Secure and Enhanced Online Recommendations: A Federated Intelligence Approach
IEEE Transactions on Consumer Electronics(2023)
摘要
The ever-increasing social and economic activities on online platforms, demand data protection and privacy regulations worldwide. The existing datasets and open-source repositories that are developed for building online recommendation systems for consumers are valuable sources. Nonetheless, these systems are vulnerable to cyber-attacks where intruders can introduce fraudulent profiles into the rating database with the intention of manipulating the system’s results. To tackle this issue, a security framework that utilizes federated intelligence and a deep deterministic policy gradient is proposed. This framework aims to mitigate potential model-free fake data injection attacks, jeopardizing the rating behavior to assess the vulnerability by exploiting the business value measures such as the number of clicks of a recommendation system. The framework uses optimized federated intelligent detection to detect any malicious activity while keeping consumers’ privacy intact. Compared to existing techniques, it provides cost-effective and highly accurate detection of malicious activity to enhance the reliability of the recommendation system while protecting consumers’ privacy in selecting online products such as consumer electronics, movies, or any other online services. The outcomes demonstrate that the suggested framework outperforms existing methods by achieving exceptionally high scores in the recall, F-score, and precision score, as high as 95.5%, 95.3%, and 95.9%, respectively.
更多查看译文
关键词
Collaborative recommendation system,consumer electronics,fake data injection,federated learning,security,privacy
AI 理解论文
溯源树
样例
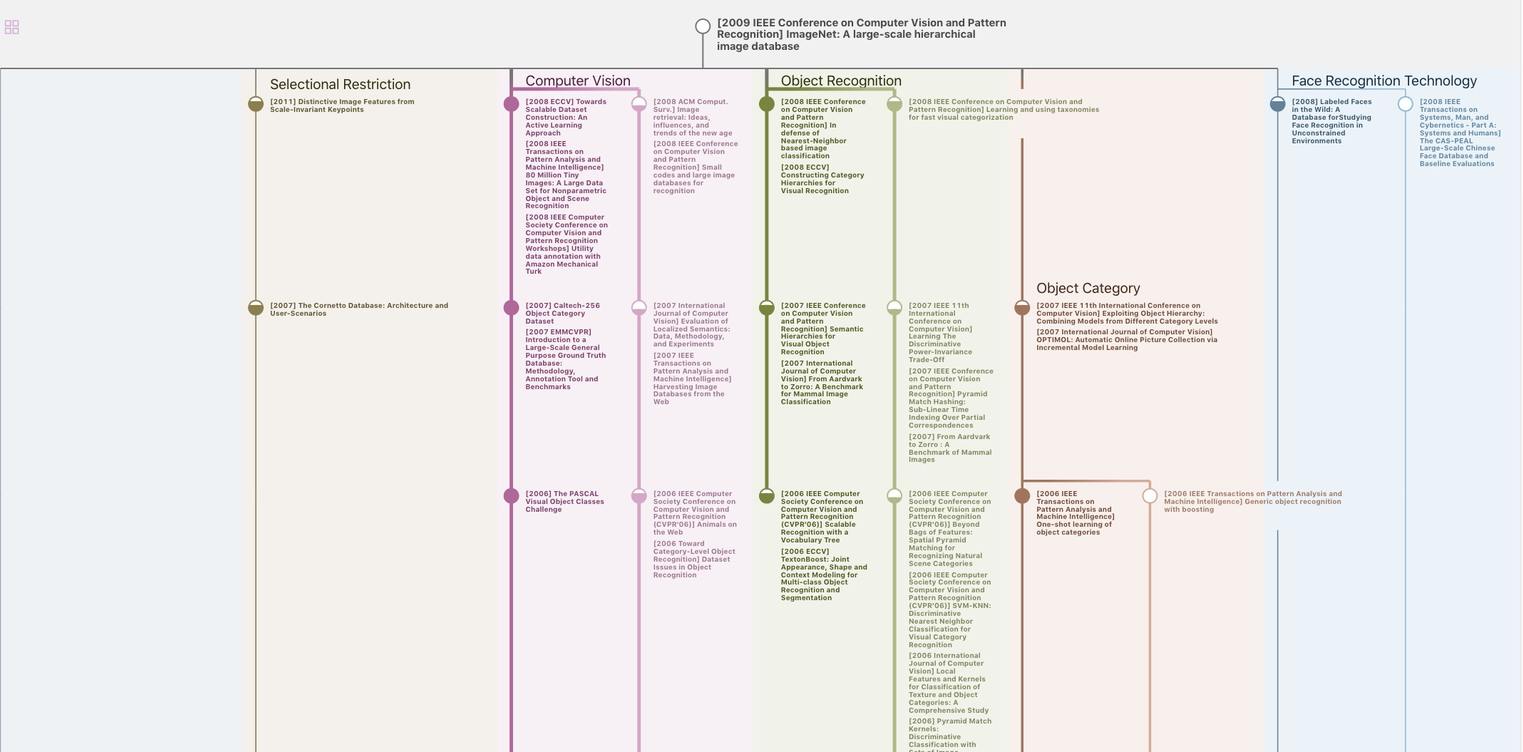
生成溯源树,研究论文发展脉络
Chat Paper
正在生成论文摘要