Adversarial Mixture Density Network and Uncertainty-based Joint Learning for 360$^{\circ }$ Monocular Depth Estimation
IEEE Transactions on Multimedia(2023)
摘要
Due to the increased demand of 360
$^{\circ }$
images (
e.g.
virtual reality), estimating 360
$^{\circ }$
depths via deep learning has drawn attention recently. However, all previous studies share the same fundamental limitation: a lack of data. To address the issue of data insufficiency, self-supervised learning and uncertainty-aware learning based on a mixture density network (MDN) have been actively studied and have achieved great success on various tasks. Unfortunately, under the harsh training environment of 360
$^{\circ }$
depth estimation tasks (
e.g.
a large field-of-view, distortion), we observe that the practical difficulties of self-supervised learning and MDN-based uncertainty-aware learning become a critical factor degrading the depth results. In this paper, we propose an
adversarial mixture density network (AMDN)
and
uncertainty-based joint learning
to improve the depth qualities by addressing the data insufficiency issue properly. For the AMDN, architectures and objective functions of the MDN are redesigned in an adversarial manner. For uncertainty-based joint supervised and self-supervised learning, the negative effects of the self-supervised learning of 360
$^{\circ }$
depths are filtered out based on the epistemic uncertainties of the AMDN. Therefore, only the positive effects of self-supervised learning can be realized. Through extensive experiments, we demonstrate that the proposed approaches achieve much more accurate depths as compared with very recent studies for various datasets. Moreover, the proposed approaches also yield sophisticated uncertainties in a single forward path, in which previous studies could not.
更多查看译文
关键词
360 $^{\circ }$ depth estimation,mixture density network,uncertainty,self-supervised learning
AI 理解论文
溯源树
样例
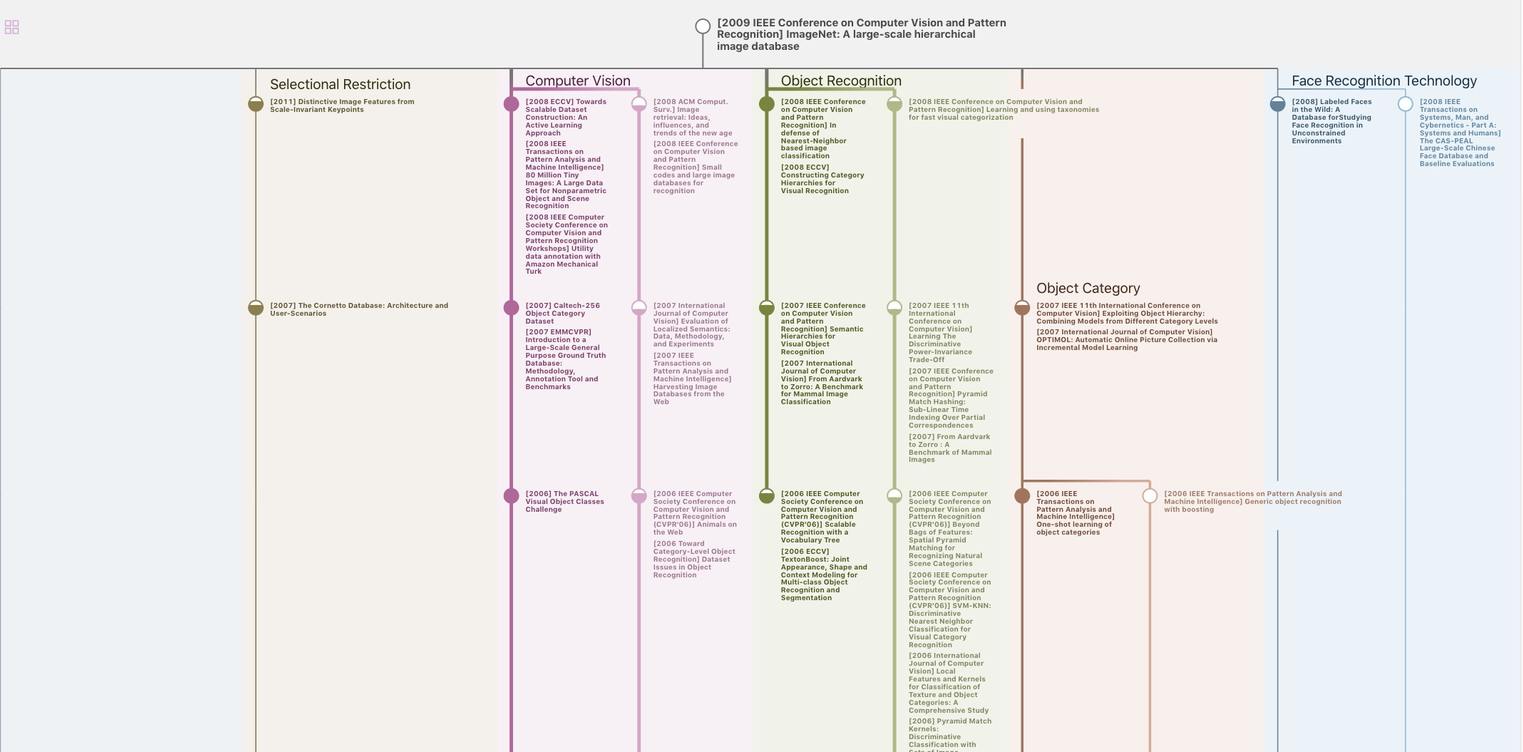
生成溯源树,研究论文发展脉络
Chat Paper
正在生成论文摘要