Elastic Task Offloading and Resource Allocation Over Hybrid Cloud: A Reinforcement Learning Approach.
IEEE Trans. Netw. Serv. Manag.(2024)
摘要
Hybrid cloud is an emerging computing cloud solution that leverages the power of the public cloud, without abandoning the computation resources of existing on-premises data-centers. Further, the wide adoption of cloud-native technology, like containers, brings the capability of rapid horizontal and vertical scaling to task workloads. However, the heterogeneity and flexibility can bring more complexity to task processing performance optimization, especially with constrained on-premises energy consumption and public cloud renting cost quota. In this paper, we seek to optimize the task processing performance under long-term on-premises energy consumption and public cloud renting cost constraints via dynamic task offloading and elastic scaling. We formulate the problem as a two-stage mixed integer non-linear programming (MINLP) problem, and propose an online approach named ETHC (elastic task offloading and resource allocation handler over hybrid cloud). For the first stage, we introduce a Lyapunov optimization-assisted Deep Reinforcement Learning (DRL) agent to decompose the long-term optimization problem into per-time-segment sub-problems on making task offloading decisions. In the second stage, based on the M/M/k queuing model, we prove the container instance number configuration and per-instance resource allocation problem as a convex MINLP problem. An efficient bi-section-based algorithm is introduced to obtain the optimal configurations. Extensive simulations show that ETHC manages to stabilize the task processing queue and satisfy the long-term constraints under various environments and parameters setup, with slight overhead on the convergence speed. Besides, optimal resource configuration and instance number can be obtained at each time-segment with low time complexity.
更多查看译文
关键词
Hybrid Cloud,Task Offloading,Deep Reinforcement Learning,Lyapunov Optimization
AI 理解论文
溯源树
样例
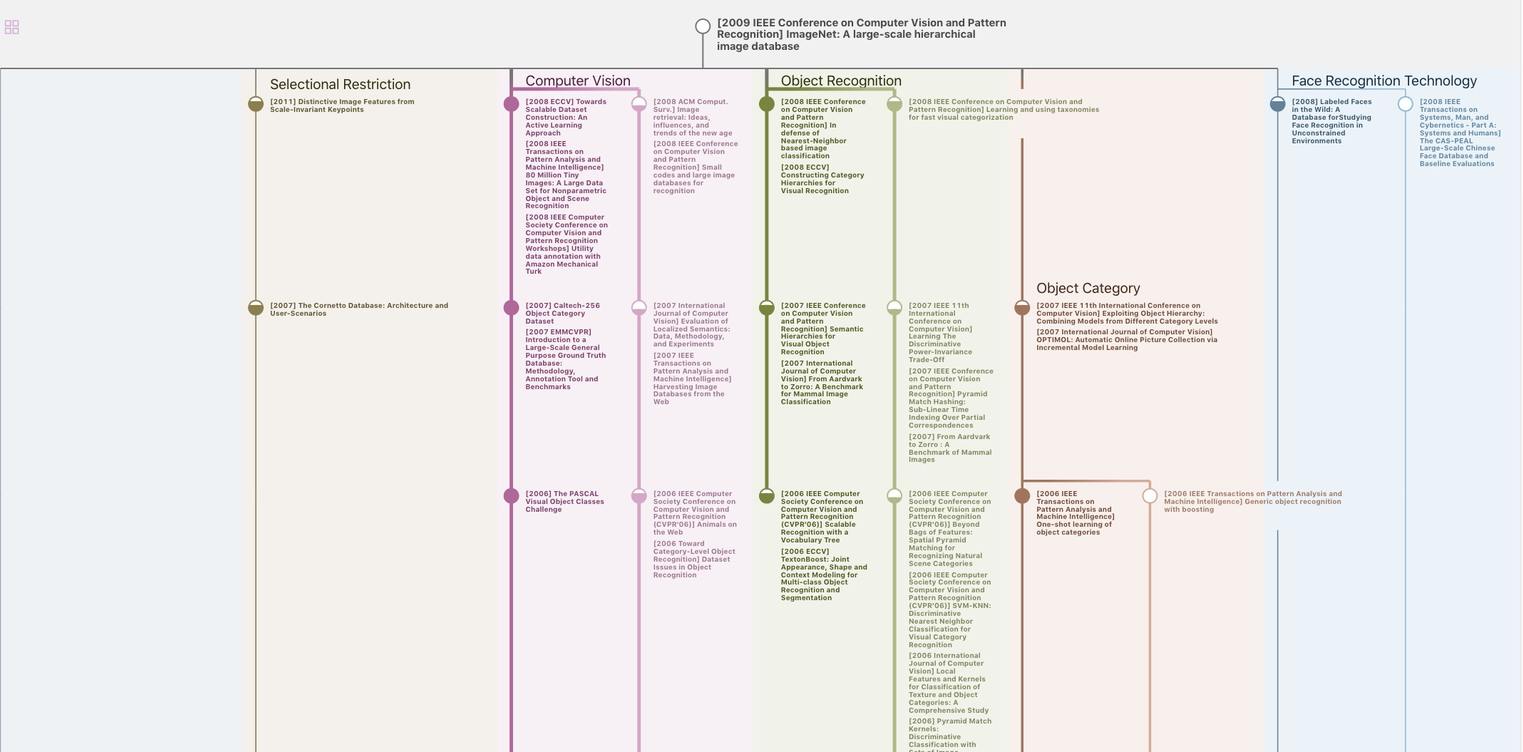
生成溯源树,研究论文发展脉络
Chat Paper
正在生成论文摘要