Nonstationary Prestack Linear Bayesian Stochastic Inversion.
IEEE Transactions on Geoscience and Remote Sensing(2024)
摘要
Bayesian inversion is capable of integrating seismic data, well-log data, and geological data to obtain a posterior probability distribution function (PPDF) of elastic parameters. Due to the absorption of strata, amplitude attenuation and phase distortion inevitably occur during the propagation of seismic wave, resulting in low resolution of seismic data. The traditional linear Bayesian inversion method is based on the stationary convolution model, amplitude compensation and phase correction are needed to be conducted in advance. Uncertainties exist in both compensation and inversion, and the inversion results cannot account for the uncertainties in the directly observed seismic data resulting in accurate inversion results. To estimate uncertainty more accurately and improve inversion accuracy, in this paper, we develop a non-stationary pre-stack linear Bayesian stochastic inversion (NSPLBSI) method. Through the proposed method, prior information from well-log data can be effectively introduced to constrain inversion. Compensation and inversion are integrated into one procedure, which can estimate the posterior uncertainties more accurately directly from seismic data compared with traditional two-step inversion methods, i.e., firstly compensating attenuation and secondly performing inversion. Also, more accurate inversion results are obtained. In order to verify the rationality and effectiveness of our proposed method, we conduct inversions using synthetic seismic data on the Marmousi model and a field seismic dataset. Numerical examples show that it cannot only compensate amplitude well, but also obtain high-precision inversion results with smaller prediction interval.
更多查看译文
关键词
Bayesian inversion,posterior probability distribution function,non-stationary,stochastic inversion,prediction interval
AI 理解论文
溯源树
样例
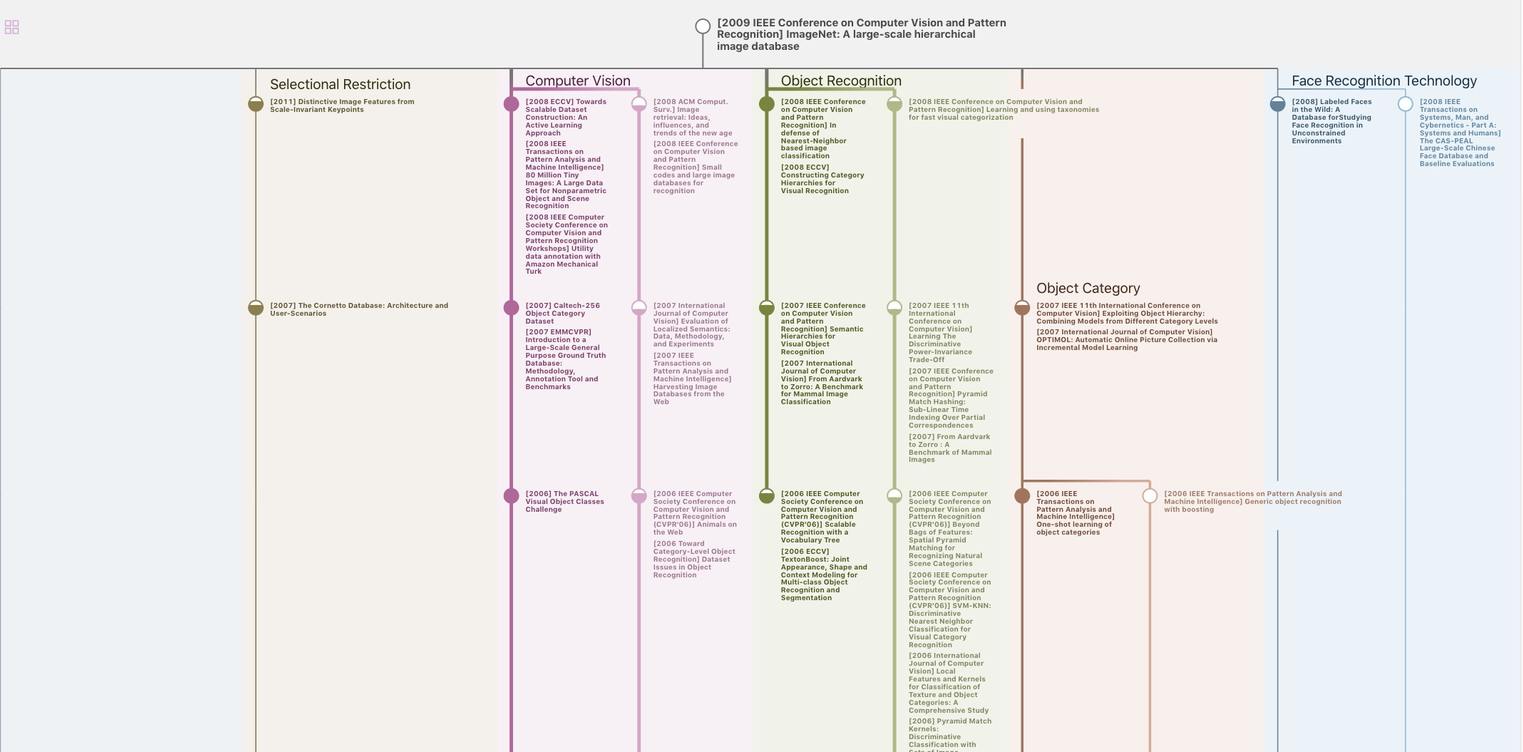
生成溯源树,研究论文发展脉络
Chat Paper
正在生成论文摘要