General-Purpose Sim2Real Protocol for Learning Contact-Rich Manipulation With Marker-Based Visuotactile Sensors.
IEEE Trans. Robotics(2024)
摘要
Visuotactile sensors can provide rich contact information, having great potential in contact-rich manipulation tasks with reinforcement learning (RL) policies. Sim2Real technique tackles the challenge of RL's reliance on a large amount of interaction data. However, most Sim2Real methods for manipulation tasks with visuotactile sensors rely on rigid-body physics simulation, which fails to simulate the real elastic deformation precisely. Moreover, these methods do not exploit the characteristic of tactile signals for designing the network architecture. In this paper, we build a general-purpose Sim2Real protocol for manipulation policy learning with marker-based visuotactile sensors. To improve the simulation fidelity, we employ an FEM-based physics simulator that can simulate the sensor deformation accurately and stably for arbitrary geometries. We further propose a novel tactile feature extraction network that directly processes the set of pixel coordinates of tactile sensor markers and a self-supervised pre-training strategy to improve the efficiency and generalizability of RL policies. We conduct extensive Sim2Real experiments on the peg-in-hole task to validate the effectiveness of our method. And we further show its generalizability on additional tasks including plug adjustment and lock opening. The protocol, including the simulator and the policy learning framework, will be open-sourced for community usage.
更多查看译文
关键词
Tactile sensing,robot simulation,sim-to-real,contact-rich manipulation
AI 理解论文
溯源树
样例
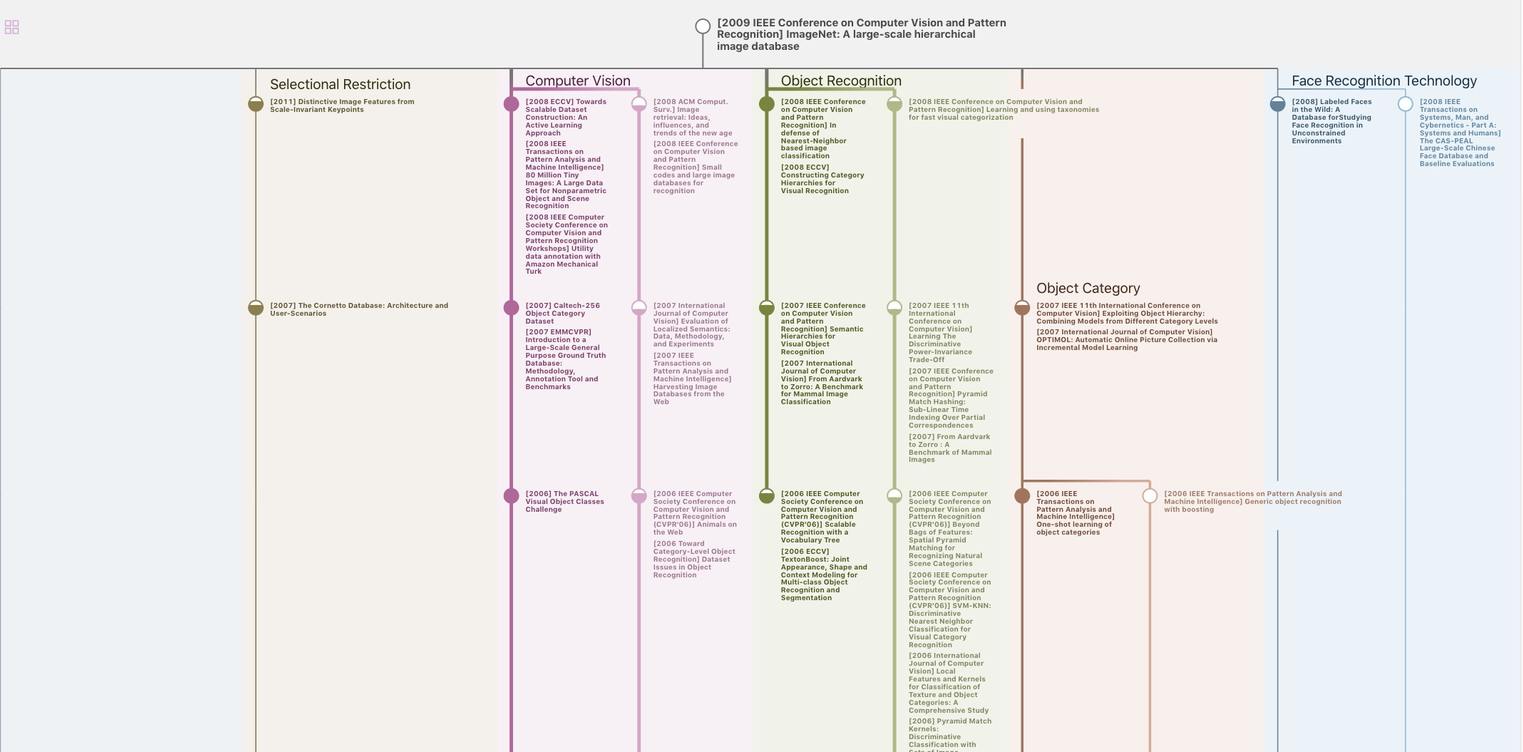
生成溯源树,研究论文发展脉络
Chat Paper
正在生成论文摘要