QALL: Distributed Queue-Behavior-Aware Load Balancing Using Programmable Data Planes
IEEE Transactions on Network and Service Management(2023)
摘要
Existing load-balancing methods used in data center networks involve some shortcomings such as excessively large decision delays during reactions to microbursts and large overheads involved in active probing. Programmable data planes have provided new opportunities for local decision-making on switches to address these issues. We observe that queue behavior (i.e., queue occupancy, queuing trend, and dequeue time interval) in switches can reflect the current or future congestion degree on a network. Furthermore, following data-driven experiments, we found an accurate fitting function of congestion degree to queue behavior. Thus, we propose an in-network load-balancing scheme based on a programmable switch, called queue-behavior-aware localized load balancing (QALL). In QALL, each switch independently selects egress ports probabilistically according to fine-grained-measured local queue behavior. The key concept of QALL is to take account the evolutionary process of reaching the current queue state into its decision basis for load balancing. Experimental results under actual DCN workloads (including web search and data mining workloads) demonstrate the effectiveness of QALL. In terms of flow completion time, decision delay, network shock, load sharing accuracy, and packet reordering, QALL outperformed recent perpacket (DRILL), per-flowlet (LetFlow and CONGA), and per-flow (ECMP) load balancers, particularly under heavy load. For example, under asymmetrical topology with 90% load level, the flow completion time of QALL was lower than that of ECMP, LetFlow, CONGA, and DRILL by up to 54.7%, 46.5%, 38.9%, and 18.9%, respectively.
更多查看译文
关键词
Data center networks,Distributed,Load balancing,Programmable Data Plane,Queue behavior
AI 理解论文
溯源树
样例
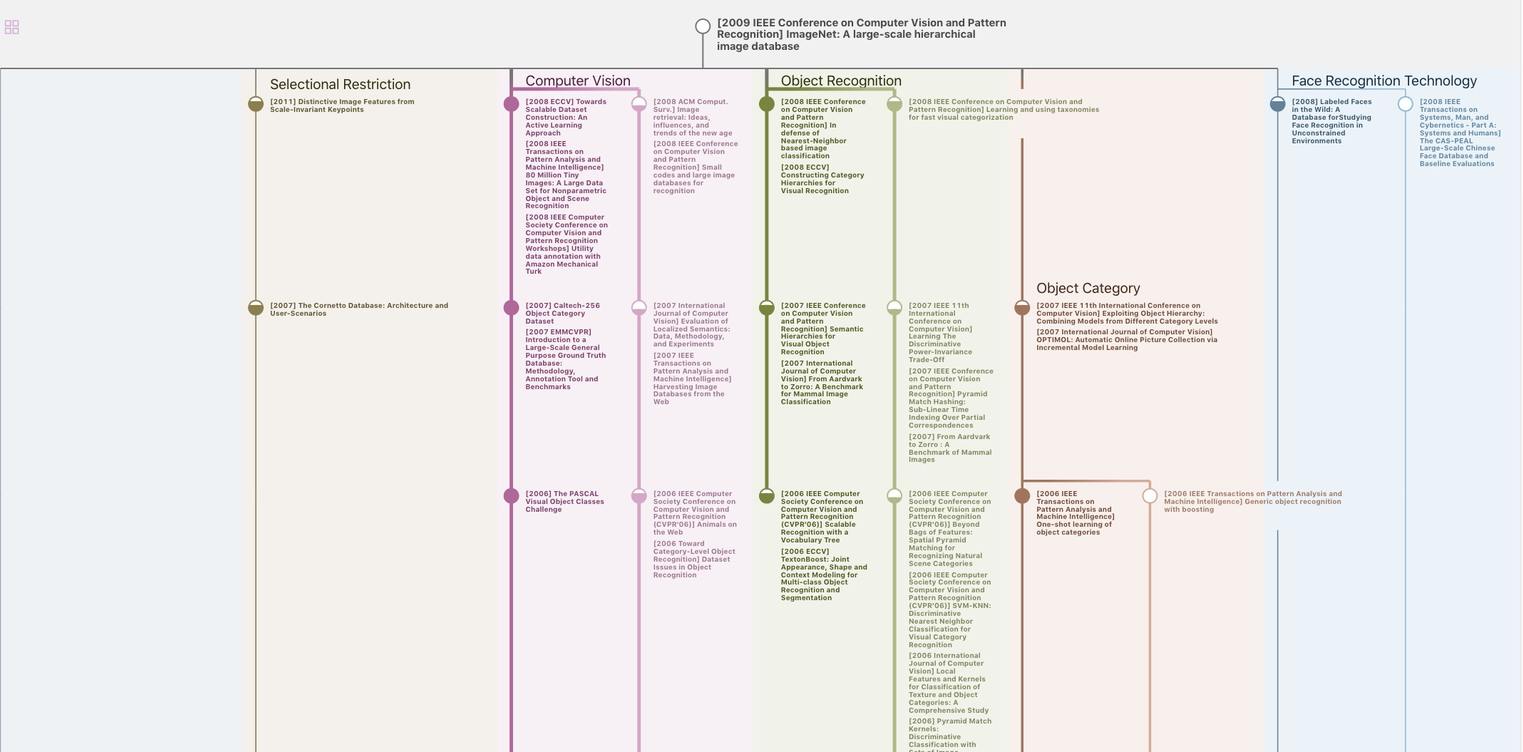
生成溯源树,研究论文发展脉络
Chat Paper
正在生成论文摘要