Cross-modal and Cross-level Attention Interaction Network for Salient Object Detection
IEEE Transactions on Artificial Intelligence(2023)
摘要
Most existing RGB-D salient object detection methods utilize the Convolutional Neural Networks (CNNs) to extract features. However, they fail to extract global information due to the inherent defect of sliding window. On the other hand, with the emergence of depth clues, how to effectively incorporate cross-modal features has become an underlying challenge. In addition, in terms of cross-level feature fusion, most methods do not fully consider the complementarity between different layers and usually adopt simple fusion strategies, thereby leading to the missing of detailed information. To relieve these issues, a Cross-modal and Cross-level Attention Interaction Network (CAINet) is proposed. First, different from most existing methods, we adopt a two-stream Swin Transformers to extract RGB and depth features. Second, a High-level Context Refinement Module (HCRM) is designed to further extract refined features and give accurate guidance in early prediction stage. Third, we design a Cross-modal Interaction Enhancement Module (CIEM) to explore the complementarity of different modalities via co-attention. In terms of fusion for high-level and low-level features in decoding, a Multi-scale Attention Induced Decoder (MAID) is designed to extract and fuse the complementary information at different scales. Finally, the Edge Enhancement Module (EEM) is employed to compensate the dilution of edge. Our proposed CAINet achieves excellent performance compared to other state-of-the-art (SOTA) methods on seven widely used datasets.
更多查看译文
关键词
Salient Object Detection,Swin Transformer,Cross-modal Interaction,Attention Mechanism
AI 理解论文
溯源树
样例
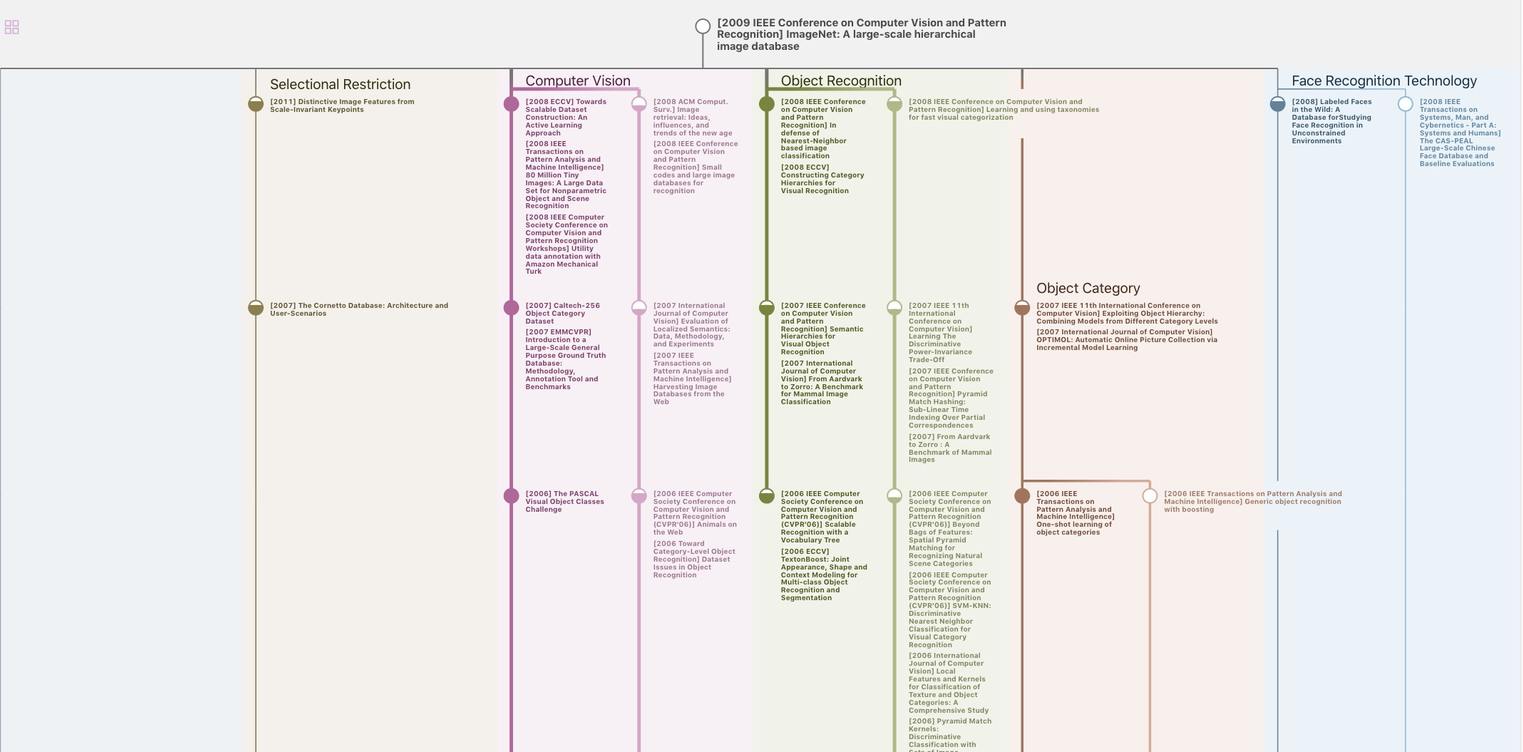
生成溯源树,研究论文发展脉络
Chat Paper
正在生成论文摘要