Enabling Dataspaces Using Foundation Models: Technical, Legal and Ethical Considerations and Future Trends.
2023 IEEE International Conference on Big Data (BigData)(2023)
摘要
Foundation Models are pivotal in advancing artificial intelligence, driving notable progress across diverse areas. When merged with dataspace, these models enhance our capability to develop algorithms that are powerful, predictive, and honor data sovereignty and quality. This paper highlights the potential benefits of a comprehensive repository of Foundation Models, contextualized within dataspace. Such an archive can streamline research, development, and education by offering a comparative analysis of various models and their applications. While serving as a consistent reference point for model assessment and fostering collaborative learning, the repository does face challenges like unbiased evaluations, data privacy, and comprehensive information delivery. The paper also notes the importance of the repository being globally applicable, ethically constructed, and user-friendly. We delve into the nuances of integrating Foundation Models within dataspace, balancing the repository’s strengths against its limitations.
更多查看译文
关键词
dataspace,linked,machine learning,foundation model
AI 理解论文
溯源树
样例
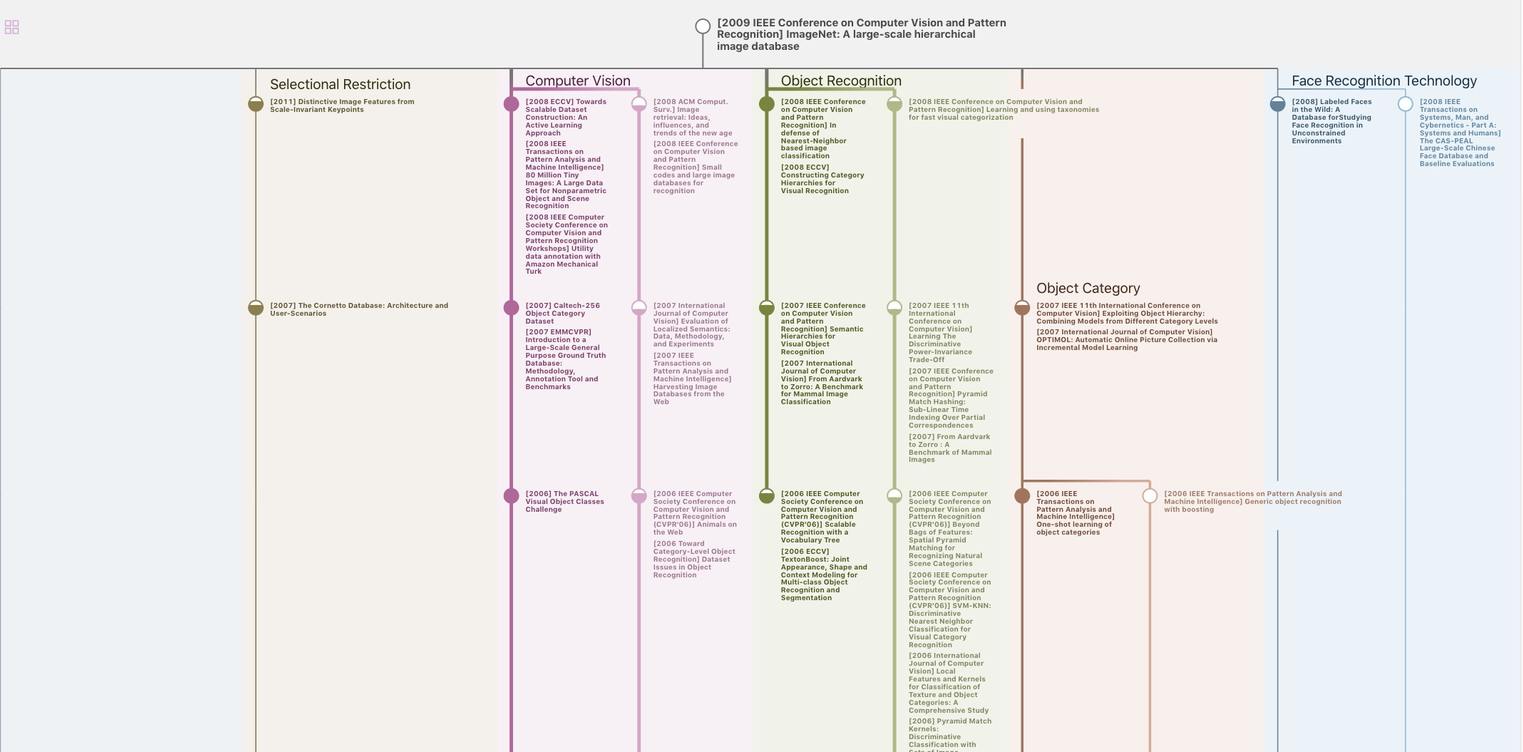
生成溯源树,研究论文发展脉络
Chat Paper
正在生成论文摘要