TPGraph: A Spatial-Temporal Graph Learning Framework for Accurate Traffic Prediction on Arterial Roads
IEEE TRANSACTIONS ON INTELLIGENT TRANSPORTATION SYSTEMS(2024)
摘要
The accurate prediction of traffic conditions, including speed, flow, and travel time, poses a critical challenge in urbanization that significantly impacts car owners and road administrators. However, in certain scenarios with restricted road data availability (e.g. lack of traffic light status and signal control strategies, cooperation between road administrators and third parties, etc.), it is imperative to make effective use of basic road information (e.g. historical traffic data and road connectivity) to improve both prediction accuracy and scalability on various arterial road networks against state-of-art deep learning models. In this paper, we propose a spatial-temporal learning framework TPGraph for an accurate prediction of arterial roads' traffic data by effectively utilizing upstream and downstream road information. TPGraph is composed of three major parts: 1) A multi-scale temporal feature fusion module that utilizes a multi-head attention mechanism to integrate recently-periodic features, daily-periodic features, and weekly-periodic features; 2) A multi-graph convolution module that employs graph fusion and graph convolution networks to capture richer spatial semantics, and 3) A dynamic spatial-temporal prediction module that leverages a spatial-temporal transformer for single or multiple traffic-state predictions. Our proposed framework, TPGraph, leverages just multi-scale historical traffic conditions and readily accessible spatial factors as input to generate accurate predictions of future traffic conditions. We mainly evaluate the performance of our approach through multi-step prediction experiments conducted at hourly intervals, forecasting travel time or travel speed for each road at 15 mins, 30 mins, and 1 hour. Furthermore, we conduct extensive experiments on real-world arterial road datasets to demonstrate the superior predictive performance of TPGraph compared to existing methods.
更多查看译文
关键词
Roads,Feature extraction,Data mining,Convolutional neural networks,Convolution,Transformers,Predictive models,Traffic prediction,spatial-temporal transformer,multi-head attention mechanism,graph neural networks
AI 理解论文
溯源树
样例
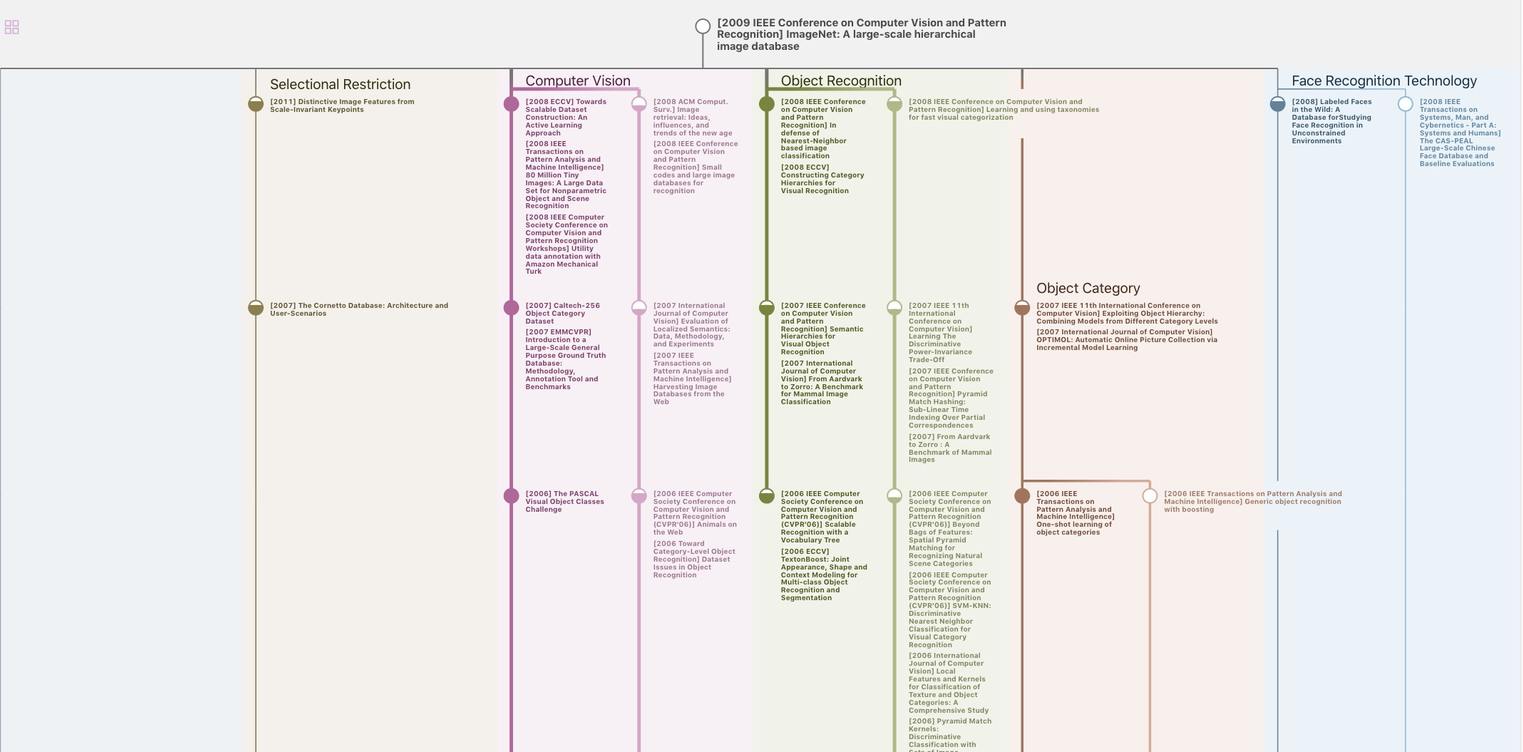
生成溯源树,研究论文发展脉络
Chat Paper
正在生成论文摘要