One for All: A Unified Generative Framework for Image Emotion Classification
IEEE Transactions on Circuits and Systems for Video Technology(2023)
摘要
Image Emotion Classification (IEC) is an essential research area, offering valuable insights into user emotional states for a wide range of applications, including opinion mining, recommendation systems, and mental health treatment. The challenges associated with IEC are mainly attributed to the complexity and ambiguity of human emotions, the lack of a universally accepted emotion model, and excessive dependence on prior knowledge. To address these challenges, we propose a novel Unified Generative framework for Image Emotion Classification (UGRIE), which is capable of simultaneously modeling various emotion models and capturing intricate semantic relationships between emotion labels. Our approach employs a flexible natural language template, converting the IEC task into a template-filling process that can be easily adapted to accommodate a diverse range of IEC tasks. To further enhance the performance, we devise a mapping mechanism to seamlessly integrate the multimodal pre-training model CLIP with the text generation pre-training model BART, thus leveraging the strengths of both models. A comprehensive set of experiments conducted on multiple public datasets demonstrates that our proposed method consistently outperforms existing approaches to a large margin in supervised settings, exhibits remarkable performance in low-resource scenarios, and unifies distinct emotion models within a single, versatile framework.
更多查看译文
关键词
Pre-training model,images emotion classification,multi-modal learning
AI 理解论文
溯源树
样例
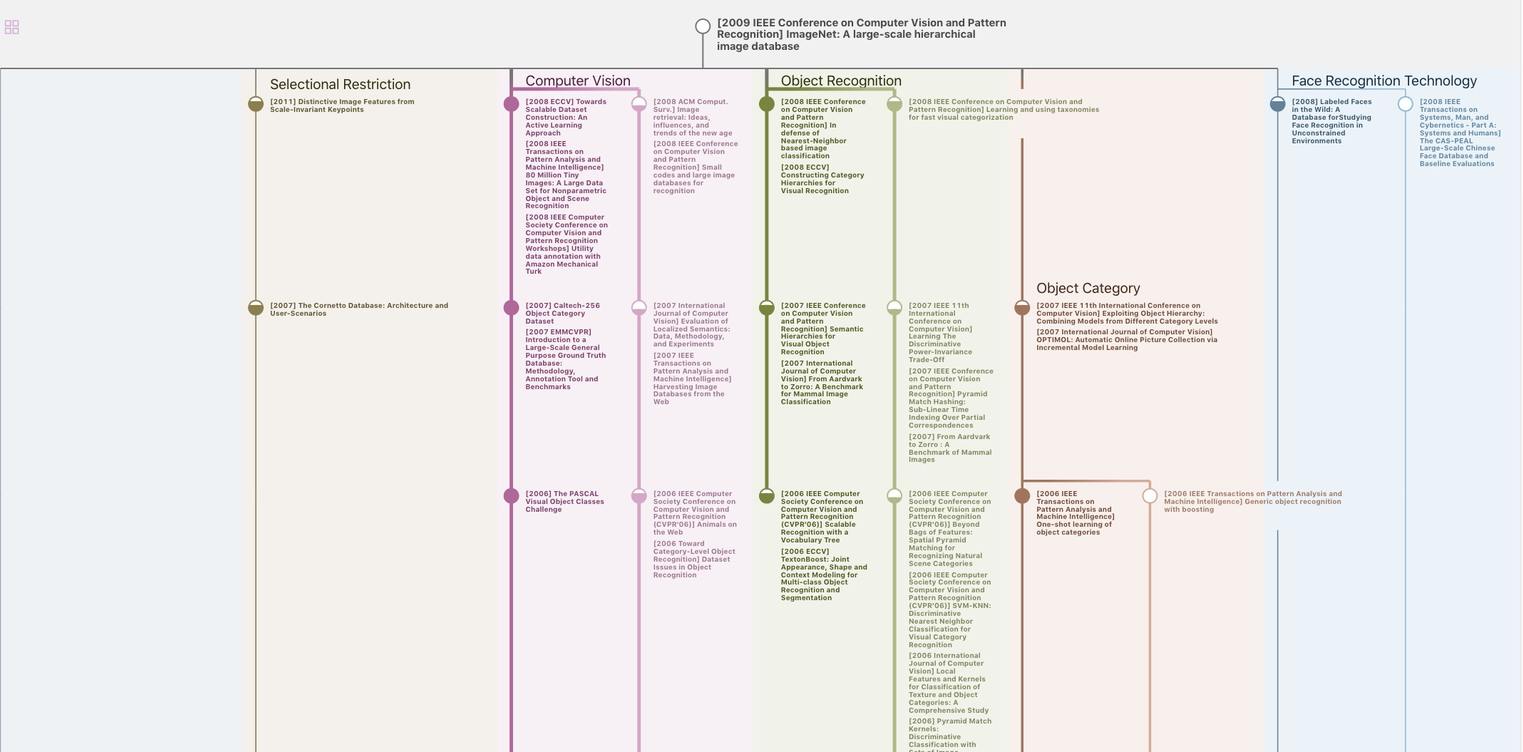
生成溯源树,研究论文发展脉络
Chat Paper
正在生成论文摘要