Sparse Mobile Crowdsensing for Cost-Effective Traffic State Estimation With Spatio-Temporal Transformer Graph Neural Network.
IEEE Internet Things J.(2024)
摘要
Recently, mobile crowdsensing (MCS) has emerged as a promising solution for traffic state estimation (TSE), which provides real-time and accurate traffic information for supporting diversified intelligent transportation systems (ITS) applications. However, the prohibitive overhead of collecting massive data in vehicular networks limits the available data amount, while the sparsification of MCS data incurs instability and degrades TSE accuracy. To this end, this paper proposes a novel sparse MCS framework to facilitate cost-effective TSE, which utilizes a small number of vehicular MCS participants distributed across all regions as data sources. By utilizing spatial and temporal correlations of traffic flow, an innovative spatiotemporal deep learning model, namely Transformer Graph Attentional Sample and Aggregate neural network (TGASA), is proposed to improve the TSE accuracy with sparse MCS data. Specifically, we design an incorporated graph neural network (GNN) to aggregate the spatial correlation by taking both node features and edge properties into account. And, the transformer neural network architecture is applied to capture the temporal correlation. Extensive simulation results based on real-world datasets demonstrate that the proposed framework can significantly address the instability incurred by the sparsification of MCS data and effectively achieve a more accurate TSE.
更多查看译文
关键词
Mobile crowdsensing,vehicular network,traffic state estimation,graph neural network,transformer
AI 理解论文
溯源树
样例
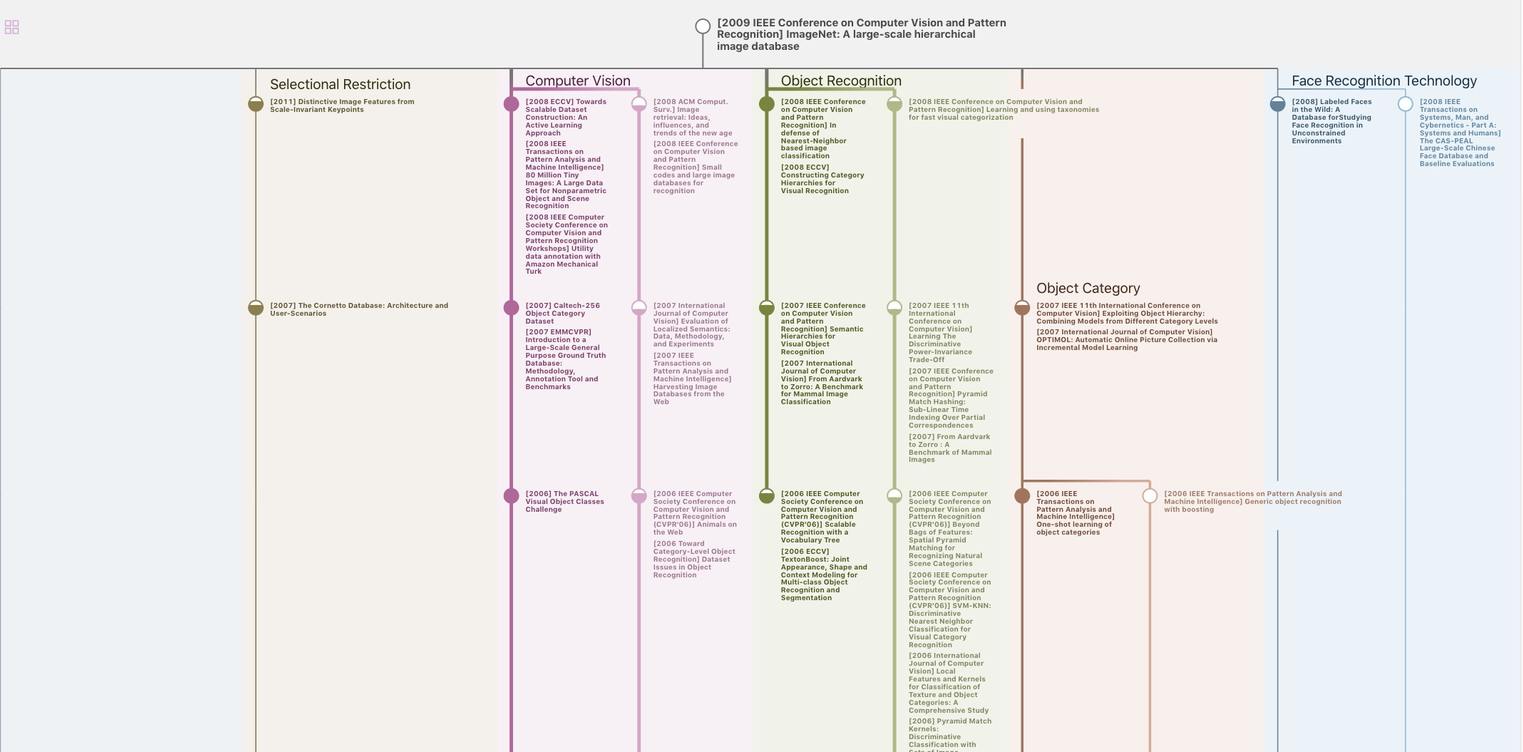
生成溯源树,研究论文发展脉络
Chat Paper
正在生成论文摘要