How Often Channel Estimation is Required for Adaptive IRS Beamforming: A Bilevel Deep Reinforcement Learning Approach
IEEE Transactions on Wireless Communications(2024)
摘要
In an intelligent reflecting surface (IRS)-assisted wireless communication system, obtaining the real-time channel state information (CSI) through channel estimation (CE) is crucial for achieving the IRS’s passive beamforming gain, which however shortens the effective data transmission time due to the CSI feedback overhead. It is of utmost importance to decide how often to estimate the channels in an IRS-assisted system. In this paper, we propose an integrated CE and beamforming scheme to jointly optimize the adaptive CE interval and passive beamforming strategy, based on the past observation sequences composed of imperfect CSI and data rate feedback. We formulate the two-stage optimization problem as a bilevel partially observable Markov decision process (POMDP), aiming to maximize the expectation of cumulative throughput of the system. We propose two bilevel deep reinforcement learning (DRL) algorithms, namely recurrent neural network (RNN) based proximal policy optimization (PPO) algorithm and Belief-based PPO algorithm, to solve this problem. In these two algorithms, the CSI features from the past observation sequences are implicitly extracted by the RNN network or explicitly inferred by the belief network, which then serve as the inputs for the two-stage policy networks to determine the necessity of CE and the IRS beamforming vector based on the PPO algorithm. Simulation results demonstrate the superiority of the proposed adaptive CE scheme over the periodic counterpart in terms of throughput. Moreover, the results show that it is profitable to estimate the channels less frequently if the channels exhibit a higher correlation across time.
更多查看译文
关键词
Intelligent reflecting surface,passive beamforming,partially observable Markov decision process,deep reinforcement learning
AI 理解论文
溯源树
样例
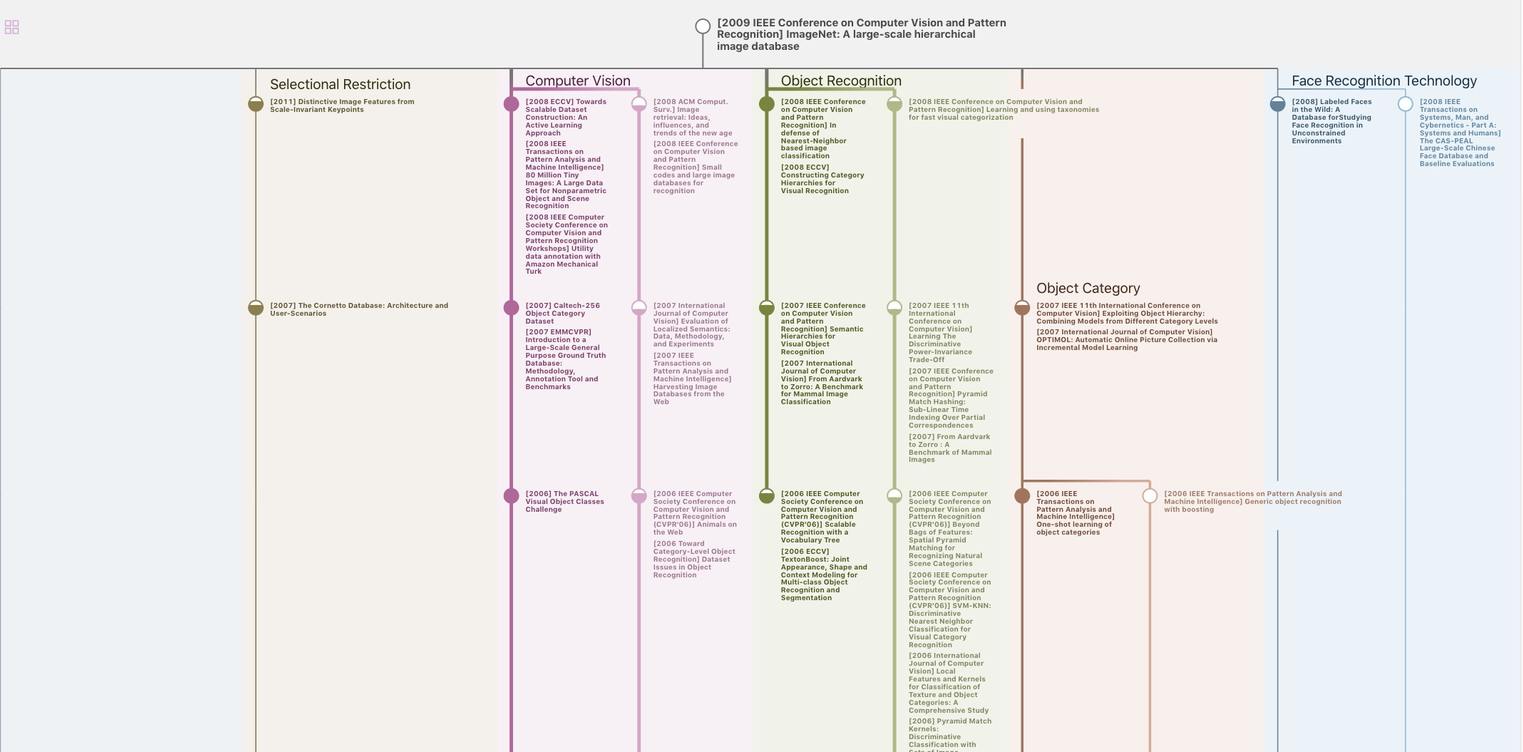
生成溯源树,研究论文发展脉络
Chat Paper
正在生成论文摘要