Type-LDD: A Type-Driven Lite Concept Drift Detector for Data Streams
IEEE Transactions on Knowledge and Data Engineering(2023)
摘要
Concept drift is a phenomenon that the distribution of data streams changes with time. When this happens, model predictions become less accurate. Hence, concept drift needs to be detected and adapted. Existing drift detection methods are good at determining when drift has occurred, but few retrieve information about how the drift came to be present in the stream, i.e., what type of drift has occurred. Hence, discussing the impact of the type of drift on adaptation is a difficult thing. To fill this gap, we propose a pre-trained framework for training a drift detector called a type-driven lite concept drift detector (Type-LDD) that retrieves information about both when and how a drift has occurred. In our proposed pre-trained framework, the Type-LDD including a drift-type identifier and a drift-point locator was based on a synthetic dataset containing a range of drift types. When repurposing the pre-trained model for detecting new data streams, a knowledge distillation module fine-tunes the proposed Type-LDD to speed up inference and keep detection accuracy. The proposed Type-LDD is validated on both synthetic data and real-world data, and demonstrated that accurately identifying the type of drift that has occurred can improve adaptation accuracy.
更多查看译文
关键词
data streams,concept drift,prototypical network,knowledge distillation
AI 理解论文
溯源树
样例
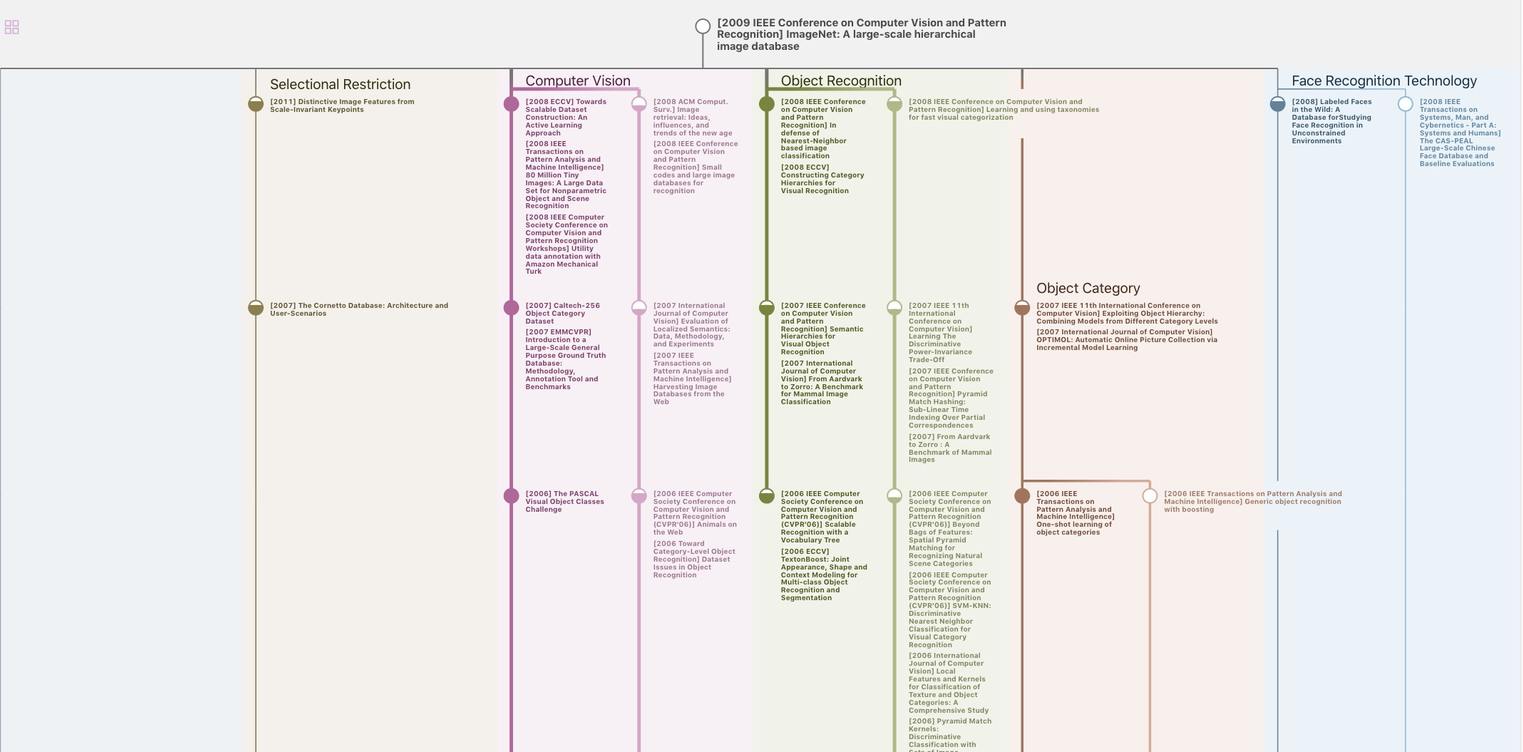
生成溯源树,研究论文发展脉络
Chat Paper
正在生成论文摘要