Federated Synchrophasor Data Prediction, Aggregation and Inference Using Deep Learning: A Case of Proactive Control for Short-Term Stability
IEEE Transactions on Power Delivery(2023)
摘要
A novel asynchronous federated architecture is proposed in this work for data prediction, aggregation, and inference with a use case for fast short-term instability mitigation in transmission systems. Existing machine learning (ML) prediction approaches rely on centralized-schemes that accumulate measurements from distributed regions at a central computing node. require all the measurement data from distributed regions to be accumulated at a central computing node. This centralized-schemes increase
computational overhead
leading to prediction delay. Federated-learning collaboratively learns a global model without centralized data processing. Nevertheless, its performance degrades when data becomes
non-independent and identically-distributed
due to the changes in grid dynamics, leading to expensive model re-training. This research proposes a
federated-inference architecture
that can exploit data-dependency in different regions to effectively exchange model parameters among high-quality local models and improve local predictive results, hence avoiding the need for global model and re-training effort. The architecture includes several major modules: a) federated deep ML models using gated-recurrent unit for local synchrophasor data prediction in each region, b) computationally-efficient data anomaly detection and mitigation using statistical and unsupervised autoencoders, c) a federated inference algorithm that aggregates model weights and outputs from top-
$k$
correlated neighbors to improve local performance, upon system dynamics change, d) instability prediction and e) a case analysis for its application in proactive short-term stability control. The efficiency and effectiveness of the proposed approach is validated on modified IEEE test systems with multiple test event scenarios with varying factors, including number of PMUs, error tolerances, and length of input and prediction windows.
更多查看译文
关键词
Federated inference,machine learning,short-term stability,synchrophasor data,unsupervised learning
AI 理解论文
溯源树
样例
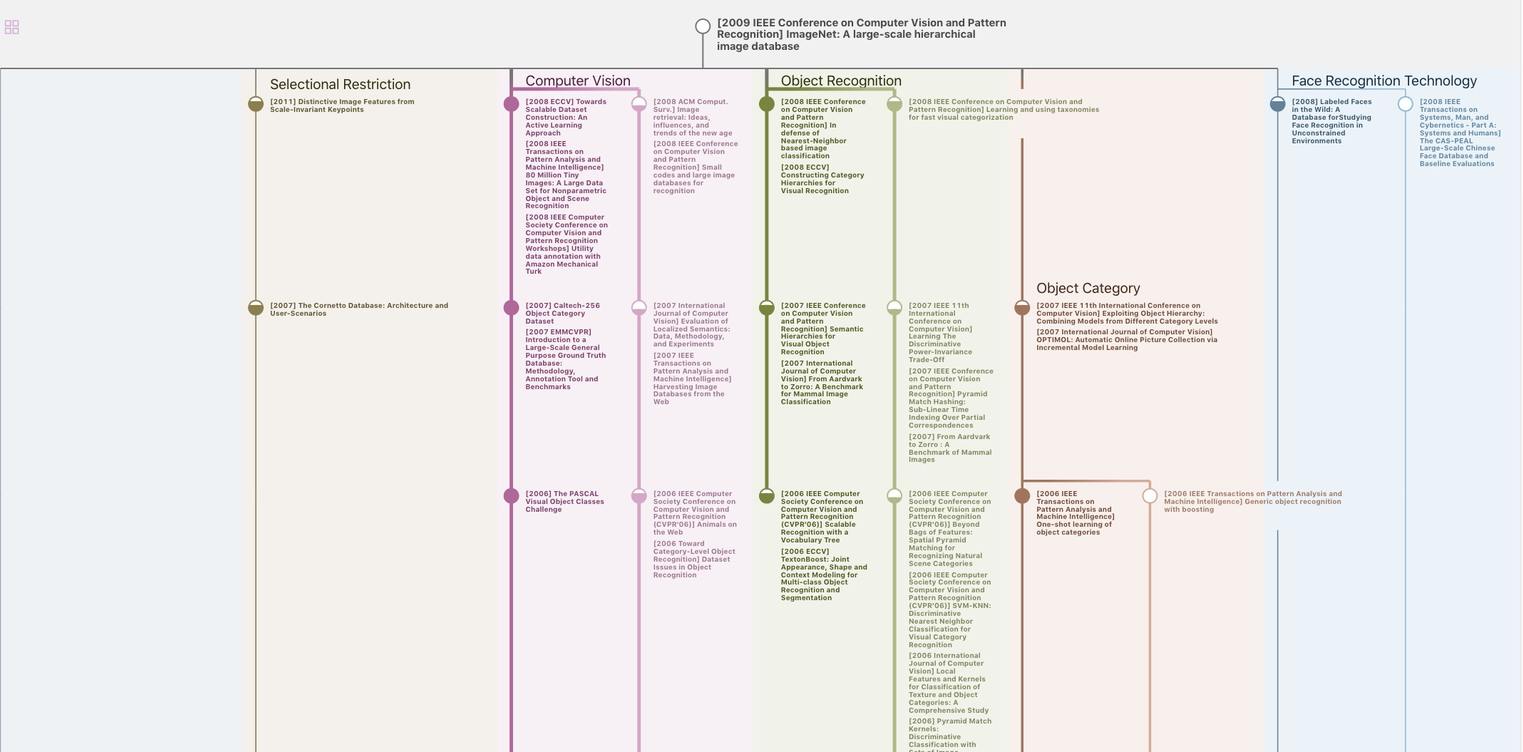
生成溯源树,研究论文发展脉络
Chat Paper
正在生成论文摘要