FLOW: A Robust Federated Learning Framework to Defend Against Model Poisoning Attacks in IoTs
IEEE Internet of Things Journal(2023)
摘要
Federated Learning (FL) is a promising distributed learning approach to enable intelligent Internet of Things (IoT) applications. However, FL is vulnerable to model poisoning attacks in which malicious clients abate the accuracy of the global model by committing crafted local model updates to the server. Existing defense methods either rely on a validation dataset or simply remove the detected malicious clients from the subsequent training process to handle attacks from a large number of malicious clients. Thus, the performance of existing methods deteriorates drastically in many scenarios where the data distributions of clients are unpredictable. To address these deficiencies, we propose a framework called FL overwatch (FLOW) to efficiently defend against model poisoning attacks taking advantages of the local model updates in current and historical training iterations. On one hand, FLOW detects malicious clients in each iteration by measuring the cosine distances between the local model updates of clients, such that malicious updates are eliminated from the current aggregation. On the other hand, FLOW gracefully punishes the previously identified malicious clients rather than removes them from the whole training process. As a result, FLOW can embrace a richer reliable set of local model updates than existing methods in aggregation. Extensive experiments on widely-used benchmark datasets show that FLOW can achieve higher success defending ratio and higher accuracy of global models over existing Byzantine-robust FL methods under typical untargeted attacks and targeted attacks. Furthermore, FLOW also shows significant effectiveness in defending against adaptive attacks tailored to FLOW.
更多查看译文
关键词
Federated learning (FL),model poisoning attacks,Internet of Things,model aggregation
AI 理解论文
溯源树
样例
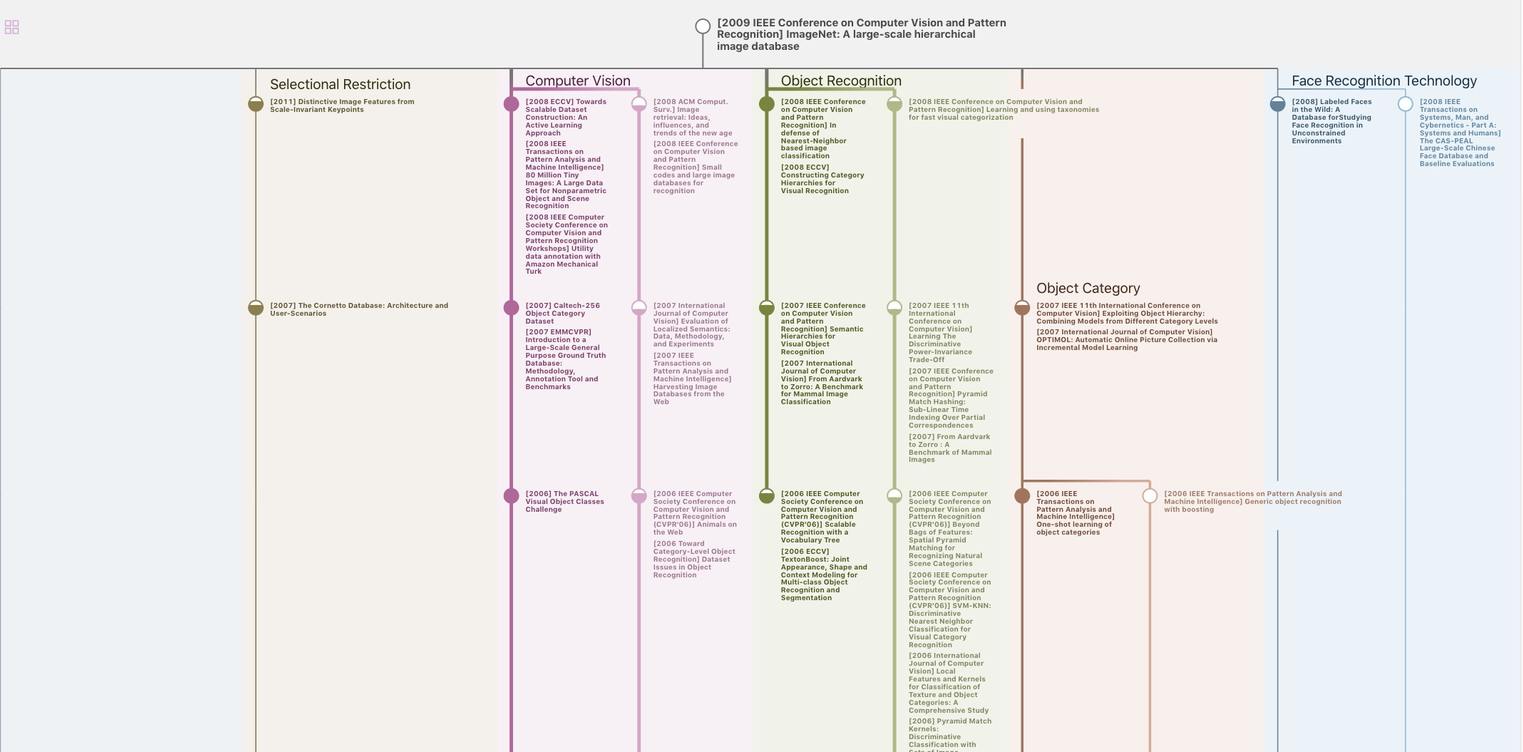
生成溯源树,研究论文发展脉络
Chat Paper
正在生成论文摘要