Incentive Mechanism for Federated Learning With Random Client Selection.
IEEE Trans. Netw. Sci. Eng.(2024)
摘要
Federated learning (FL) is a distributed machine learning framework allowing edge devices (a.k.a clients) to participate in training while protecting their privacy. While much research in this field focuses on improving training performance and reducing communication costs, how to incentivize clients to participate in FL still remains a challenge. Most existing FL algorithms assume that clients voluntarily participate in the training process, which is unrealistic. This paper proposes an incentive mechanism for FL servers to motivate clients to contribute their data and computing power to local training. The mechanism consists of two steps. First, a subset of clients is selected randomly under an importance sampling scheme. Then, the interaction between the server and the subset of sampled clients is modeled as a Stackelberg game, where the server releases offers to the clients based on their potential contributions. The clients then decide how much data and computation to contribute. We prove that the client-level subgame of the Stackelberg game has a subgame equilibrium that can be written in a semi-closed form. We also propose an approximation algorithm for computing the subgame equilibrium of the server's level subgame. Our simulation results verify the analysis and demonstrate the effectiveness of the proposed mechanism.
更多查看译文
AI 理解论文
溯源树
样例
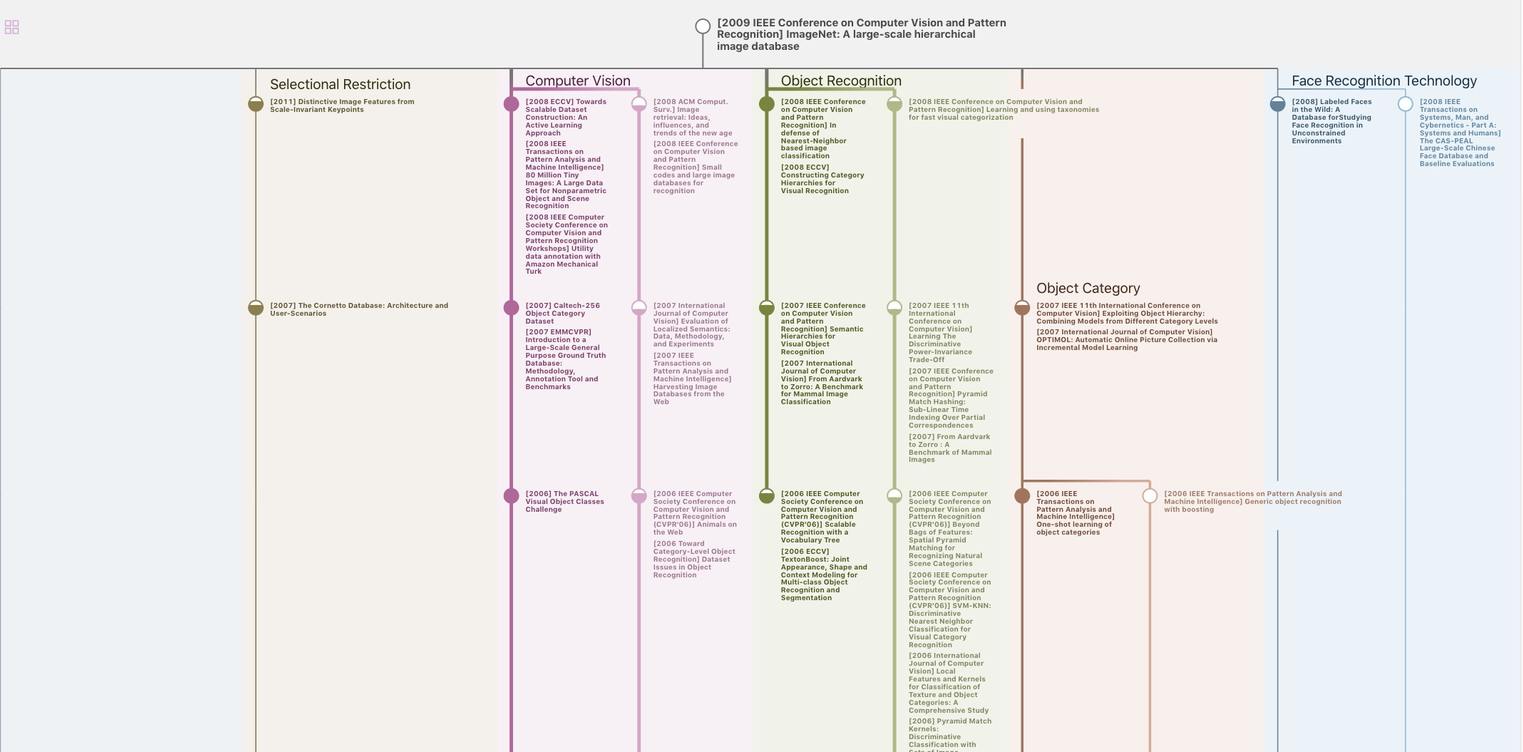
生成溯源树,研究论文发展脉络
Chat Paper
正在生成论文摘要