Near Neighbor Search for Constraint Queries.
2023 IEEE International Conference on Big Data (BigData)(2023)
摘要
There is increasing attention toward search indexes based on dense vector representations as they can encode latent semantic features and can be generated efficiently, thanks to the advances in representation learning. Building an index on vectors was addressed by methods like locality-sensitive hashing (LSH), Inverted File Index (IVF), and near-neighbor graphs and perfected for high performance. However, the current near-neighbor (NN) indexes cannot be directly used in a real recommendation engine where both learned dense features and the constraints-attributes are used. The existing methods are cascaded index with vector search followed by a naive matching or inverted index for filtering on attribute tokens. This filtering on post-vector search matched sets often limits the control on output size and adds latency due to repeated calls to the NN engine. We aim to make a single-stage retrieval model that can retrieve in a single pass and control the output size without compromising on latency. An NN index amenable to this should have a very similar structure to the attribute token-based inverted index. Hence, we develop an efficient constraint search engine based on the high dimensional sparse embeddings of semantic features added with attribute tokens. With this, we get an Inverted index-based Constraint Near Neighbor search-ICONN, where we retrieve search results with 100% match on query attributes and close to its semantic features. We achieve a better latency vs recall10@10 tradeoff compared to the standard NN search followed by attribute filtering.
更多查看译文
关键词
Constraint near neighbor,sparse embedding index,hashing
AI 理解论文
溯源树
样例
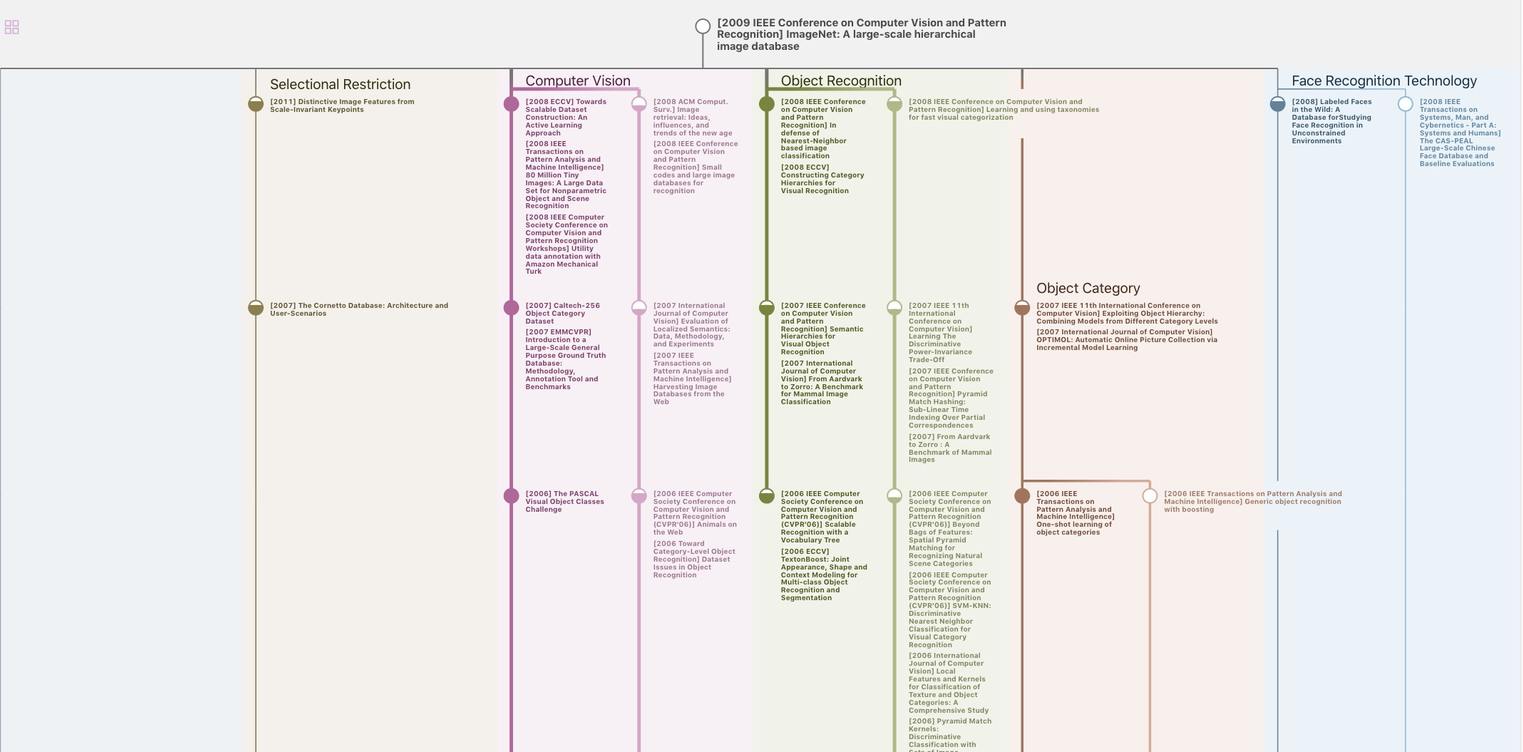
生成溯源树,研究论文发展脉络
Chat Paper
正在生成论文摘要