Point Transformer-Based Salient Object Detection Network for 3-D Measurement Point Clouds.
IEEE Transactions on Geoscience and Remote Sensing(2024)
摘要
While salient object detection (SOD) on 2D images has been extensively studied, there is very little SOD work on 3D measurement surfaces. We propose an effective point transformer-based SOD network for 3D measurement point clouds, termed PSOD-Net. PSOD-Net is an encoder-decoder network that takes full advantage of transformers to model the contextual information in both multi-scale point- and scene-wise manners. In the encoder, we develop a Point Context Transformer (PCT) module to capture region contextual features at the point level; PCT contains two different transformers to excavate the relationship among points. In the decoder, we develop a Scene Context Transformer (SCT) module to learn context representations at the scene level; SCT contains both Upsampling-and-Transformer blocks and Multi-context Aggregation units to integrate the global semantic and multi-level features from the encoder into the global scene context. Experiments show clear improvements of PSOD-Net over its competitors and validate that PSOD-Net is more robust to challenging cases such as small objects, multiple objects, and objects with complex structures. Code is available at: https://github.com/ZeyongWei/PSOD-Net.
更多查看译文
关键词
PSOD-Net,3D salient object detection,point transformer,3D measurement point cloud
AI 理解论文
溯源树
样例
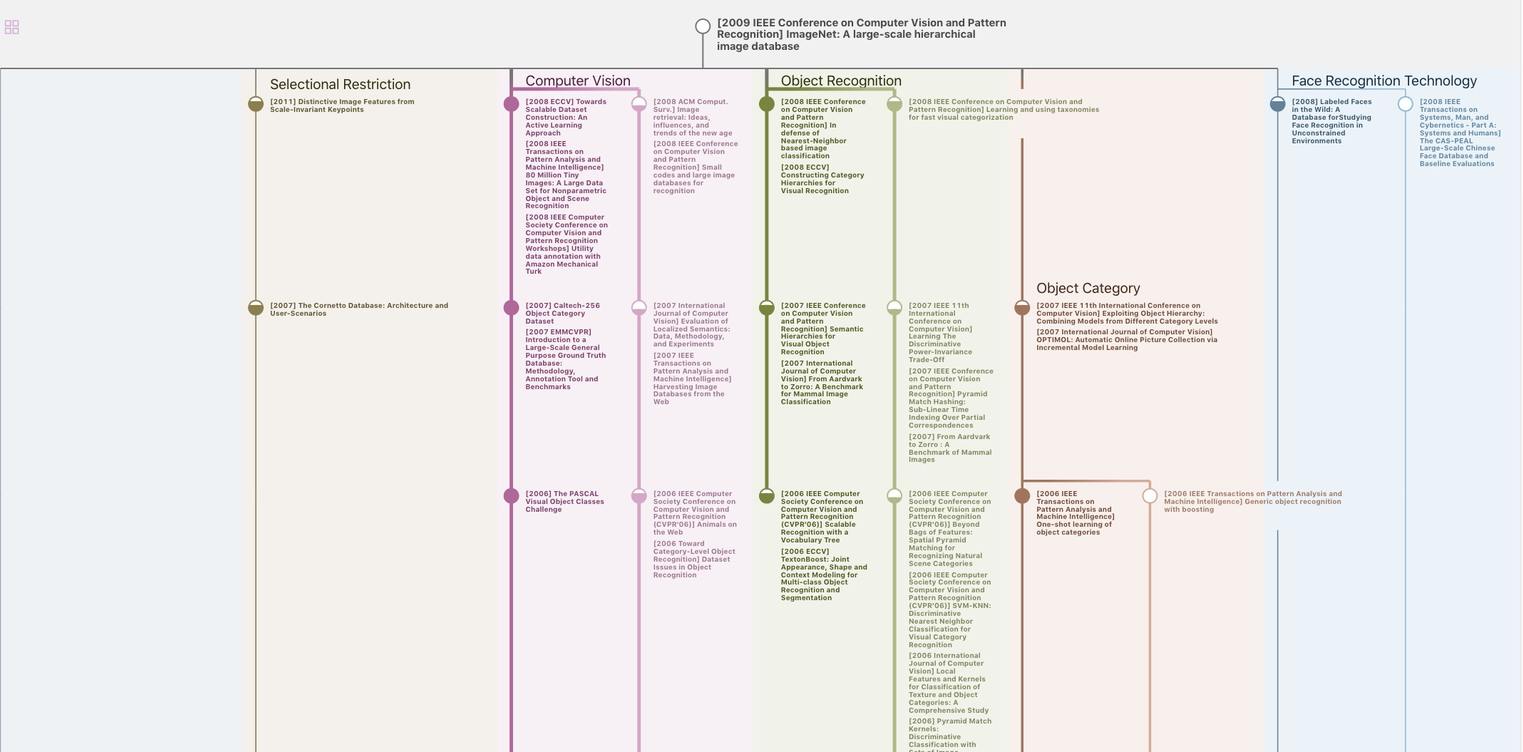
生成溯源树,研究论文发展脉络
Chat Paper
正在生成论文摘要