Enhancing Scientific Image Classification through Multimodal Learning: Insights from Chest X-Ray and Atomic Force Microscopy Datasets.
2023 IEEE International Conference on Big Data (BigData)(2023)
摘要
In this study, we conduct a detailed evaluation of machine learning and multimodal learning approaches in two distinct areas: a standard medical imaging benchmark and a novel material sciences benchmark. We utilize the CheXpert chest x-ray dataset for medical imaging and introduce a newly created Fluoropolymer Atomic Force Microscopy (AFM) dataset for material sciences. Both datasets are enhanced with additional images and binary metadata, encoded as one-hot vectors. We tested both pretrained and non-pretrained Convolutional Neural Network (CNN) models, such as ResNet50, ResNet101, DenseNet121, InceptionV3, and Xception, across different combinations of image and metadata inputs. Our results reveal that integrating multimodal data, including simple binary metadata, significantly enhances classification accuracy compared to conventional unimodal approaches or advanced MADDi models. This indicates the efficacy of multimodal learning in enriching data representation and boosting image classification performance. Notably, Xception models showed exceptional performance in CheXpert tests, and most models improved crystal structure predictions in AFM datasets. These insights set a new benchmark for performance and underscore the potential of multimodal learning in data-intensive applied science research.
更多查看译文
关键词
Machine Learning,Multimodal Learning,Image Classification,Atomic Force Microscopy (AFM),CheXpert Dataset,Material Science
AI 理解论文
溯源树
样例
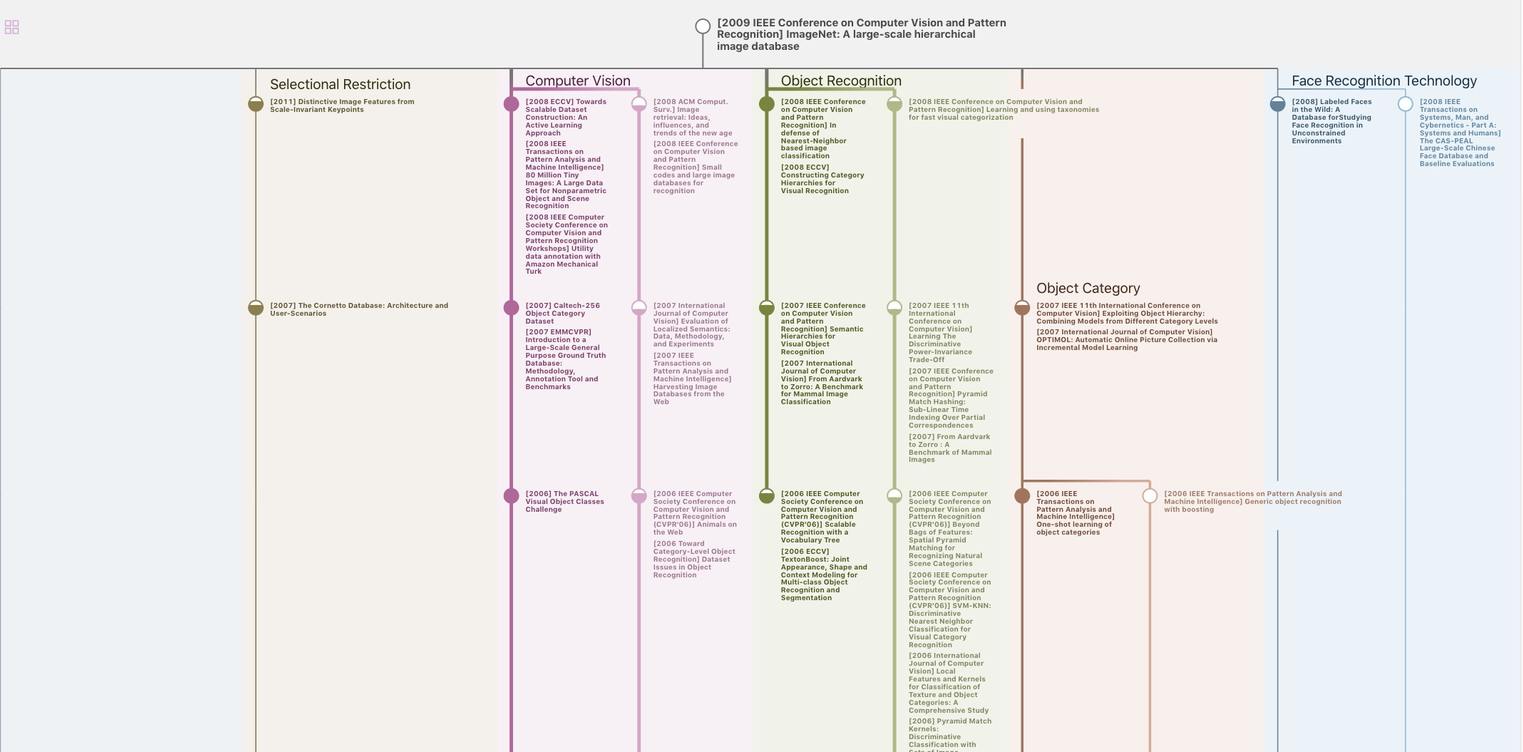
生成溯源树,研究论文发展脉络
Chat Paper
正在生成论文摘要