Soft Weight Pruning for Cross-Domain Few-Shot Learning with Unlabeled Target Data
IEEE Transactions on Multimedia(2024)
摘要
Cross-domain few-shot learning (CDFSL) has received great interest for its effectiveness in solving the problem of the shift between source and target domains in few-shot scenarios. To extract more representative features, recent CDFSL works have exploited small-scale unlabeled samples from the target domain during the feature extraction phase. Existing self-supervised CDFSL methods, however, typically fine-tune the weights of the pre-trained model without taking into account the mismatch between source and target domains. To address this shortcoming, we introduce a self-supervised soft weight pruning strategy for cross-domain few-shot classification tasks with unlabeled target data. Starting from a pre-trained network from the source domain, our approach iterates between pruning out the relatively unimportant connections of the network and reactivating the pruned connections in a joint contrastive and
$L^{2}$
-
SP
regularized training framework. By combining the soft weight pruning strategy and regularization, our method effectively restricts redundant weights while simultaneously learning crucial features for both source and target tasks. Our approach, in comparison to other methods, does not involve any additional modules in the models; however, it can still achieve remarkable performance. Our approach can be efficiently incorporated into a variety of contrastive learning methods in a plug-and-play fashion. Extensive experimental results on several benchmark datasets demonstrate that our proposed method outperforms existing representative cross-domain few-shot methods by a large margin. The code for our work can be found at
https://github.com/nuistji/swp-cdfsl
.
更多查看译文
关键词
Cross-domain few-shot learning,self-supervised,soft weight pruning,regularized training
AI 理解论文
溯源树
样例
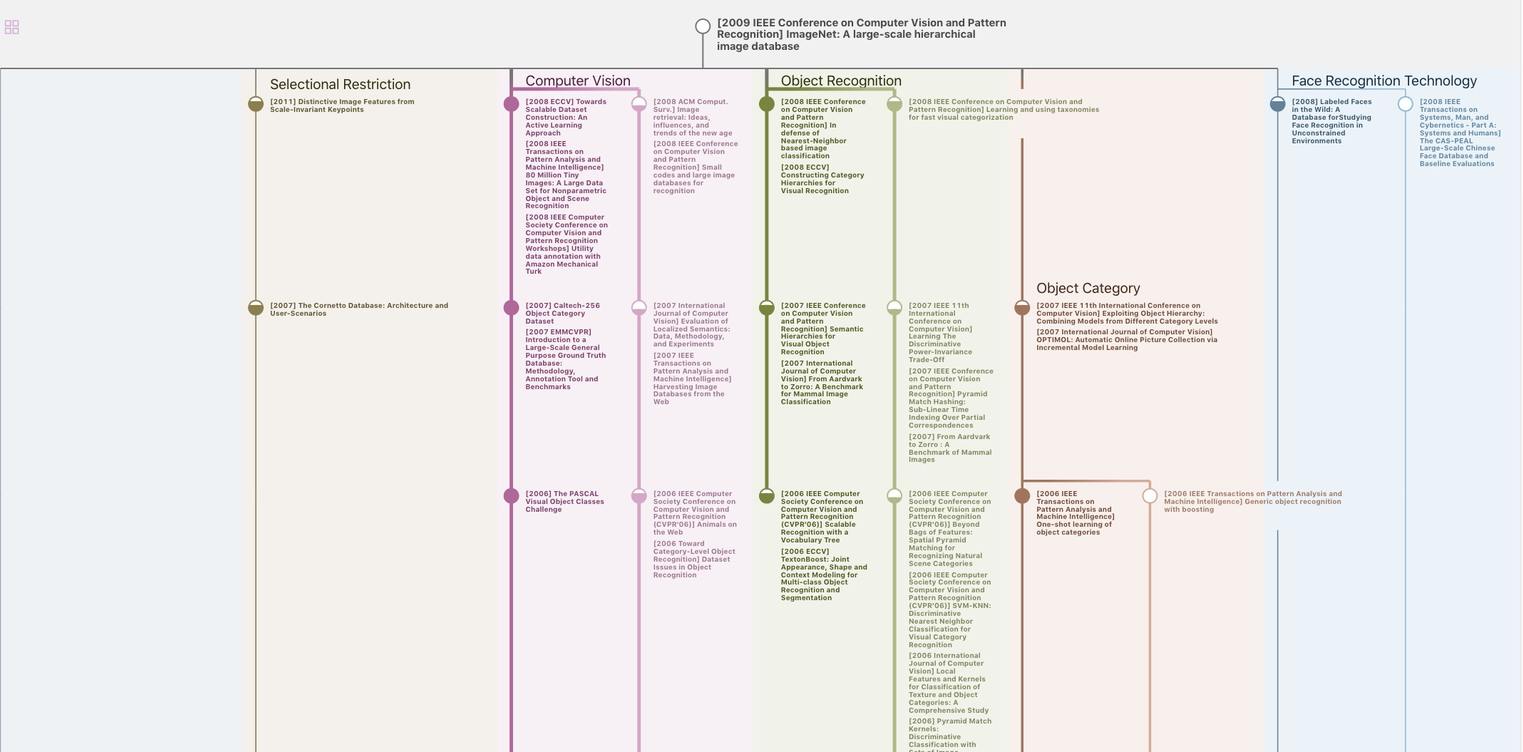
生成溯源树,研究论文发展脉络
Chat Paper
正在生成论文摘要