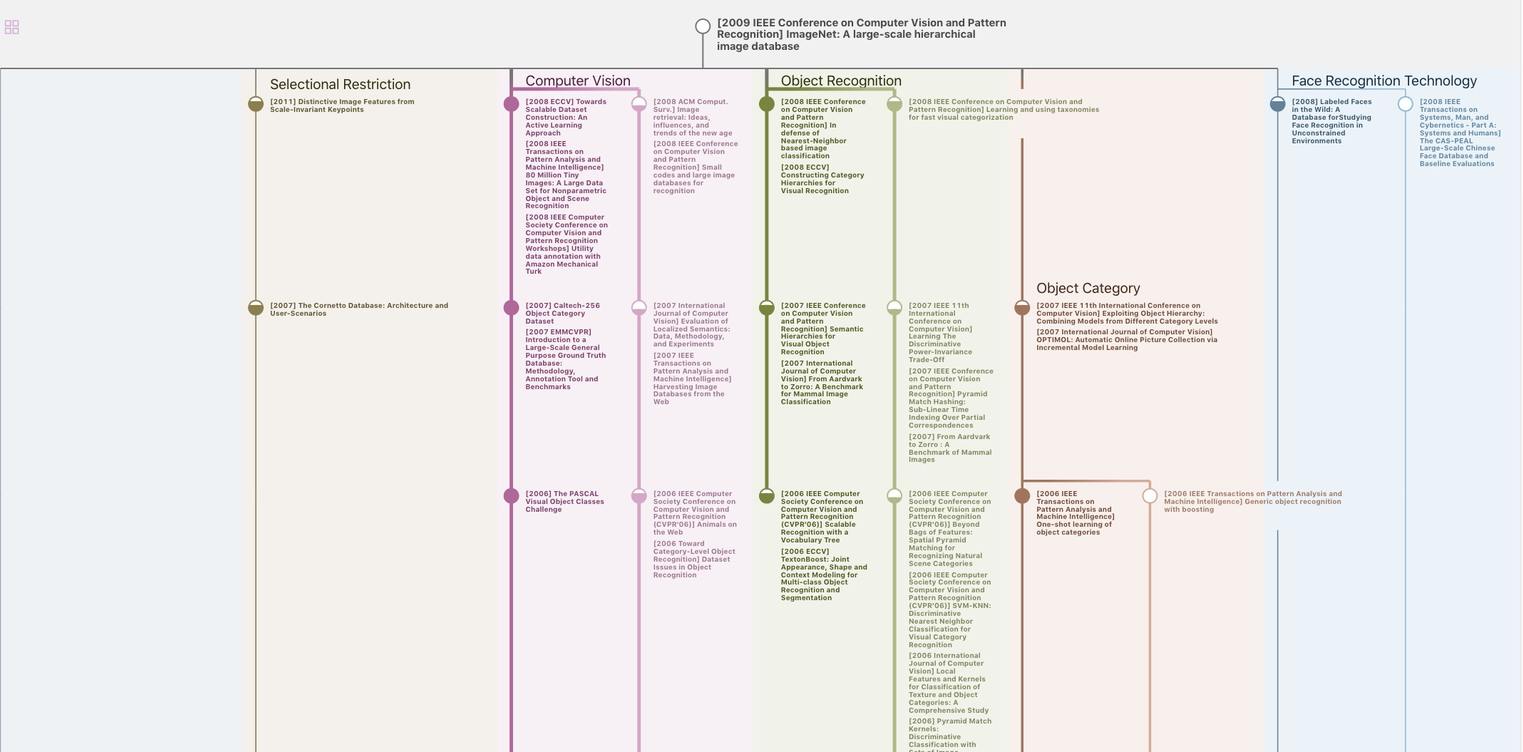
生成溯源树,研究论文发展脉络
Chat Paper
正在生成论文摘要