Dual-track spatio-temporal learning for urban flow prediction with adaptive normalization
Artificial Intelligence(2024)
摘要
Robust urban flow prediction is crucial for transportation planning and management in urban areas. Although recent advances in modeling spatio-temporal correlations have shown potential, most models fail to adequately consider the complex spatio-temporal semantic information present in real-world scenarios. We summarize the following three primary limitations in existing models: a) The majority of existing models project overall time periods into the same latent space, neglecting the diverse temporal semantics between different time intervals. b) Existing models tend to capture spatial dependencies from a locale perspective such as surroundings but do not pay attention to the global influence factors. c) Beyond the spatio-temporal properties, the dynamics and instability of the data sequences introduce perturbations to the prediction results, potentially leading to model degradation. To address these issues, we propose a dual-track spatial-temporal learning module named DualST for accurate urban flow inference. To more effectively differentiate semantic information in the time dimension, we assign the overall time scales into closeness and periodicity. The dual-track module, which includes temporal causality inference and temporal contextual inference, simultaneously exploits the dynamic evolutionary trends and periodic traffic patterns, respectively. The proposed DualST captures global spatial features in a self-supervised manner which not only enriches the spatial semantics but also avoids introducing additional prior knowledge. To eliminate the instability caused by dynamics, we first adopt spatio-temporal adaptive normalization to learn appropriate data sequence normalization. We evaluate the proposed DualST on two typical urban flow datasets. The experiment results show that our model not only exhibits a consistent superiority over various state-of-the-art baselines but also has remarkable generalization capability.
更多查看译文
关键词
Urban flow prediction,Spatio-temporal learning,Spatio-temporal normalization,Contrastive learning,Regional and global correlations
AI 理解论文
溯源树
样例
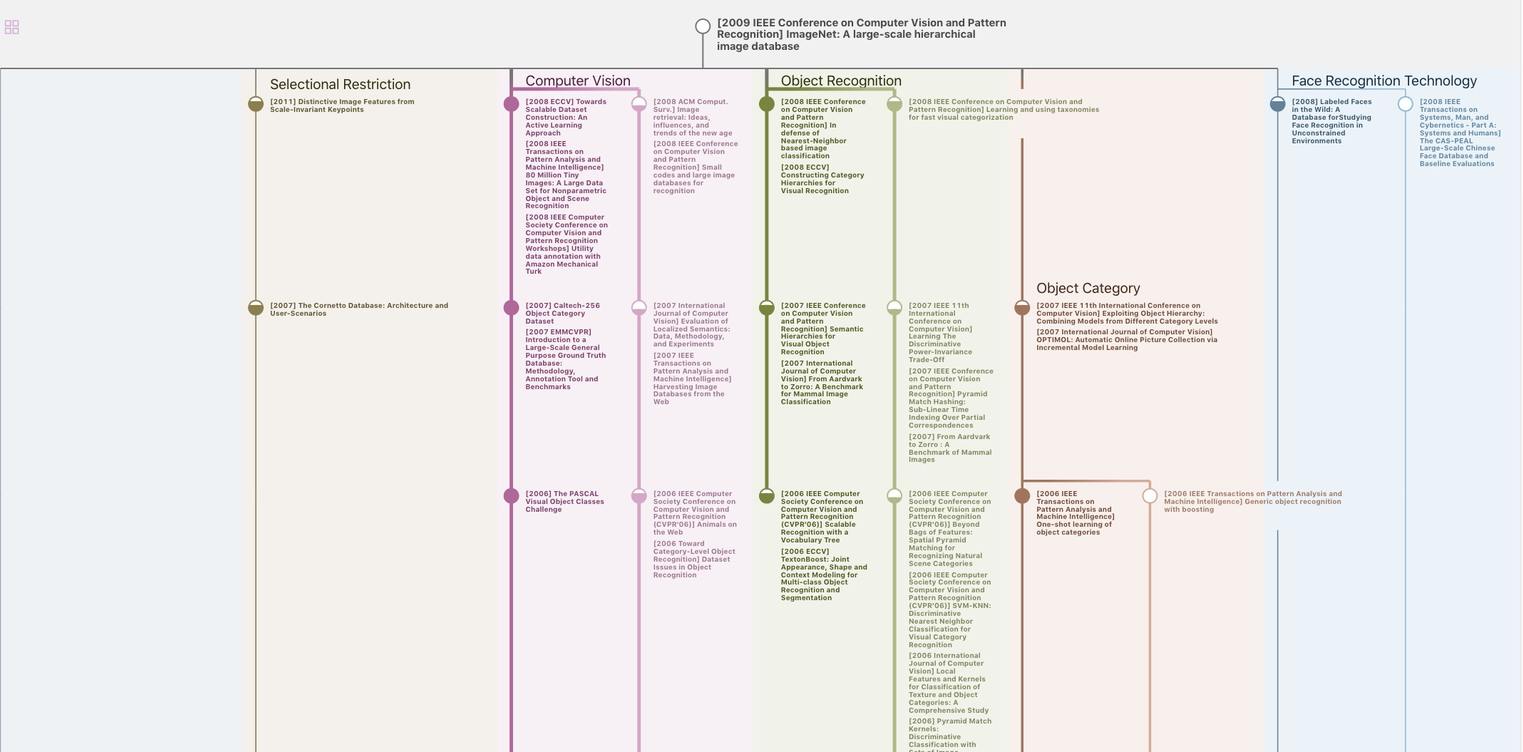
生成溯源树,研究论文发展脉络
Chat Paper
正在生成论文摘要