Process Model-Assisted Optimization Control Method Based on Model Predictive Control and Deep Reinforcement Learning for Coordinated Control System in Coal-Fired Power Unit
IEEE TRANSACTIONS ON AUTOMATION SCIENCE AND ENGINEERING(2024)
摘要
As the large-scale integration of renewable energy into the power grid, coal-fired power units have to undertake the task of load-frequency regulation, the optimization control of the coal-fired power units is becoming increasingly important. Reinforcement learning is being used for optimization control of the process increasingly but may encounter high learning costs. In this paper, a process model-assisted optimization control framework called PMA-OC is proposed to achieve the optimization control of coal-fired power unit. In this framework, a low-precision process model developed by process operation knowledge is employed to achieve the initial control based on model predictive control algorithm (MPC) and improve the dynamic characteristics of the coordinated control system. Then the deep reinforcement learning algorithm (DRL) is utilized to improve the initial control continuously, through constantly interacting with the improved process. With the full mining of process knowledge from the power unit, the improved process offers the better training samples for DRL, reduces the learning complexity of DRL, and strengthens the learning efficiency of DRL. Finally, the proposed control framework is employed to achieve the optimization control of the coordinated control system, and simulation results indicate that the proposed control framework outperforms the existing standalone MPC and DRL methods in terms of anti-interference ability, dynamic response time, and performs well control capability.
更多查看译文
关键词
Optimization,Heuristic algorithms,Control systems,Turbines,Boilers,Predictive control,Predictive models,Process knowledge,deep reinforcement learning,coordinated control system,coal-fired power unit
AI 理解论文
溯源树
样例
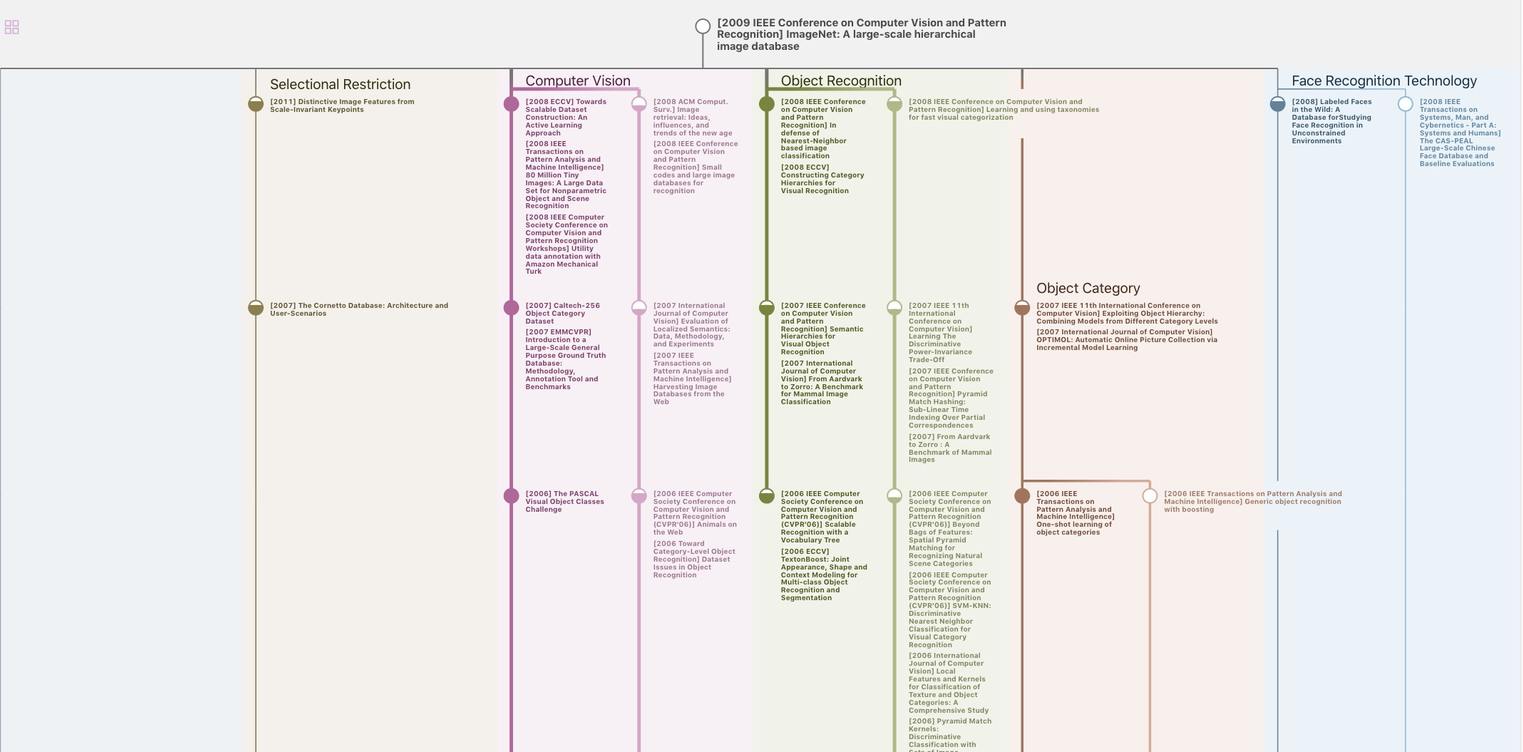
生成溯源树,研究论文发展脉络
Chat Paper
正在生成论文摘要