Few-Shot Contrastive Transfer Learning With Pretrained Model for Masked Face Verification.
IEEE Trans. Multim.(2024)
摘要
Face verification has seen remarkable progress that benefits from large-scale publicly available databases. However, it remains a challenge how to generalize a pretrained face verification model to a new scenario with a limited amount of data. In many real-world applications, the training database only contains a limited number of identities with two images for each identity due to the privacy concern. In this paper, we propose to transfer knowledge from a pretrained unmasked face verification model to a new model for verification between masked and unmasked faces, to meet the application requirements during the COVID-19 pandemic. To overcome the lack of intra-class diversity resulting from only a pair of masked and unmasked faces for each identity (
$i.e.,$
two shots for each identity), a static prototype classification function is designed to learn features for masked faces by utilizing unmasked face knowledge from the pretrained model. Meanwhile, a contrastive constrained embedding function is designed to preserve unmasked face knowledge of the pretrained model during the transfer learning process. By combining these two functions, our method uses knowledge acquired from the pretrained unmasked face verification model to proceed with verification between masked and unmasked faces with a limited amount of training data. Extensive experiments demonstrate that our method can perform better than state-of-the-art methods for verification between masked and unmasked faces in the few-shot transfer learning setting.
更多查看译文
关键词
face verification,transfer learning,few-shot learning,masked face,COVID-19
AI 理解论文
溯源树
样例
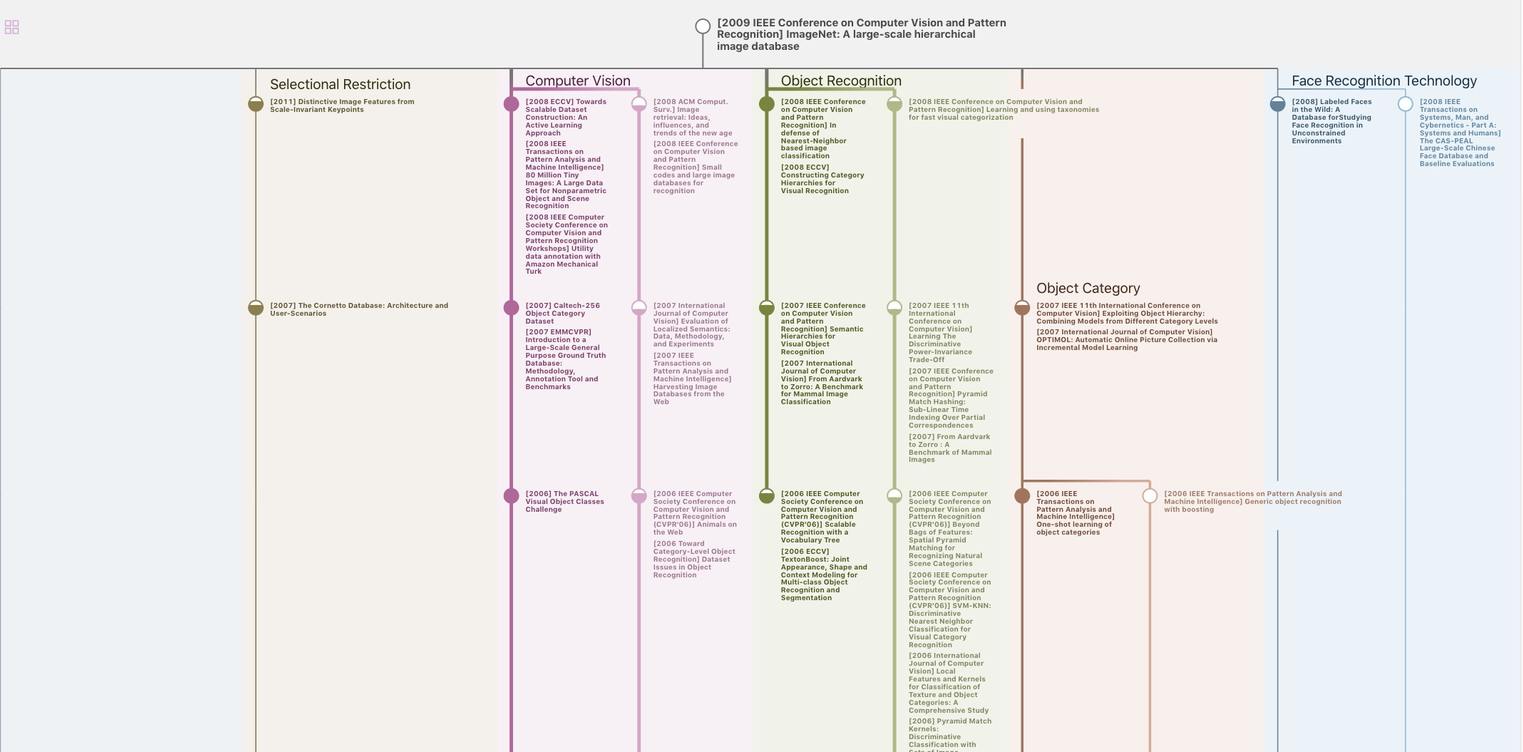
生成溯源树,研究论文发展脉络
Chat Paper
正在生成论文摘要