Data-driven fault detection in a thermocouple network using neighbouring redundancy, XGBoost classifier and up-down counter
IEEE Sensors Journal(2023)
摘要
Fault Detection and Isolation (FDI) is of great interest for the control community since it can drive improved performance in a system by allowing predictive maintenance/repairing and catering for improved operational safety. Fault Detection and Isolation in large-scale smelting furnaces presents several challenges, as it requires the understanding of complex thermal and chemical reactions occurring inside the structure. Furthermore, the impossibility of having full operational information about the process makes the use of model-based methods very complex or unfeasible. This paper introduces a methodology to develop a Data-Driven FDI system for the detection of incipient and intermittent failures in a network made out of 322 thermocouples located on the shell of the furnace. Statistical metrics over Fault Counter Time Windows (FTCW) were used to identify different types of sensor failures, which led to establishing a baseline of known failure events and to create a dataset to train the Machine Learning (ML) classification models. A data-driven approach was proposed based on the sensors physical (neighbouring) redundancy, which led to some type of physical redundancy. A post-processing stage was used to stabilize the model’s response in time, determining that the proposed FDI system successfully detects faults whilst reducing reported false negatives.
更多查看译文
关键词
FDI,machine learning,parameter variation,redundancy,sensors network,thermocouple,up-down counter,XGBoost
AI 理解论文
溯源树
样例
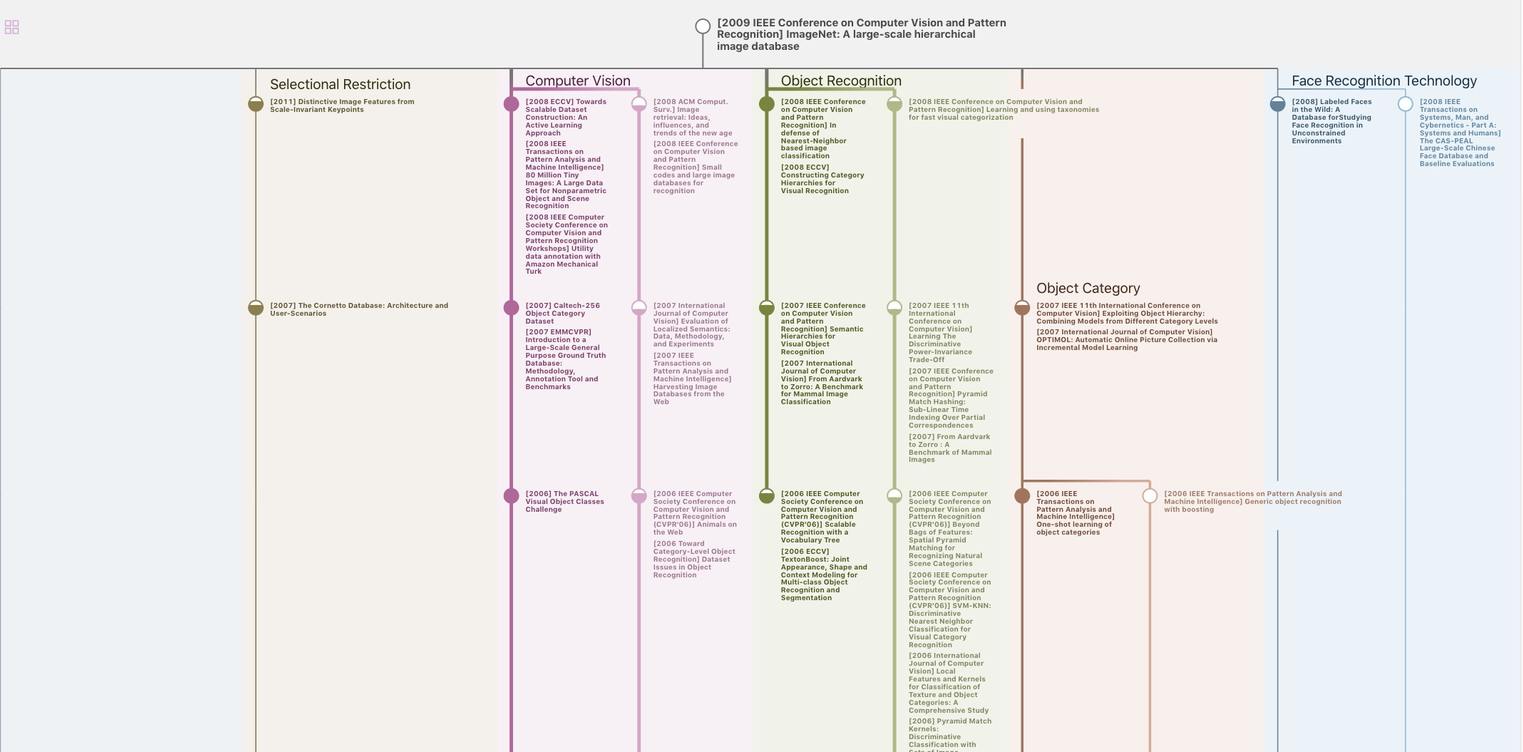
生成溯源树,研究论文发展脉络
Chat Paper
正在生成论文摘要