Fall-Attention: An Attention-Based Fall Detection Method for Adjoint Activities
IEEE Transactions on Mobile Computing(2023)
摘要
WiFi-based wireless sensing has gained popularity for enabling smart indoor services, one of which is fall detection which plays a vital role in mitigating health risks for elders. Previous approaches have treated daily activities as independent events and built models to distinguish falls from others. However, human activities are usually adjoint in practice, e.g., the elder may suddenly fall when she walks. This adjoining introduces shared features between different activities, thereby affecting the classification performance. To address this problem, we propose Fall-attention, an attention-based fall detection method that can focus on the features related to fall events and suppress interference of irrelevant activities to improve performance. Its basic idea is to produce a task-oriented feature representation of fall events inside the signal using attention-based sentence embedding techniques and Recurrent Neural Network (RNN). We incorporate multi-task learning into Fall-attention by adopting multiple independent classification modules. This enables the model to explore different regions of the signal, capturing the composition of adjoint activities. A series of signal preprocessing and data enhancement techniques are also adopted to promote model training. Experimental results of the dataset containing adjoint activities demonstrate the superiority of Fall-attention over previous methods, which achieves an average accuracy of 95%.
更多查看译文
关键词
WiFi sensing,fall detection,human activity recognition (HAR),natural language processing (NLP)
AI 理解论文
溯源树
样例
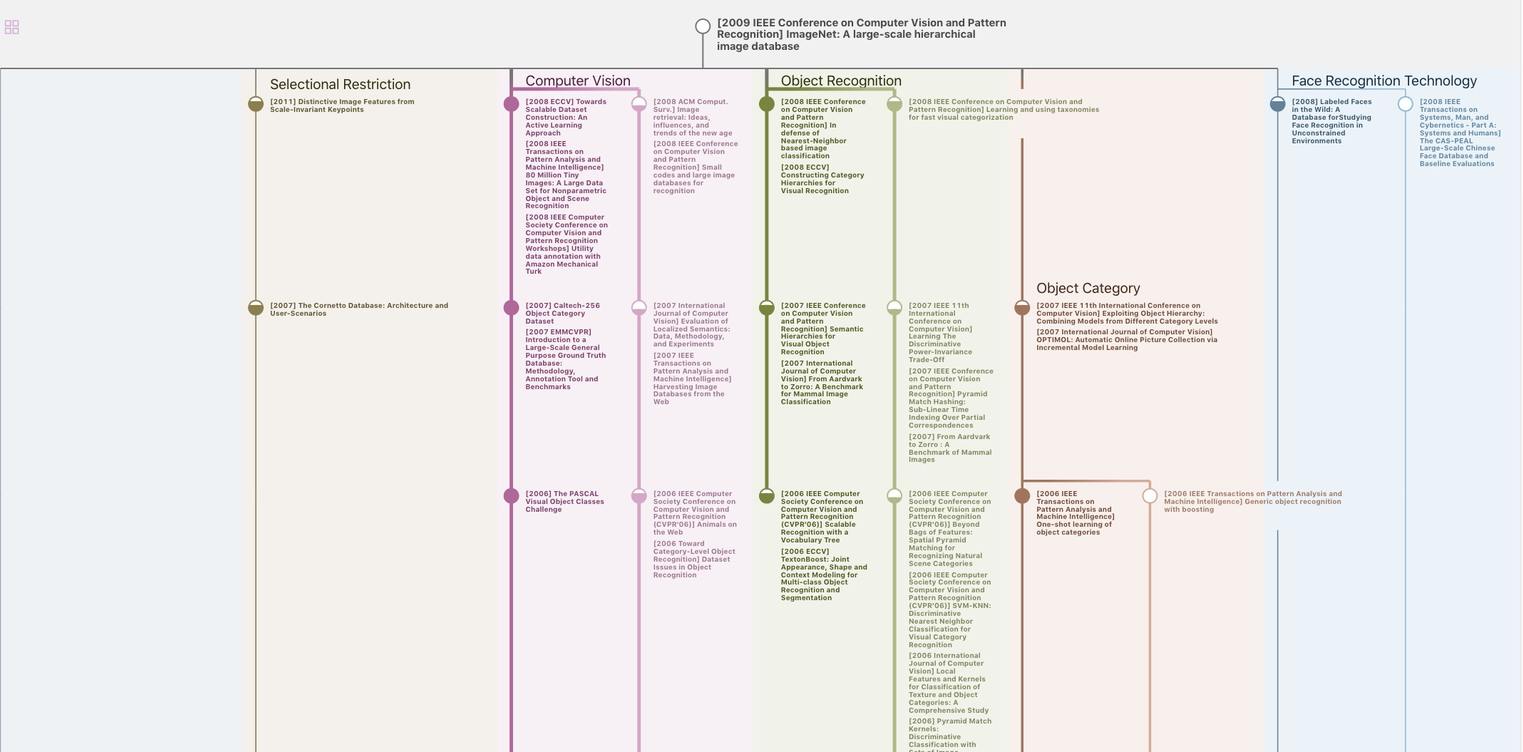
生成溯源树,研究论文发展脉络
Chat Paper
正在生成论文摘要