A 28.8-mW Accelerator IC for Dark Channel Prior-Based Blind Image Deblurring
IEEE JOURNAL OF SOLID-STATE CIRCUITS(2023)
摘要
This work presents an accelerator that performs blind deblurring based on the dark channel prior. The alternating minimization algorithm is leveraged for latent image and blur kernel estimation. A 2-D Laplace equation solver is embedded to reduce the latency by 56% for boundary wrapping. For latent image estimation, gradient data locality is employed to reduce the latency by 57%. A sorting engine is designed to reduce the latency in data access by 96% for calculating the dark channel. A pipelined mixed-radix 1-D fast Fourier transform (FFT) engine is used for efficient latent image estimation and blur kernel estimation. By employing image size approximation, 85% of additions and 97% of multiplications for FFT can further be saved. In the blur kernel estimator, a 2-D convolution engine with a parallel architecture is implemented, reducing the latency by 79%. The accelerator supports blur kernels of 25 x 25 and 49 x 49 pixels for blurred images of 129 x 129 and 257 x 257 pixels, respectively. Fabricated in 40-nm CMOS, the accelerator's core area is 3.98 mm(2). The chip dissipates 28.8 mW at 65 MHz from a 0.65-V supply. It can estimate a blur kernel of 25 x 25 pixels for an image patch with 129 x 129 pixels for deblurring a full-HD image in 1.7 s, achieving a 2562x shorter latency than a high-end CPU. Compared with the state-of-theart design, the chip achieves a four times higher normalized area efficiency and a 7.5x higher normalized energy efficiency.
更多查看译文
关键词
Alternating minimization,blind image deblurring,CMOS integrated circuits,energy-efficient architecture,hardware accelerator
AI 理解论文
溯源树
样例
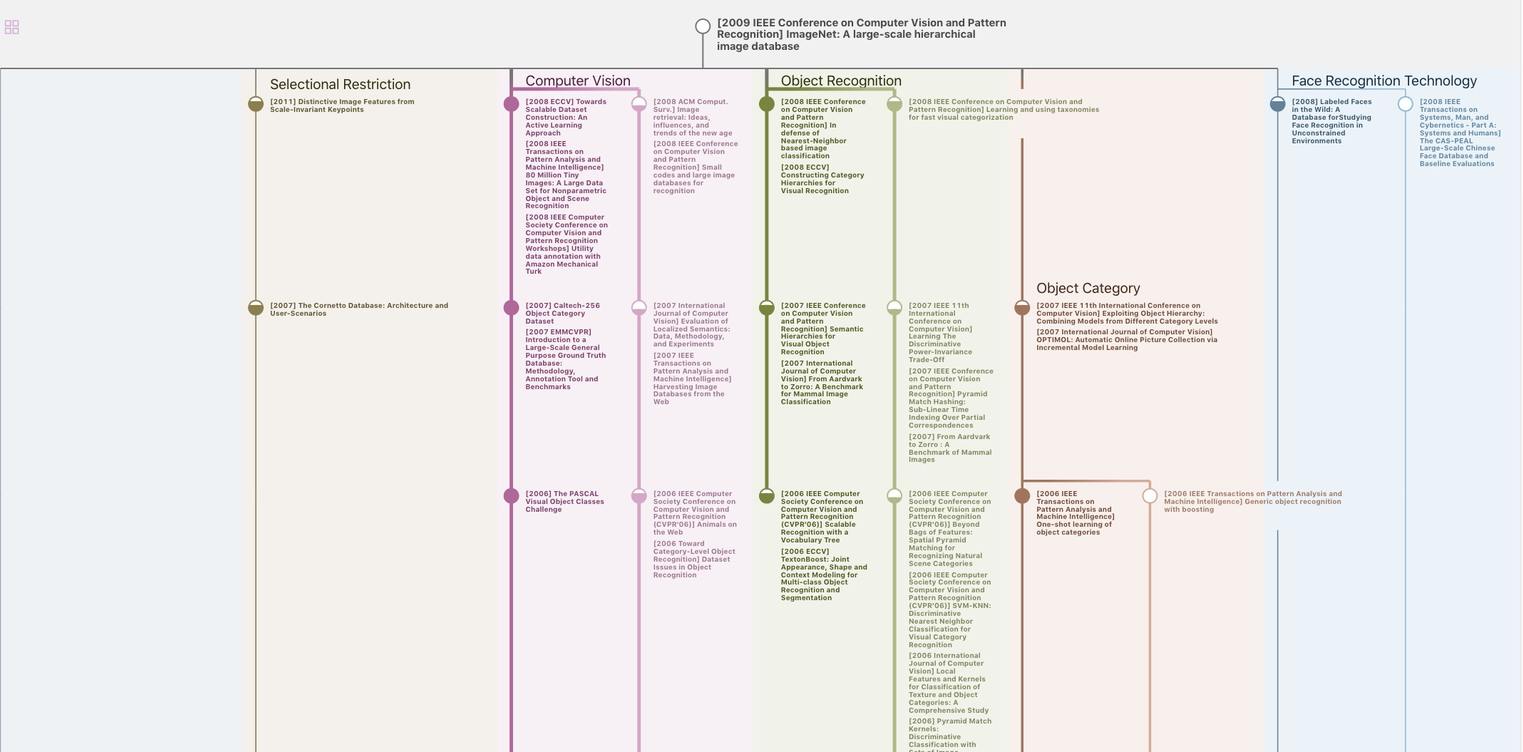
生成溯源树,研究论文发展脉络
Chat Paper
正在生成论文摘要